用1层隐藏层的神经网络分类二维数据
欢迎来到第3周的编程作业。 现在是时候建立你的第一个神经网络了,它将具有一层隐藏层。 你将看到此模型与你使用逻辑回归实现的模型之间的巨大差异。
你将学到如何:
- 实现具有单个隐藏层的2分类神经网络
- 使用具有非线性激活函数的神经元,例如tanh
- 计算交叉熵损失
- 实现前向和后向传播
1- 安装包
让我们首先导入在作业过程中需要的所有软件包。
- numpy是Python科学计算的基本包。
- sklearn提供了用于数据挖掘和分析的简单有效的工具。
- matplotlib 是在Python中常用的绘制图形的库。
- testCases提供了一些测试示例用以评估函数的正确性
- planar_utils提供了此作业中使用的各种函数
In [2]:
cd ../input/deeplearning24054
/home/kesci/input/deeplearning24054
In [3]:
import numpy as np
import matplotlib.pyplot as plt
from testCases import *
import sklearn
import sklearn.datasets
import sklearn.linear_model
from planar_utils import plot_decision_boundary, sigmoid, load_planar_dataset, load_extra_datasets
%matplotlib inline
np.random.seed(1)
2- 数据集
首先,让我们获取处理的数据集。 以下代码会将“flower” 2分类数据集加载到变量 X 和 Y 中。
In [4]:
X, Y = load_planar_dataset()
使用matplotlib可视化数据集。 数据看起来像是带有一些红色(标签y = 0)和一些蓝色(y = 1)点的“花”。 我们的目标是建立一个适合该数据的分类模型。
In [5]:
plt.scatter(X[0, :], X[1, :], c=Y.reshape(X[0,:].shape), s=40, cmap=plt.cm.Spectral)
Out[5]:
<matplotlib.collections.PathCollection at 0x7f90e3ed0668>
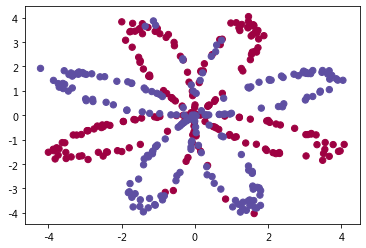
现在你有: - 包含特征(x1,x2)的numpy数组(矩阵)X - 包含标签(红色:0,蓝色:1)的numpy数组(向量)Y。
首先让我们深入地了解一下我们的数据。
练习:数据集中有多少个训练示例? 另外,变量“ X”和“ Y”的“shape”是什么?
提示:如何获得numpy数组的shape维度? (help)
In [4]:
shape_X = X.shape
shape_Y = Y.shape
m = shape_X[1]
print ('The shape of X is: ' + str(shape_X))
print ('The shape of Y is: ' + str(shape_Y))
print ('I have m = %d training examples!' % (m))
The shape of X is: (2, 400)
The shape of Y is: (1, 400)
I have m = 400 training examples!
预期输出: The shape of X is: (2, 400) The shape of Y is: (1, 400) I have m = 400 training examples!
3- 简单Logistic回归
在构建完整的神经网络之前,首先让我们看看逻辑回归在此问题上的表现。 你可以使用sklearn的内置函数来执行此操作。 运行以下代码以在数据集上训练逻辑回归分类器。
In [5]:
clf = sklearn.linear_model.LogisticRegressionCV();
clf.fit(X.T, Y.T);
/opt/conda/lib/python3.6/site-packages/sklearn/utils/validation.py:724: DataConversionWarning: A column-vector y was passed when a 1d array was expected. Please change the shape of y to (n_samples, ), for example using ravel().
y = column_or_1d(y, warn=True)
/opt/conda/lib/python3.6/site-packages/sklearn/model_selection/_split.py:1978: FutureWarning: The default value of cv will change from 3 to 5 in version 0.22. Specify it explicitly to silence this warning.
