学习目标:
学习内容:
- 关于PatchGAN的理解
- 卷积层总结
- ReLU激活函数总结
- CycleGAN代码训练过程Trace
学习时间:
学习产出:
马尔科夫判别器PatchGAN
马尔可夫判别器的区别:
- 基于CNN的分类模型有很多种,很多网络都是在最后引入了一个全连接层,然后将判别的结果输出。
- 马尔可夫判别器是由卷积层构成,最后输出一个n*n的矩阵,最后取输出矩阵的均值作为True/False的输出。
- 事实上,输出矩阵中的每个数据代表着原图中一个感受野,对应了原图的一片(patch),这样的GAN称PatchGAN.
感受域计算 每一层感受域的计算公式为: input_size = (output_size-1)*k_stride+k_size 1.感受域:输出节点(输出网络中的每个节点)对输入网络的感受野的范围(可以从输出网络大小反推感受野的大小) 2.input_size:为输出结点感受域的大小 3.k_stride:卷积核的移动步长 4.k_size:输入输出之间卷积核的大小h’j 以CycleGAN代码中的马尔可夫判别器为例: 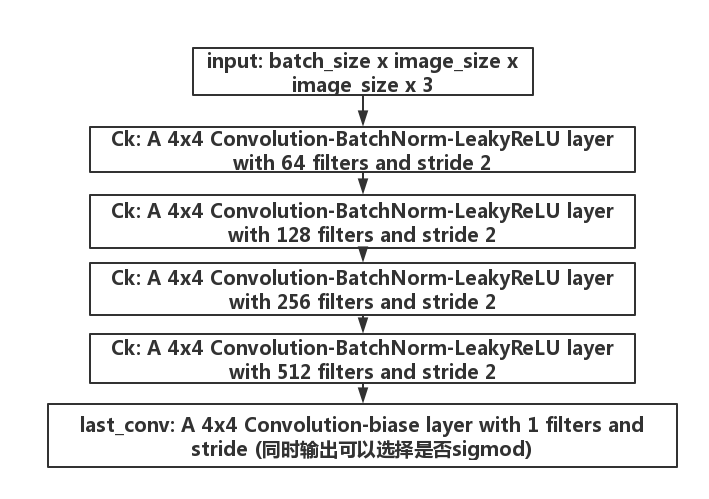
- last_conv输出(k_stride=1),假设其中一个节点,即output_size=1,计算得到CK4的大小(input_size)为4;
- 同理,假设CK4_size = 4,则CK3_size = (4-1)*2+4=10
- 同理,CK2_size = (10-1)*2+4=22;
- 同理,CK1_size = (22-1)*2+4=46;
同理,input的感受域大小为94
前人关于patchgan对作者的提问: 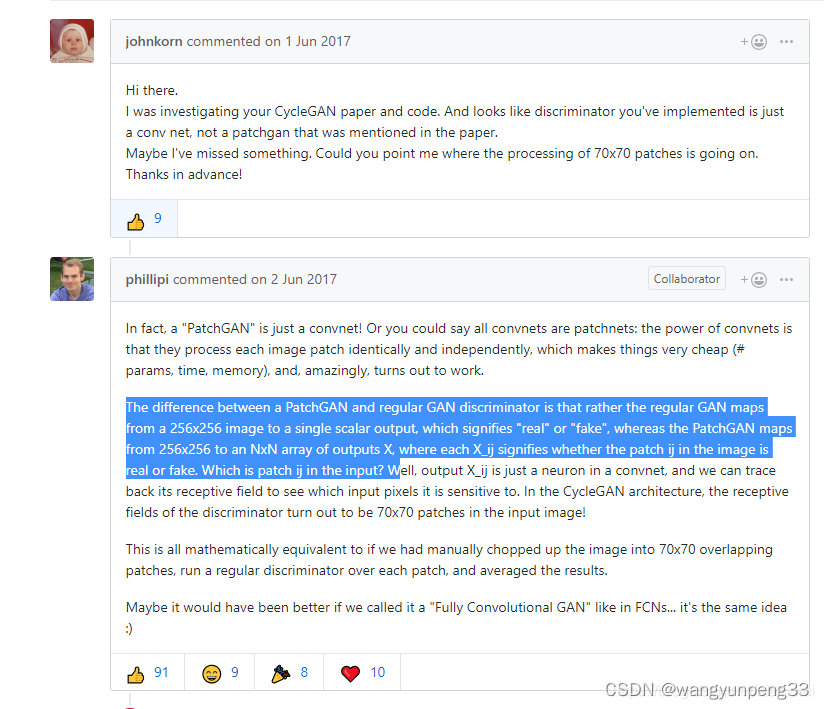
卷积神经网络总结
卷积有一维卷积、二维卷积、三维卷积。一般情况下,卷积核在几个维度上滑动,就是几维卷积。比如我们的cyclegan的网络在图片上的卷积,就是二维卷积。
二维卷积:nn.Conv2d()
nn.Conv2d(self, in_channels, out_channels, kernel_size, stride=1, padding=0, dilation=1, groups=1, bias=True, padding_mode=‘zeros’)
这个函数的功能是对多个二维信号进行二维卷积,主要参数如下:
in_channels:输入通道数 out_channels:输出通道数,等价于卷积核个数 kernel_size:卷积核尺寸 stride:步长 padding:填充宽度,主要是为了调整输出的特征图大小,一般把 padding 设置合适的值后,保持输入和输出的图像尺寸不变。 dilation:空洞卷积大小,默认为 1,这时是标准卷积,常用于图像分割任务中,主要是为了提升感受野 groups:分组卷积设置,主要是为了模型的轻量化,如在 ShuffleNet、MobileNet、SqueezeNet 中用到 bias:偏置
这里不考虑空洞卷积,假设输入图片大小为
I
×
I
I×I
I×I ,卷积核大小为
k
×
k
k×k
k×k ,stride 为
s
s
s ,padding 的像素数为
p
p
p ,图片经过卷积之后的尺寸
O
O
O 如下:
O
=
I
?
