Introduction
- 大部分 VLM (Visual-Language Model) 依赖于目标检测模型抽取视觉特征,因此会难以学得多个物体间的关系。为了解决上述问题,作者提出了端到端的多模态模型 X-VLM 来进行 “multi-grained vision language pre-training” (align the text with fine-grained (object-centric) features + learn alignments between the texts and coarse-grained (overall) features of the image). 例如在下图中,数据集包含 image caption、region annotations such as “man wearing backpack”、object labels such as “backpack”
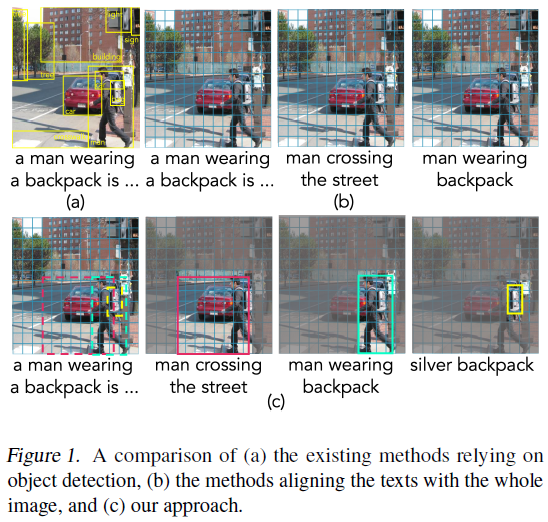
Method
- Overview: X-VLM 由 image encoder
I
t
r
a
n
s
I_{trans}
Itrans? (swin transformer), text encoder
T
t
r
a
n
s
T_{trans}
Ttrans? (6-layer transformer; 由 BERT base 的前 6 层初始化) 和 cross-modal encoder
X
t
r
a
n
s
X_{trans}
Xtrans? (6-layer transformer; 由 BERT base 的后 6 层初始化) 组成,所有 encoders 均为 Transformer. 同时,X-VLM 更充分地利用了预训练数据集中的物体检测框信息,每个样本可以表示为
(
I
,
T
,
{
(
V
j
,
T
j
)
}
N
)
(I,T,\{(V^j,T^j)\}^N)
(I,T,{(Vj,Tj)}N),其中
I
I
I 为 image,
T
T
T 为 text,
{
(
V
j
,
T
j
)
}
N
\{(V^j,T^j)\}^N
{(Vj,Tj)}N 为图片中
N
N
N 个 bbox 的图像和文本信息 (对于某些样本而言,可能有
T
=
NaN
T=\text{NaN}
T=NaN,即图像没有描述文本,或
N
=
0
N=0
N=0,即没有物体检测框信息)
- Vision Encoding:
I
t
r
a
n
s
I_{trans}
Itrans? 为 Swin transformer. 对于
224
×
224
224\times224
224×224 的输入图像 (预训练时图像尺寸为
224
×
224
224\times224
224×224,微调时图像尺寸为
384
×
384
384\times384
384×384),patch 大小为
32
×
32
32\times32
32×32 时,
I
t
r
a
n
s
I_{trans}
Itrans? 输出为
{
v
1
,
.
.
.
,
v
N
I
}
\{v_1,...,v_{N^I}\}
{v1?,...,vNI?} (
N
I
=
49
N^I=49
NI=49),其中
v
p
i
v_{p_i}
vpi?? 编码了 patch
p
i
p_i
pi? 的视觉信息。因此对于视觉对象
V
j
V^j
Vj (object, region, or the image) 而言,它的视觉编码为
I
t
r
a
n
s
(
V
j
)
=
{
v
c
l
s
j
,
v
p
1
j
,
.
.
.
,
v
p
M
j
}
I_{trans}(V^j)=\{v_{cls}^j,v_{p_1^j},...,v_{p_M^j}\}
Itrans?(Vj)={vclsj?,vp1j??,...,vpMj??} (
j
∈
[
0
,
N
]
j\in[0,N]
j∈[0,N]),其中
p
1
j
,
.
.
.
,
p
M
j
p_1^j,...,p_M^j
p1j?,...,pMj? 为
V
j
V^j
Vj 包含的
M
M
M 个 patch,
v
c
l
s
j
v_{cls}^j
vclsj? 为
v
p
1
j
,
.