warnings.warn(CV_WARNING, FutureWarning)
现在,你可以运行下面的代码以绘制此模型的决策边界:
In [6]:
plot_decision_boundary(lambda x: clf.predict(x), X, Y)
plt.title("Logistic Regression")
LR_predictions = clf.predict(X.T)
print ('Accuracy of logistic regression: %d ' % float((np.dot(Y,LR_predictions) + np.dot(1-Y,1-LR_predictions))/float(Y.size)*100) +
'% ' + "(percentage of correctly labelled datapoints)")
Accuracy of logistic regression: 47 % (percentage of correctly labelled datapoints)
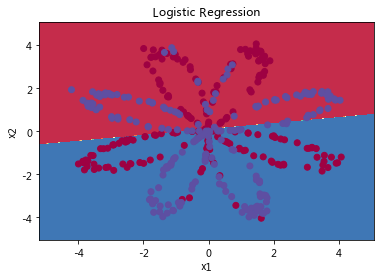
预期输出: Accuracy of logistic regression: 47 % (percentage of correctly labelled datapoints)
Interpretation: The dataset is not linearly separable, so logistic regression doesn’t perform well. Hopefully a neural network will do better. Let’s try this now!
说明:由于数据集不是线性可分类的,因此逻辑回归效果不佳。 让我们试试是否神经网络会做得更好吧!
4- 神经网络模型
从上面我们可以得知Logistic回归不适用于“flower数据集”。现在你将训练带有单个隐藏层的神经网络。
这是我们的模型:
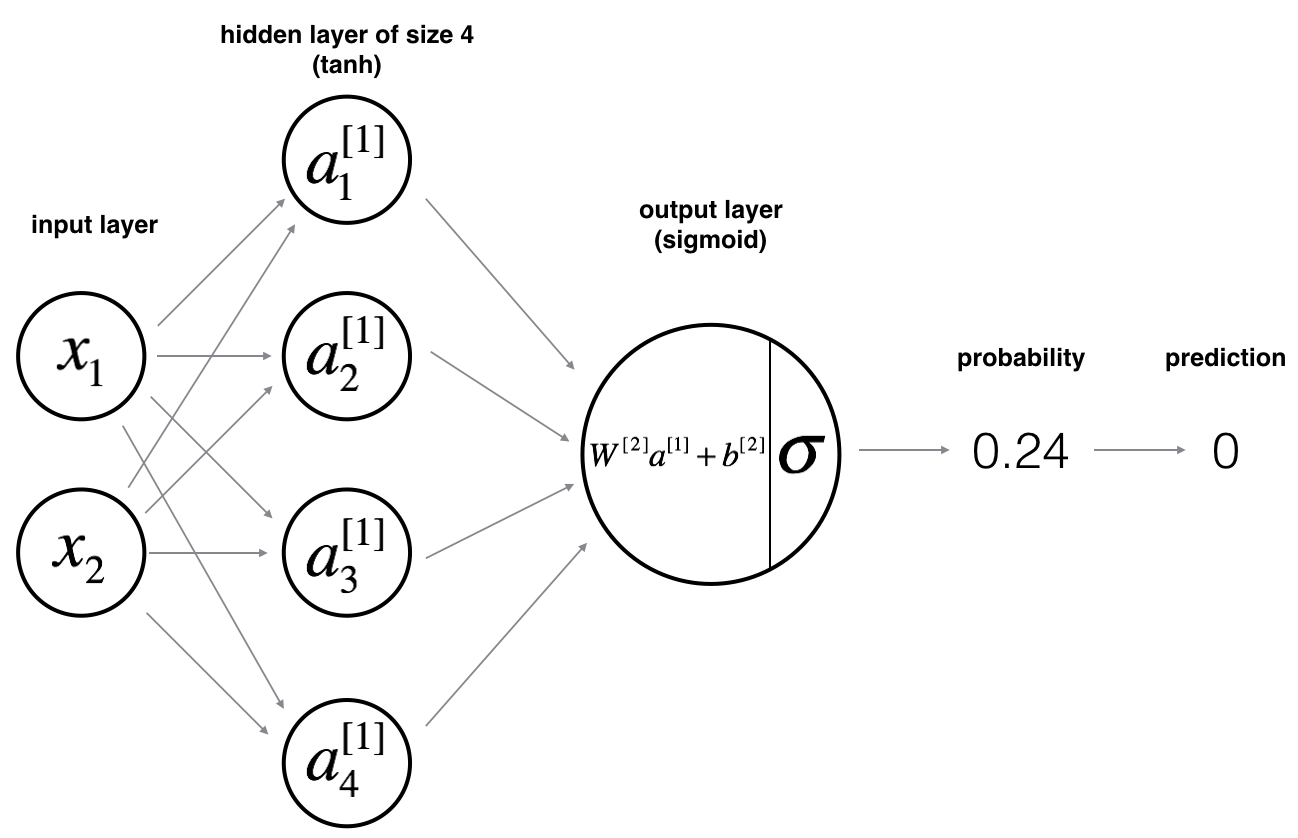
数学原理:
例如
x
(
i
)
x^{(i)}
x(i):
z
[
1
]
(
i
)
=
W
[
1
]
x
(
i
)
+
b
[
1
]
(
i
)
(1)
z^{[1] (i)} = W^{[1]} x^{(i)} + b^{[1] (i)}\tag{1}
z[1](i)=W[1]x(i)+b[1](i)(1)
a
[
1
]
(
i
)
=
tanh
?