k
+
2
?
p
s
+
1
O = \frac{I-k + 2*p}{s} + 1
O=sI?k+2?p?+1
完整版卷积公式: 假设输入图片大小为
I
×
I
I×I
I×I ,卷积核大小为
k
×
k
k×k
k×k ,stride 为
s
s
s ,padding 的像素数为
p
p
p ,空洞卷积dilation 为
d
d
d,图片经过卷积之后的尺寸
O
O
O 如下:
O
=
I
?
d
?
(
k
?
1
)
+
2
?
p
?
1
s
+
1
O = \frac{I-d*(k-1) + 2*p-1}{s} + 1
O=sI?d?(k?1)+2?p?1?+1
转置卷积:nn.ConvTranspose()
转置卷积又称为反卷积 (Deconvolution) 和部分跨越卷积 (Fractionally strided Convolution),用于对图像进行上采样。可以根据卷积核大小和输出的大小,恢复卷积前的图像尺寸,而不是恢复原始值
这里不考虑空洞卷积,假设输入图片大小为
I
×
I
I×I
I×I ,卷积核大小为
k
×
k
k×k
k×k ,stride 为
s
s
s ,padding 的像素数为
p
p
p ,图片经过卷积之后的尺寸
O
O
O 如下,刚好和普通卷积的计算是相反的:
O
=
(
I
?
1
)
×
s
+
k
?
2
p
O = (I - 1) × s + k - 2p
O=(I?1)×s+k?2p
完整版简化版转置卷积尺寸计算
O
=
(
I
?
1
)
×
s
+
d
?
(
k
?
1
)
?
2
p
+
o
u
t
p
a
d
d
i
n
g
+
1
O = (I - 1) × s +d*(k-1) - 2p+outpadding+1
O=(I?1)×s+d?(k?1)?2p+outpadding+1
正常卷积: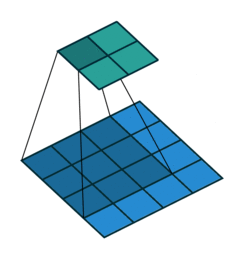 原始输入为
4
×
4
4×4
4×4,卷积核大小
3
×
3
3×3
3×3,
p
a
d
d
i
n
g
=
0
padding=0
padding=0,
s
t
r
i
d
e
=
1
stride=1
stride=1,由于卷积操作可以通过矩阵运算来解决,因此原始图片可以看作
I
16
×
1
I_{16×1}
I16×1?的矩阵 ,卷积核可以看作
K
4
×
16
K_{4×16}
K4×16?的矩阵 ,那么输出是
K
4
×
16
×
I
16
×
1
=
O
4
×
1
K_{4×16}×I_{16×1} = O_{4×1}
K4×16?×I16×1?=O4×1? 的列向量,改写为矩阵为2x2 。
转置卷积: 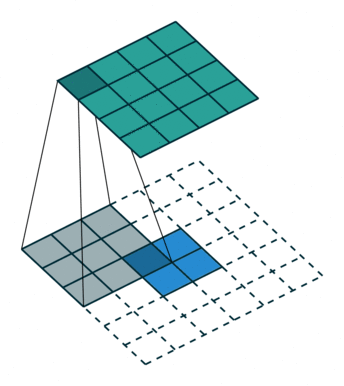 原始的图片尺寸为
2
×
2
2×2
2×2,卷积核大小为
3
×
3
3×3
3×3。由于卷积操作可以通过矩阵运算来解决,因此原始图片可以看作的
I
4
×
1
I_{4×1}
I4×1?矩阵 ,卷积核可以看作
K
16
×
4
K_{16×4}
K16×4?的矩阵 ,那么输出是
K
16
×
4
×
I
4
×
1
=
O
16
×
1
K_{16×4}×I_{4×1} = O_{16×1}
K16×4?×I4×1?=O16×1?的列向量,转化为矩阵
O
4
×
4
O_{4×4}
O4×4?。
更详细的推导参考知乎专栏:转置卷积
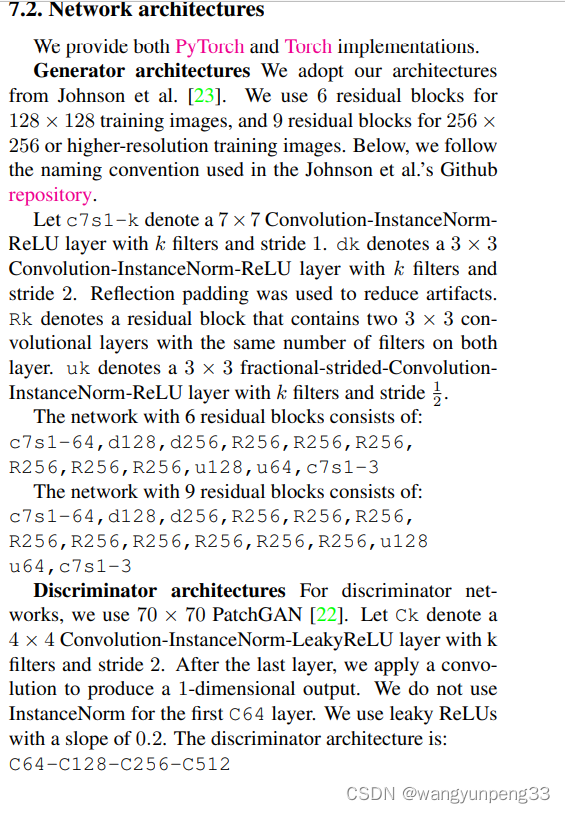
CycleGAN代码训练过程Trace
整体理一下训练过程
train.py
该脚本为训练的起始脚本,在前几行首先实例化TrainOptions(继承了BaseOptions)来接收命令行输入参数
opt = TrainOptions().parse()
然后是数据集的加载部分
dataset = create_dataset(opt)
接着就是加载模型的相关代码
model = create_model(opt)
models/init.py
看一下create_model()
def create_model(opt):
"""Create a model given the option.
This function warps the class CustomDatasetDataLoader.
This is the main interface between this package and 'train.py'/'test.py'
Example:
>>> from models import create_model
>>> model = create_model(opt)
"""
model = find_model_using_name(opt.model)
instance = model(opt)
print("model [%s] was created" % type(instance).__name__)