.
.
,
v
p
M
j
v_{p_1^j},...,v_{p_M^j}
vp1j??,...,vpMj?? 的均值,代表
V
j
V^j
Vj 整体的视觉特征。对于整张图像而言,
I
t
r
a
n
s
I_{trans}
Itrans? 可以编码出
N
+
1
N+1
N+1 个视觉对象,包括
N
N
N 个 bbox
V
1
,
.
.
.
,
V
N
V^1,...,V^N
V1,...,VN 和整张图像
I
=
V
0
I=V^0
I=V0
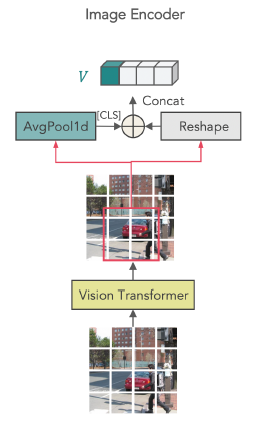 - Cross-Modal Modeling:
- Bounding Box Prediction: 作者让模型根据图像
I
I
I 和
V
j
V^j
Vj 对应的文本描述
T
j
T^j
Tj 去预测
V
j
V^j
Vj 对应 bbox
b
j
b^j
bj 的位置,这可以帮助模型更好地进行跨模态细粒度语义对齐
其中,Sigmoid 是用于输出归一化的 bbox 坐标,
x
c
l
s
j
x_{cls}^j
xclsj? 为 cross-modal encoder 输入
I
,
T
j
I,T^j
I,Tj 后输出的 [CLS] 对应的特征向量 损失函数采用 L1 损失 (scale-variant) 和 generalized IoU loss (scale-invariant) 之和  - Contrastive Learning: 和 CLIP 类似,X-VLM 也使用了对比损失。随机采样
N
N
N 个图像文本对 (1 个 batch),可以计算如下的 in-batch vision-to-text similarity:
其中,
(
V
,
T
)
(V,T)
(V,T) 为正样本,
V
V
V 和同一 batch 内的其他文本
T
i
T^i
Ti 组成
N
?
1
N-1
N?1 个负样本。
s
(
V
,
T
)
=
g
v
(
v
c
l
s
)
T
g
w
(
w
c
l
s
)
s(V,T)=g_v(v_{cls})^Tg_w(w_{cls})
s(V,T)=gv?(vcls?)Tgw?(wcls?) 为余弦相似度,
v
c
l
s
,
w
c
l
s
v_{cls},w_{cls}
vcls?,wcls? 分别为
V
V
V 和
T
T
T 的 [CLS] 对应的输出,
g
v
,
g
w
g_v,g_w
gv?,gw? 分别将
v
c
l
s
,
w
c
l
s
v_{cls},w_{cls}
vcls?,wcls? 变换为低维的归一化特征表示。类似地,text-to-vision similarity 为 损失函数为 其中,
y
v
2
t
(