(
z
[
1
]
(
i
)
)
(2)
a^{[1] (i)} = \tanh(z^{[1] (i)})\tag{2}
a[1](i)=tanh(z[1](i))(2)
z
[
2
]
(
i
)
=
W
[
2
]
a
[
1
]
(
i
)
+
b
[
2
]
(
i
)
(3)
z^{[2] (i)} = W^{[2]} a^{[1] (i)} + b^{[2] (i)}\tag{3}
z[2](i)=W[2]a[1](i)+b[2](i)(3)
y
^
(
i
)
=
a
[
2
]
(
i
)
=
σ
(
z
[
2
]
(
i
)
)
(4)
\hat{y}^{(i)} = a^{[2] (i)} = \sigma(z^{ [2] (i)})\tag{4}
y^?(i)=a[2](i)=σ(z[2](i))(4)
KaTeX parse error: Undefined control sequence: \mbox at position 43: …gin{cases} 1 & \?m?b?o?x?{if } a^{[2](i)…
根据所有的预测数据,你还可以如下计算损失J:
J
=
?
1
m
∑
i
=
0
m
(
y
(
i
)
log
?
(
a
[
2
]
(
i
)
)
+
(
1
?
y
(
i
)
)
log
?
(
1
?
a
[
2
]
(
i
)
)
)
(6)
J = - \frac{1}{m} \sum\limits_{i = 0}^{m} \large\left(\small y^{(i)}\log\left(a^{[2] (i)}\right) + (1-y^{(i)})\log\left(1- a^{[2] (i)}\right) \large \right) \small \tag{6}
J=?m1?i=0∑m?(y(i)log(a[2](i))+(1?y(i))log(1?a[2](i)))(6)
提示: 建立神经网络的一般方法是: 1.定义神经网络结构(输入单元数,隐藏单元数等)。 2.初始化模型的参数 3.循环:
- 实现前向传播
- 计算损失
- 后向传播以获得梯度
- 更新参数(梯度下降)
我们通常会构建辅助函数来计算第1-3步,然后将它们合并为nn_model() 函数。一旦构建了nn_model() 并学习了正确的参数,就可以对新数据进行预测。
4.1- 定义神经网络结构
练习:定义三个变量: - n_x:输入层的大小 - n_h:隐藏层的大小(将其设置为4) - n_y:输出层的大小
提示:使用shape来找到n_x和n_y。 另外,将隐藏层大小硬编码为4。
In [7]:
def layer_sizes(X, Y):
"""
Arguments:
X -- input dataset of shape (input size, number of examples)
Y -- labels of shape (output size, number of examples)
Returns:
n_x -- the size of the input layer
n_h -- the size of the hidden layer
n_y -- the size of the output layer
"""
n_x = X.shape[0]
n_h = 4
n_y = Y.shape[0]
return (n_x, n_h, n_y)
In [8]:
X_assess, Y_assess = layer_sizes_test_case()
(n_x, n_h, n_y) = layer_sizes(X_assess, Y_assess)
print("The size of the input layer is: n_x = " + str(n_x))
print("The size of the hidden layer is: n_h = " + str(n_h))
print("The size of the output layer is: n_y = " + str(n_y))
The size of the input layer is: n_x = 5
The size of the hidden layer is: n_h = 4
The size of the output layer is: n_y = 2
预期输出(仅用于评估刚刚编码的函数,并不代表实际网络大小)。 输入层的大小为:n_x = 5 隐藏层的大小为:n_h = 4 输出层的大小为:n_y = 2
4.2- 初始化模型的参数
练习:实现函数 initialize_parameters() 。
说明:
- 请确保参数大小正确。 如果需要,也可参考上面的神经网络图。
- 使用随机值初始化权重矩阵。
- 使用:np.random.randn(a,b)* 0.01 随机初始化维度为(a,b)的矩阵。 - 将偏差向量初始化为零。
- 使用:np.zeros((a,b)) 初始化维度为(a,b)零的矩阵。
In [9]:
def initialize_parameters(n_x, n_h, n_y):
"""
Argument:
n_x -- size of the input layer
n_h -- size of the hidden layer
n_y -- size of the output layer
Returns:
params -- python dictionary containing your parameters:
W1 -- weight matrix of shape (n_h, n_x)
b1 -- bias vector of shape (n_h, 1)