return instance
使用find_model_using_name来导入对应的包,例如命令行输入参数为cycle_gan则会导入models中的cycle_gan_model.py模块, 如下面代码中print的结果所示:
def find_model_using_name(model_name):
model_filename = "models." + model_name + "_model"
modellib = importlib.import_module(model_filename)
model = None
target_model_name = model_name.replace('_', '') + 'model'
print(modellib.__dict__.items())
for name, cls in modellib.__dict__.items():
if name.lower() == target_model_name.lower() \
and issubclass(cls, BaseModel):
model = cls
if model is None:
print("In %s.py, there should be a subclass of BaseModel with class name that matches %s in lowercase." % (model_filename, target_model_name))
exit(0)
return model
model = cls成功将模型类拿到,最后用return返回,再回到create_model中利用opt实例化模型,实例化会调用所选模型的初始化方法:
cycle_gan_model.py: class CycleGANModel(BaseModel)
cyclegan中的 init() 函数
self.netG_A = networks.define_G(opt.input_nc, opt.output_nc, opt.ngf, opt.netG, opt.norm,
not opt.no_dropout, opt.init_type, opt.init_gain, self.gpu_ids)
self.netG_B = networks.define_G(opt.output_nc, opt.input_nc, opt.ngf, opt.netG, opt.norm,
not opt.no_dropout, opt.init_type, opt.init_gain, self.gpu_ids)
if self.isTrain:
self.netD_A = networks.define_D(opt.output_nc, opt.ndf, opt.netD,
opt.n_layers_D, opt.norm, opt.init_type, opt.init_gain, self.gpu_ids)
self.netD_B = networks.define_D(opt.input_nc, opt.ndf, opt.netD,
opt.n_layers_D, opt.norm, opt.init_type, opt.init_gain, self.gpu_ids)
生成器的网络架构主要通过models/networks里面的define_G函数进行初始化,G_A与G_B构造一样。
判别器的网络架构主要通过define_D函数进行初始化,D_A与D_B也一样。
networks.py: define_G
def define_G(input_nc, output_nc, ngf, netG, norm='batch', use_dropout=False, init_type='normal', init_gain=0.02, gpu_ids=[]):
"""Create a generator
Parameters:
input_nc (int) -- the number of channels in input images
output_nc (int) -- the number of channels in output images
ngf (int) -- the number of filters in the last conv layer
netG (str) -- the architecture's name: resnet_9blocks | resnet_6blocks | unet_256 | unet_128
norm (str) -- the name of normalization layers used in the network: batch | instance | none
use_dropout (bool) -- if use dropout layers.