V
)
,
y
t
2
v
(
T
)
y^{v2t}(V),y^{t2v}(T)
yv2t(V),yt2v(T) 为 ground-truth one-hot similarity (only the positive pair has the probability of one),
H
H
H 为交叉熵损失 - Matching Prediction:对于每个
V
V
V,根据 in-batch vision-to-text similarity
p
v
2
t
(
V
)
p^{v2t}(V)
pv2t(V) 从 batch 内采样出一个 in-batch hard negative text,与
V
V
V 更相近的 text 被采样的几率更大;同理,对于每个
T
T
T,也采样出一个 in-batch hard negative visual concept,由此得到
N
N
N 个正样本和
2
N
2N
2N 个负样本。损失函数为交叉熵损失
其中,
p
m
a
t
c
h
p^{match}
pmatch 为模型预测的匹配概率 - Masked Language Modeling:随机 mask 25% 的文本,其中 10% 替换为随机 token,10% 不变,80% 替换为 [MASK],然后使用 cross-modal encoder 的输出加上线性层和 Softmax 重构 mask 掉的 token. 损失函数为
其中,
T
^
\hat T
T^ 为 masked text,
p
j
(
V
,
T
^
)
p^j(V,\hat T)
pj(V,T^) 为 masked token
t
j
t_j
tj? 的预测概率 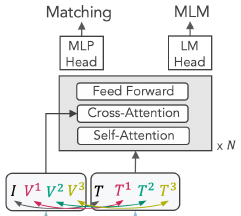
Experiment
Pre-training Datasets
- 为了便于比较,X-VLM 准备了 2 种预训练数据集设置 (4M / 16M),其中 Ann(otations) 为图片的 object annotations 和 region annotations
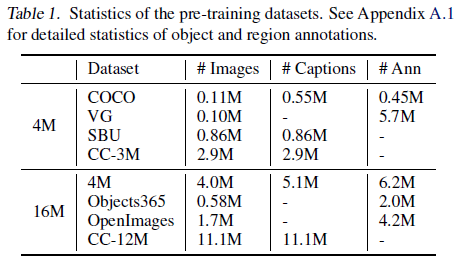
Image-Text Retrieval
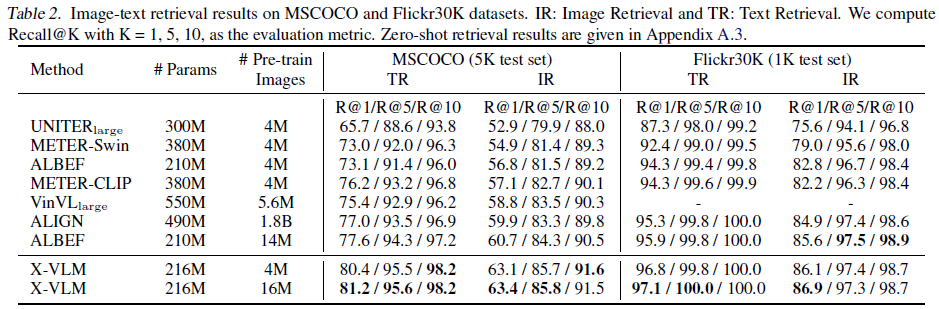
Visual Question Answering, Natural Language for Visual Reasoning (NLVR2), Visual Grounding, Image Captioning
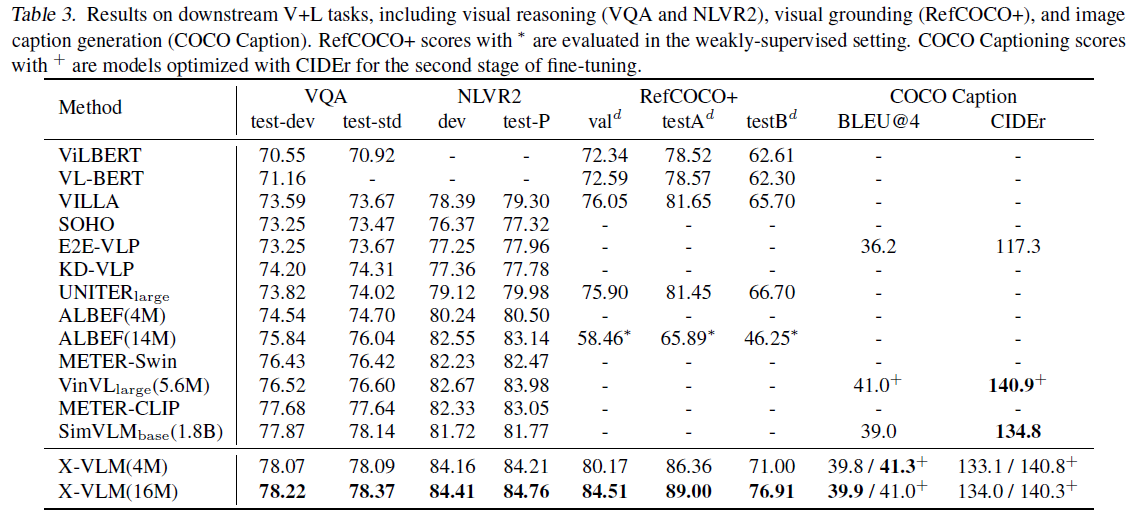
References
|