W2 -- weight matrix of shape (n_y, n_h)
b2 -- bias vector of shape (n_y, 1)
"""
np.random.seed(2)
W1 = np.random.randn(n_h,n_x) * 0.01
b1 = np.zeros((n_h,1))
W2 = np.random.randn(n_y,n_h) * 0.01
b2 = np.zeros((n_y,1))
assert (W1.shape == (n_h, n_x))
assert (b1.shape == (n_h, 1))
assert (W2.shape == (n_y, n_h))
assert (b2.shape == (n_y, 1))
parameters = {"W1": W1,
"b1": b1,
"W2": W2,
"b2": b2}
return parameters
In [10]:
n_x, n_h, n_y = initialize_parameters_test_case()
parameters = initialize_parameters(n_x, n_h, n_y)
print("W1 = " + str(parameters["W1"]))
print("b1 = " + str(parameters["b1"]))
print("W2 = " + str(parameters["W2"]))
print("b2 = " + str(parameters["b2"]))
W1 = [[-0.00416758 -0.00056267]
[-0.02136196 0.01640271]
[-0.01793436 -0.00841747]
[ 0.00502881 -0.01245288]]
b1 = [[0.]
[0.]
[0.]
[0.]]
W2 = [[-0.01057952 -0.00909008 0.00551454 0.02292208]]
b2 = [[0.]]
预期输出: W1 = [[-0.00416758 -0.00056267] [-0.02136196 0.01640271] [-0.01793436 -0.00841747] [ 0.00502881 -0.01245288]] b1 = [[0.] [0.] [0.] [0.]] W2 = [[-0.01057952 -0.00909008 0.00551454 0.02292208]] b2 = [[0.]]
4.3- 循环
问题:实现forward_propagation() 。
说明:
- 在上方查看分类器的数学表示形式。
- 你可以使用内置在笔记本中的
sigmoid() 函数。 - 你也可以使用numpy库中的
np.tanh() 函数。 - 必须执行以下步骤:
1.使用parameters [“ ..”] 从字典“ parameters”(这是initialize_parameters() 的输出)中检索出每个参数。 2.实现正向传播,计算Z[1],A[1],Z[2] 和 A[2] (所有训练数据的预测结果向量)。 - 向后传播所需的值存储在
cache 中, cache 将作为反向传播函数的输入。
In [11]:
def forward_propagation(X, parameters):
"""
Argument:
X -- input data of size (n_x, m)
parameters -- python dictionary containing your parameters (output of initialization function)
Returns:
A2 -- The sigmoid output of the second activation
cache -- a dictionary containing "Z1", "A1", "Z2" and "A2"
"""
W1 = parameters["W1"]
b1 = parameters["b1"]
W2 = parameters["W2"]
b2 = parameters["b2"]
Z1 = np.dot(W1,X) + b1
A1 = np.tanh(Z1)
Z2 = np.dot(W2,A1) + b2
A2 = sigmoid(Z2)
assert(A2.shape == (1, X.shape[1]))
cache = {"Z1": Z1,
"A1": A1,
"Z2": Z2,
"A2": A2}
return A2, cache
In [12]:
X_assess, parameters = forward_propagation_test_case()
A2, cache = forward_propagation(X_assess, parameters)
print(np.mean(cache['Z1']) ,np.mean(cache['A1']),np.mean(cache['Z2']),np.mean(cache['A2']))
-0.0004997557777419913 -0.000496963353231779 0.00043818745095914653 0.500109546852431
预期输出: -0.0004997557777419913 -0.000496963353231779 0.00043818745095914653 0.500109546852431
现在,你已经计算了包含每个示例的a2 的 A[2] (在Python变量“A2 ”中),其中,你可以计算损失函数 如下:
J
=
?