init_type (str) -- the name of our initialization method.
init_gain (float) -- scaling factor for normal, xavier and orthogonal.
gpu_ids (int list) -- which GPUs the network runs on: e.g., 0,1,2
Returns a generator
Our current implementation provides two types of generators:
U-Net: [unet_128] (for 128x128 input images) and [unet_256] (for 256x256 input images)
The original U-Net paper: https://arxiv.org/abs/1505.04597
Resnet-based generator: [resnet_6blocks] (with 6 Resnet blocks) and [resnet_9blocks] (with 9 Resnet blocks)
Resnet-based generator consists of several Resnet blocks between a few downsampling/upsampling operations.
We adapt Torch code from Justin Johnson's neural style transfer project (https://github.com/jcjohnson/fast-neural-style).
The generator has been initialized by <init_net>. It uses RELU for non-linearity.
"""
net = None
norm_layer = get_norm_layer(norm_type=norm)
if netG == 'resnet_9blocks':
net = ResnetGenerator(input_nc, output_nc, ngf, norm_layer=norm_layer, use_dropout=use_dropout, n_blocks=9)
elif netG == 'resnet_6blocks':
net = ResnetGenerator(input_nc, output_nc, ngf, norm_layer=norm_layer, use_dropout=use_dropout, n_blocks=6)
elif netG == 'unet_128':
net = UnetGenerator(input_nc, output_nc, 7, ngf, norm_layer=norm_layer, use_dropout=use_dropout)
elif netG == 'unet_256':
net = UnetGenerator(input_nc, output_nc, 8, ngf, norm_layer=norm_layer, use_dropout=use_dropout)
else:
raise NotImplementedError('Generator model name [%s] is not recognized' % netG)
return init_net(net, init_type, init_gain, gpu_ids)
ReLU激活函数(Rectify Liner Unit)
通过ReLU实现稀疏后的模型能够更好地挖掘相关特征,拟合训练数据。 引入ReLU的原因:
-
采用sigmoid等函数,算激活函数时(指数运算),计算量大,反向传播求误差梯度时,求导涉及除法,计算量相对大,而采用Relu激活函数,整个过程的计算量节省很多。 -
对于深层网络,sigmoid函数反向传播时,很容易就会出现 梯度消失 的情况(在sigmoid接近饱和区时,变换太缓慢,导数趋于0,这种情况会造成信息丢失),从而无法完成深层网络的训练。 -
ReLu会使一部分神经元的输出为0,这样就造成了 网络的稀疏性,并且减少了参数的相互依存关系,缓解了过拟合问题的发生。
此外,相比于其它激活函数来说,ReLU有以下优势:对于线性函数而言,ReLU的表达能力更强,尤其体现在深度网络中;而对于非线性函数而言,ReLU由于非负区间的梯度为常数,因此不存在梯度消失问题(Vanishing Gradient Problem),使得模型的收敛速度维持在一个稳定状态
参考:ReLU知乎专栏
networks.py: class ResnetGenerator(nn.Module)
因为存在梯度消失和梯度爆炸问题,深层神经网络往往难以训练。跳跃连接(Skip connection)可以从某一层网络层获取激活,然后迅速反馈给另外一层,甚至是神经网络的更深层。利用跳跃连接构建能够训练深度网络的ResNets。
以残差生成器为例,单个残差块的构造如下:残差块是带skip connection的卷积块。残差结构最大的优点:有效地缓解梯度消失,梯度爆炸的问题 一个残差块的构造: 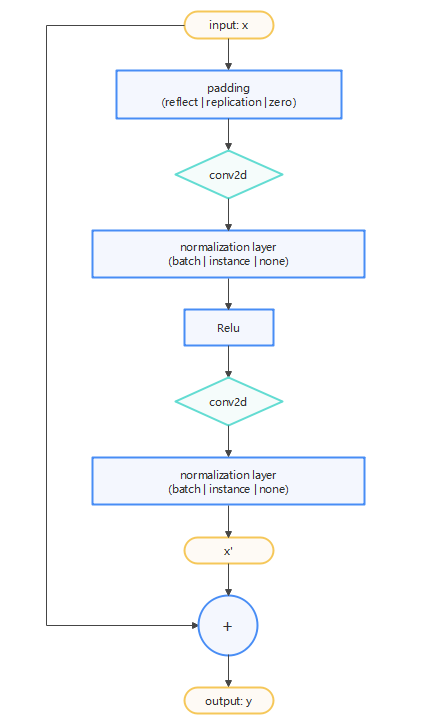
class ResnetBlock(nn.Module):
"""Define a Resnet block"""
def __init__(self, dim, padding_type, norm_layer, use_dropout, use_bias):
"""Initialize the Resnet block
A resnet block is a conv block with skip connections
We construct a conv block with build_conv_block function,
and implement skip connections in <forward> function.