1
m
∑
i
=
0
m
(
y
(
i
)
log
?
(
a
[
2
]
(
i
)
)
+
(
1
?
y
(
i
)
)
log
?
(
1
?
a
[
2
]
(
i
)
)
)
(13)
J = - \frac{1}{m} \sum\limits_{i = 0}^{m} \large{(} \small y^{(i)}\log\left(a^{[2] (i)}\right) + (1-y^{(i)})\log\left(1- a^{[2] (i)}\right) \large{)} \small\tag{13}
J=?m1?i=0∑m?(y(i)log(a[2](i))+(1?y(i))log(1?a[2](i)))(13)
练习:实现compute_cost() 以计算损失J的值。
说明:
- 有很多种方法可以实现交叉熵损失。 我们为你提供了实现方法:
?
∑
i
=
0
m
y
(
i
)
log
?
(
a
[
2
]
(
i
)
)
-\sum\limits_{i=0}^{m} y^{(i)}\log(a^{[2](i)})
?i=0∑m?y(i)log(a[2](i)) :
logprobs = np.multiply(np.log(A2),Y)
cost = - np.sum(logprobs)
(你也可以使用np.multiply()然后使用np.sum()或直接使用np.dot())。
In [13]:
def compute_cost(A2, Y, parameters):
"""
Computes the cross-entropy cost given in equation (13)
Arguments:
A2 -- The sigmoid output of the second activation, of shape (1, number of examples)
Y -- "true" labels vector of shape (1, number of examples)
parameters -- python dictionary containing your parameters W1, b1, W2 and b2
Returns:
cost -- cross-entropy cost given equation (13)
"""
m = Y.shape[1]
logprobs = Y*np.log(A2) + (1-Y)* np.log(1-A2)
cost = -1/m * np.sum(logprobs)
cost = np.squeeze(cost)
assert(isinstance(cost, float))
return cost
In [14]:
A2, Y_assess, parameters = compute_cost_test_case()
print("cost = " + str(compute_cost(A2, Y_assess, parameters)))
cost = 0.6929198937761265
预期输出: cost = 0.6929198937761265
现在,通过使用在正向传播期间计算的缓存,你可以实现后向传播。
问题:实现函数backward_propagation() 。
说明: 反向传播通常是深度学习中最难(最数学)的部分。为了帮助你更好地了解,我们提供了反向传播课程的幻灯片。你将要使用此幻灯片右侧的六个方程式以构建向量化实现。
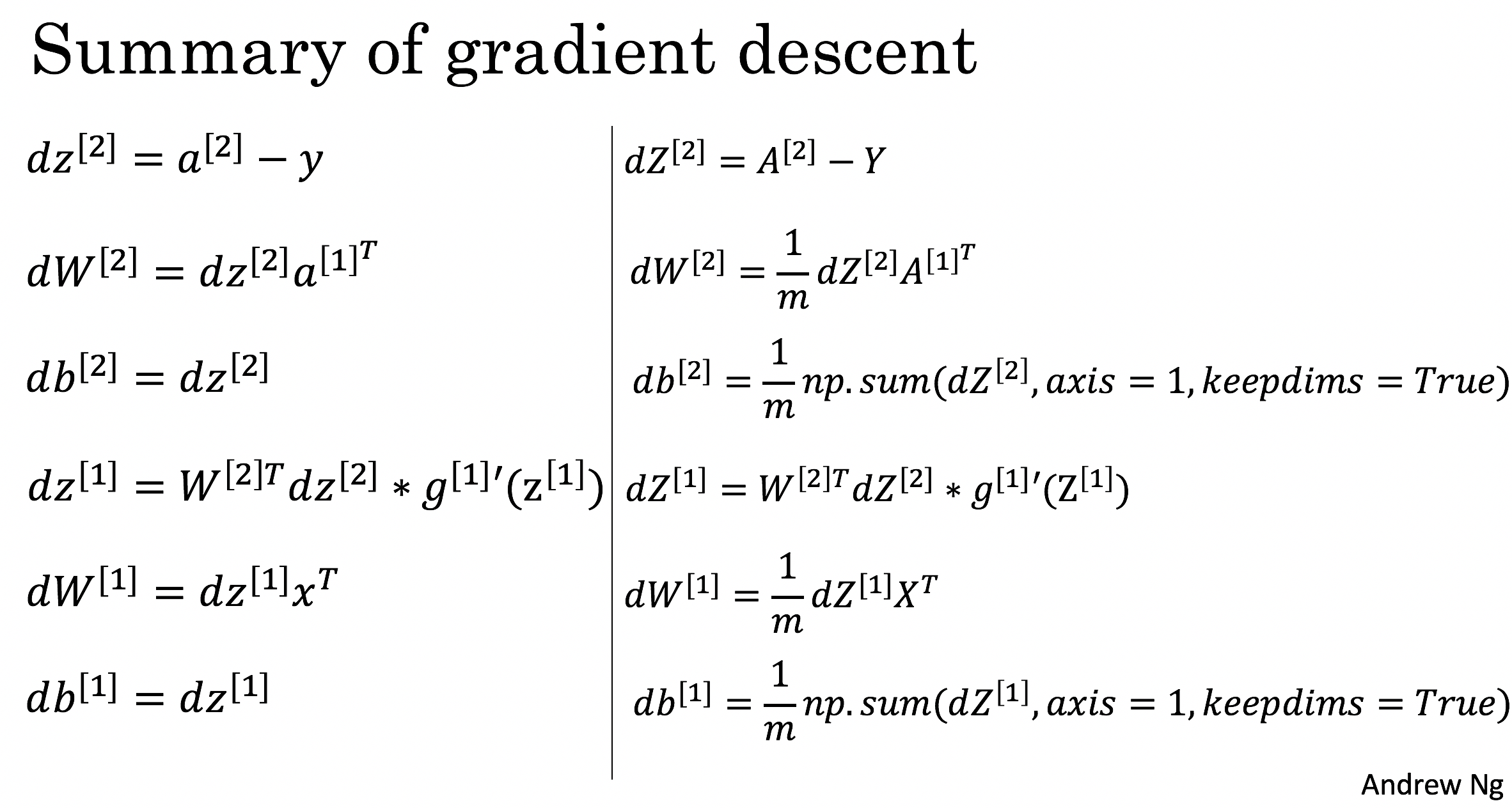
?
J
?
z
2
(
i
)
=
1
m
(
a
[
2
]
(
i
)
?
y
(
i
)
)
\frac{\partial \mathcal{J} }{ \partial z_{2}^{(i)} } = \frac{1}{m} (a^{[2](i)} - y^{(i)})
?z2(i)??J?=m1?(a[2](i)?y(i))
?
J
?
W
2
=
?
J
?
z
2
(
i
)
a
[
1
]
(
i
)
T
\frac{\partial \mathcal{J} }{ \partial W_2 } = \frac{\partial \mathcal{J} }{ \partial z_{2}^{(i)}} a^{[1](i)T}
?W2??J?=?z2(i)??J?a[1](i)T
?
J
?
b
2
=
∑
i
?
J
?
z
2
(
i
)
\frac{\partial \mathcal{J} }{ \partial b_2 } = \sum_i{\frac{\partial \mathcal{J} }{ \partial z_{2}^{(i)}}}
?b2??J?=i∑??z2(i)??J?
?
J
?
z
1
(
i
)
=
W
2
T
?
J
?
z
2
(
i
)
?
(
1
?
a
[
1
]
(
i
)
2
)
\frac{\partial \mathcal{J} }{ \partial z_{1}^{(i)} } = W_2^T \frac{\partial \mathcal{J} }{ \partial z_{2}^{(i)} } * ( 1 - a^{[1](i)2})
?z1(i)??J?=W2T??z2(i)??J??(1?a[1](i)2)
?
J
?
W
1
=
?