Original Resnet paper: https://arxiv.org/pdf/1512.03385.pdf
"""
super(ResnetBlock, self).__init__()
self.conv_block = self.build_conv_block(dim, padding_type, norm_layer, use_dropout, use_bias)
def build_conv_block(self, dim, padding_type, norm_layer, use_dropout, use_bias):
"""Construct a convolutional block.
Parameters:
dim (int) -- the number of channels in the conv layer.
padding_type (str) -- the name of padding layer: reflect | replicate | zero
norm_layer -- normalization layer
use_dropout (bool) -- if use dropout layers.
use_bias (bool) -- if the conv layer uses bias or not
Returns a conv block (with a conv layer, a normalization layer, and a non-linearity layer (ReLU))
"""
conv_block = []
p = 0
if padding_type == 'reflect':
conv_block += [nn.ReflectionPad2d(1)]
elif padding_type == 'replicate':
conv_block += [nn.ReplicationPad2d(1)]
elif padding_type == 'zero':
p = 1
else:
raise NotImplementedError('padding [%s] is not implemented' % padding_type)
conv_block += [nn.Conv2d(dim, dim, kernel_size=3, padding=p, bias=use_bias), norm_layer(dim), nn.ReLU(True)]
if use_dropout:
conv_block += [nn.Dropout(0.5)]
p = 0
if padding_type == 'reflect':
conv_block += [nn.ReflectionPad2d(1)]
elif padding_type == 'replicate':
conv_block += [nn.ReplicationPad2d(1)]
elif padding_type == 'zero':
p = 1
else:
raise NotImplementedError('padding [%s] is not implemented' % padding_type)
conv_block += [nn.Conv2d(dim, dim, kernel_size=3, padding=p, bias=use_bias), norm_layer(dim)]
return nn.Sequential(*conv_block)
def forward(self, x):
"""Forward function (with skip connections)"""
out = x + self.conv_block(x)
return out
skip connections: forward 前向传播返回
x
+
x
′
x + x_{'}
x+x′?
生成器构造
class ResnetGenerator(nn.Module):
"""Resnet-based generator that consists of Resnet blocks between a few downsampling/upsampling operations.
We adapt Torch code and idea from Justin Johnson's neural style transfer project(https://github.com/jcjohnson/fast-neural-style)
"""
def __init__(self, input_nc, output_nc, ngf=64, norm_layer=nn.BatchNorm2d, use_dropout=False, n_blocks=6, padding_type='reflect'):
"""Construct a Resnet-based generator
Parameters:
input_nc (int) -- the number of channels in input images
output_nc (int) -- the number of channels in output images
ngf (int) -- the number of filters in the last conv layer
norm_layer -- normalization layer
use_dropout (bool) -- if use dropout layers
n_blocks (int) -- the number of ResNet blocks
padding_type (str) -- the name of padding layer in conv layers: reflect | replicate | zero
"""
assert(n_blocks >= 0)
super(ResnetGenerator, self).__init__()
if type(norm_layer) == functools.partial:
use_bias = norm_layer.func == nn.InstanceNorm2d
else:
use_bias = norm_layer == nn.InstanceNorm2d
model = [nn.ReflectionPad2d(3),
nn.Conv2d(input_nc, ngf, kernel_size=7, padding=0, bias=use_bias),
norm_layer(ngf),
nn.ReLU(True)]
n_downsampling = 2
for i in range(n_downsampling):
mult = 2 ** i
model += [nn.Conv2d(ngf * mult, ngf * mult * 2, kernel_size=3, stride=2, padding=1, bias=use_bias),
norm_layer(ngf * mult * 2),
nn.ReLU(True)]
mult = 2 ** n_downsampling
for i in range(n_blocks):
model += [ResnetBlock(ngf * mult, padding_type=padding_type, norm_layer=norm_layer, use_dropout=use_dropout, use_bias=use_bias)]
for i in range(n_downsampling):
mult = 2 ** (n_downsampling - i)
model += [nn.ConvTranspose2d(ngf * mult, int(ngf * mult / 2),
kernel_size=3, stride=2,
padding=1, output_padding=1,
bias=use_bias),
norm_layer(int(ngf * mult / 2)),
nn.ReLU(True)]
model += [nn.ReflectionPad2d(3)]
model += [nn.Conv2d(ngf, output_nc, kernel_size=7, padding=0)]
model += [nn.Tanh()]
self.model = nn.Sequential(*model)
def forward(self, input):
"""Standard forward"""
return self.model(input)
输入首先经过reflection padding,再经过一个conv2d, norm layer 和 Rule 的组合,然后进入下采样阶段——先后经过两个下采样模块(conv2d → norm layer → Relu),然后经过若干个残差块(6 || 9),随后进入上采样阶段——先后经过两个上采样模块,只不过将下采样模块中的conv2d换成反卷积操作convtranspose2d,最后在经过一次reflection padding,conv2d, 然后经过tanh得到最后输出。
整个架构为:
x → reflection padding → conv2d → Relu → down sampling → down sampling → resblock * n → up sampling → up sampling → reflection padding → conv2d → tanh → y
判别器构造
class NLayerDiscriminator(nn.Module):
"""Defines a PatchGAN discriminator"""
def __init__(self, input_nc, ndf=64, n_layers=3, norm_layer=nn.BatchNorm2d):
"""Construct a PatchGAN discriminator
Parameters:
input_nc (int) -- the number of channels in input images
ndf (int) -- the number of filters in the last conv layer
n_layers (int) -- the number of conv layers in the discriminator
norm_layer -- normalization layer
"""
super(NLayerDiscriminator, self).__init__()
if type(norm_layer) == functools.partial:
use_bias = norm_layer.func == nn.InstanceNorm2d
else:
use_bias = norm_layer == nn.InstanceNorm2d
kw = 4
padw = 1
sequence = [nn.Conv2d(input_nc, ndf, kernel_size=kw, stride=2, padding=padw), nn.LeakyReLU(0.2, True)]
nf_mult = 1
nf_mult_prev = 1
for n in range(1, n_layers):
nf_mult_prev = nf_mult
nf_mult = min(2 ** n, 8)
sequence += [
nn.Conv2d(ndf * nf_mult_prev, ndf * nf_mult, kernel_size=kw, stride=2, padding=padw, bias=use_bias),
norm_layer(ndf * nf_mult),
nn.LeakyReLU(0.2, True)
]
nf_mult_prev = nf_mult
nf_mult = min(2 ** n_layers, 8)
sequence += [
nn.Conv2d(ndf * nf_mult_prev, ndf * nf_mult, kernel_size=kw, stride=1, padding=padw, bias=use_bias),
norm_layer(ndf * nf_mult),
nn.LeakyReLU(0.2, True)
]
sequence += [nn.Conv2d(ndf * nf_mult, 1, kernel_size=kw, stride=1, padding=padw)]
self.model = nn.Sequential(*sequence)
def forward(self, input):
"""Standard forward."""
return self.model(input)
整个架构如下:
*x → n (conv2d → norm_layer → LeakyRelu) → conv2d → norm_layer → LeakyRelu → conv2d → y
整个网络的前向传播过程:
def forward(self):
"""Run forward pass; called by both functions <optimize_parameters> and <test>."""
self.fake_B = self.netG_A(self.real_A)
self.rec_A = self.netG_B(self.fake_B)
self.fake_A = self.netG_B(self.real_B)
self.rec_B = self.netG_A(self.fake_A)
train.py 计算损失
for epoch in range(opt.epoch_count, opt.n_epochs + opt.n_epochs_decay + 1):
epoch_start_time = time.time()
iter_data_time = time.time()
epoch_iter = 0
visualizer.reset()
model.update_learning_rate()
for i, data in enumerate(dataset):
iter_start_time = time.time()
if total_iters % opt.print_freq == 0:
t_data = iter_start_time - iter_data_time
total_iters += opt.batch_size
epoch_iter += opt.batch_size
model.set_input(data)
model.optimize_parameters()
通过 optimize_parameters() 计算损失,继承自class BaseModel,看cycle_gan如何复写基类中的这个抽象函数。
cycle_gan_model.py: class CycleGANModel(BaseModel)
def optimize_parameters(self):
"""Calculate losses, gradients, and update network weights; called in every training iteration"""
self.forward()
self.set_requires_grad([self.netD_A, self.netD_B], False)
self.optimizer_G.zero_grad()
self.backward_G()
self.optimizer_G.step()
self.set_requires_grad([self.netD_A, self.netD_B], True)
self.optimizer_D.zero_grad()
self.backward_D_A()
self.backward_D_B()
self.optimizer_D.step()
其中 set_requires_grad() 方法负责设置判别器和生成器中的参数是否需要记录梯度,这里生成器不需要记录的原因是,生成器的进化只依赖于判别器给生成器的反馈与判别器的参数无关。
def set_requires_grad(self, nets, requires_grad=False):
"""Set requies_grad=Fasle for all the networks to avoid unnecessary computations
Parameters:
nets (network list) -- a list of networks
requires_grad (bool) -- whether the networks require gradients or not
"""
if not isinstance(nets, list):
nets = [nets]
for net in nets:
if net is not None:
for param in net.parameters():
param.requires_grad = requires_grad
backward_G()
lambda_A:weight for cycle loss (A -> B -> A) A方向重建损失权重
lambda_B:weight for cycle loss (B -> A -> B) B方向重建损失权重
lambda_identity:identity loss相对于cycle loss的比例
通过判断lambda_idt是否大于0,来判断当前实验是否计算Identity Loss
def backward_G(self):
"""Calculate the loss for generators G_A and G_B"""
lambda_idt = self.opt.lambda_identity
lambda_A = self.opt.lambda_A
lambda_B = self.opt.lambda_B
if lambda_idt > 0:
self.idt_A = self.netG_A(self.real_B)
self.loss_idt_A = self.criterionIdt(self.idt_A, self.real_B) * lambda_B * lambda_idt
self.idt_B = self.netG_B(self.real_A)
self.loss_idt_B = self.criterionIdt(self.idt_B, self.real_A) * lambda_A * lambda_idt
else:
self.loss_idt_A = 0
self.loss_idt_B = 0
self.loss_G_A = self.criterionGAN(self.netD_A(self.fake_B), True)
self.loss_G_B = self.criterionGAN(self.netD_B(self.fake_A), True)
self.loss_cycle_A = self.criterionCycle(self.rec_A, self.real_A) * lambda_A
self.loss_cycle_B = self.criterionCycle(self.rec_B, self.real_B) * lambda_B
self.loss_G = self.loss_G_A + self.loss_G_B + self.loss_cycle_A + self.loss_cycle_B + self.loss_idt_A + self.loss_idt_B
self.loss_G.backward()
GAN loss调用network.py中的GANLoss Cycle loss, Identity loss均为L1损失函数。
self.criterionGAN = networks.GANLoss(opt.gan_mode).to(self.device)
self.criterionCycle = torch.nn.L1Loss()
self.criterionIdt = torch.nn.L1Loss()
networks.py: class GANLoss(nn.Module)
在 init() 方法中有三种loss可以选择:lsgan,vanilla,wgangp。然后用register_buffer(无需在反向传播中更新,因此用buffer创建,state_dict也会记录用buffer创建的参数)创建real label,fake label对应的tensor。随后在get_target_tensor()方法中将label tensor用expend_as扩展为和prediction一样shape的tensor,方便后续loss的计算。
class GANLoss(nn.Module):
"""Define different GAN objectives.
The GANLoss class abstracts away the need to create the target label tensor
that has the same size as the input.
"""
def __init__(self, gan_mode, target_real_label=1.0, target_fake_label=0.0):
""" Initialize the GANLoss class.
Parameters:
gan_mode (str) - - the type of GAN objective. It currently supports vanilla, lsgan, and wgangp.
target_real_label (bool) - - label for a real image
target_fake_label (bool) - - label of a fake image
Note: Do not use sigmoid as the last layer of Discriminator.
LSGAN needs no sigmoid. vanilla GANs will handle it with BCEWithLogitsLoss.
"""
super(GANLoss, self).__init__()
self.register_buffer('real_label', torch.tensor(target_real_label))
self.register_buffer('fake_label', torch.tensor(target_fake_label))
self.gan_mode = gan_mode
if gan_mode == 'lsgan':
self.loss = nn.MSELoss()
elif gan_mode == 'vanilla':
self.loss = nn.BCEWithLogitsLoss()
elif gan_mode in ['wgangp']:
self.loss = None
else:
raise NotImplementedError('gan mode %s not implemented' % gan_mode)
def get_target_tensor(self, prediction, target_is_real):
"""Create label tensors with the same size as the input.
Parameters:
prediction (tensor) - - tpyically the prediction from a discriminator
target_is_real (bool) - - if the ground truth label is for real images or fake images
Returns:
A label tensor filled with ground truth label, and with the size of the input
"""
if target_is_real:
target_tensor = self.real_label
else:
target_tensor = self.fake_label
return target_tensor.expand_as(prediction)
def __call__(self, prediction, target_is_real):
"""Calculate loss given Discriminator's output and grount truth labels.
Parameters:
prediction (tensor) - - tpyically the prediction output from a discriminator
target_is_real (bool) - - if the ground truth label is for real images or fake images
Returns:
the calculated loss.
"""
if self.gan_mode in ['lsgan', 'vanilla']:
target_tensor = self.get_target_tensor(prediction, target_is_real)
loss = self.loss(prediction, target_tensor)
elif self.gan_mode == 'wgangp':
if target_is_real:
loss = -prediction.mean()
else:
loss = prediction.mean()
return loss
horse2zebra数据集训练过程可视化
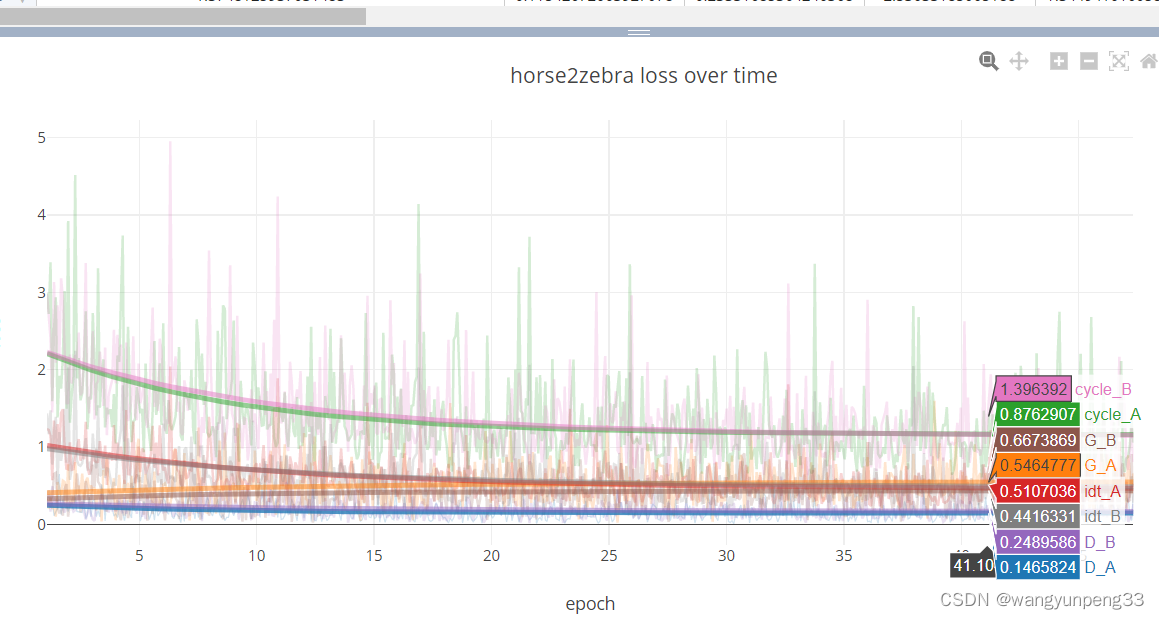
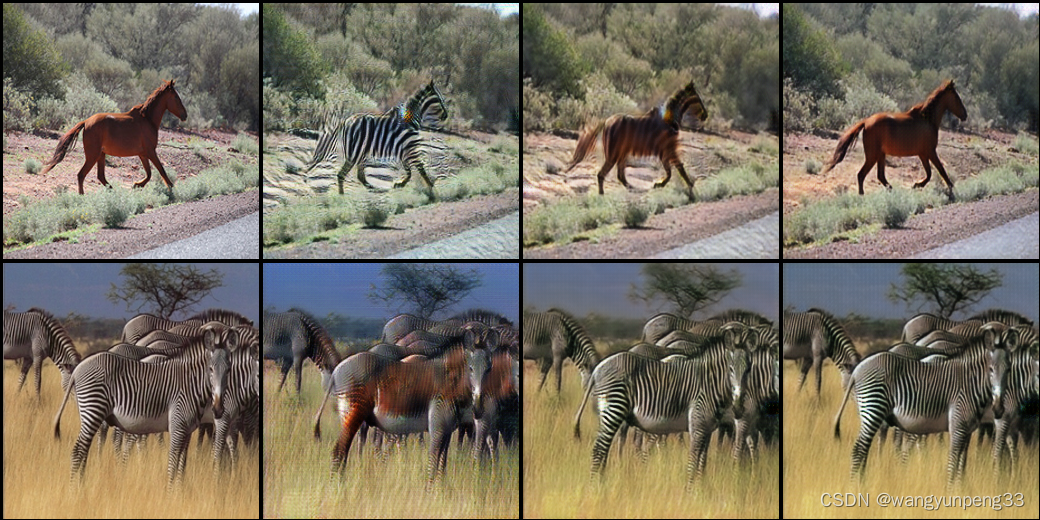
Screen使用
我们常需要SSH 或者telent 远程登录到Linux 服务器,经常运行一些需要很长时间才能完成的任务,最常用的就是我们训练一个模型,时间很长。在此期间不能关掉窗口或者断开连接,否则这个任务就会被杀掉,一切半途而废了。这时,我们可以用screen命令解决这个问题。 screen命令用法:
创建新的screen会话 screen -S [SCREEN]
加入screen会话
screen -x [SCREEN]
退出并关闭screen会话
exit
离开当前screen会话(不退出)
Ctrl+a+d
显示所有已经打开的screen会话
screen -ls
恢复某screen会话(只有当此会话没人连接时,用-r才可以恢复连接,)
screen -r [SCREEN]
|