J
?
z
1
(
i
)
X
T
\frac{\partial \mathcal{J} }{ \partial W_1 } = \frac{\partial \mathcal{J} }{ \partial z_{1}^{(i)}} X^T
?W1??J?=?z1(i)??J?XT
?
J
i
?
b
1
=
∑
i
?
J
?
z
1
(
i
)
\frac{\partial \mathcal{J} _i }{ \partial b_1 } = \sum_i{\frac{\partial \mathcal{J} }{ \partial z_{1}^{(i)}}}
?b1??Ji??=i∑??z1(i)??J?
- 请注意,? 表示元素乘法。
- 你将使用在深度学习中很常见的编码表示方法:
- dW1 =
?
J
?
W
1
\frac{\partial \mathcal{J} }{ \partial W_1 }
?W1??J?
- db1 =
?
J
?
b
1
\frac{\partial \mathcal{J} }{ \partial b_1 }
?b1??J?
- dW2 =
?
J
?
W
2
\frac{\partial \mathcal{J} }{ \partial W_2 }
?W2??J?
- db2 =
?
J
?
b
2
\frac{\partial \mathcal{J} }{ \partial b_2 }
?b2??J?
- 提示:
-要计算dZ1,你首先需要计算
g
[
1
]
′
(
Z
[
1
]
)
g^{[1]'}(Z^{[1]})
g[1]′(Z[1])。由于
g
[
1
]
(
.
)
g^{[1]}(.)
g[1](.) 是tanh激活函数,因此如果
a
=
g
[
1
]
(
z
)
a = g^{[1]}(z)
a=g[1](z) 则
g
[
1
]
′
(
z
)
=
1
?
a
2
g^{[1]'}(z) = 1-a^2
g[1]′(z)=1?a2。所以你可以使用(1 - np.power(A1, 2)) 计算
g
[
1
]
′
(
Z
[
1
]
)
g^{[1]'}(Z^{[1]})
g[1]′(Z[1])。
In [15]:
def backward_propagation(parameters, cache, X, Y):
"""
Implement the backward propagation using the instructions above.
Arguments:
parameters -- python dictionary containing our parameters
cache -- a dictionary containing "Z1", "A1", "Z2" and "A2".
X -- input data of shape (2, number of examples)
Y -- "true" labels vector of shape (1, number of examples)
Returns:
grads -- python dictionary containing your gradients with respect to different parameters
"""
m = X.shape[1]
W1 = parameters["W1"]
W2 = parameters["W2"]
A1 = cache["A1"]
A2 = cache["A2"]
dZ2= A2 - Y
dW2 = 1 / m * np.dot(dZ2,A1.T)
db2 = 1 / m * np.sum(dZ2,axis=1,keepdims=True)
dZ1 = np.dot(W2.T,dZ2) * (1-np.power(A1,2))
dW1 = 1 / m * np.dot(dZ1,X.T)
db1 = 1 / m * np.sum(dZ1,axis=1,keepdims=True)
grads = {"dW1": dW1,
"db1": db1,
"dW2": dW2,
"db2": db2}
return grads
In [16]:
parameters, cache, X_assess, Y_assess = backward_propagation_test_case()
grads = backward_propagation(parameters, cache, X_assess, Y_assess)
print ("dW1 = "+ str(grads["dW1"]))
print ("db1 = "+ str(grads["db1"]))
print ("dW2 = "+ str(grads["dW2"]))
print ("db2 = "+ str(grads["db2"]))
dW1 = [[ 0.01018708 -0.00708701]
[ 0.00873447 -0.0060768 ]
[-0.00530847 0.00369379]
[-0.02206365 0.01535126]]
db1 = [[-0.00069728]
[-0.00060606]
[ 0.000364 ]
[ 0.00151207]]
dW2 = [[ 0.00363613 0.03153604 0.01162914 -0.01318316]]
db2 = [[0.06589489]]
预期输出: dW1 = [[ 0.01018708 -0.00708701] [ 0.00873447 -0.0060768 ] [-0.00530847 0.00369379] [-0.02206365 0.01535126]] db1 = [[-0.00069728] [-0.00060606] [ 0.000364 ] [ 0.00151207]] dW2 = [[ 0.00363613 0.03153604 0.01162914 -0.01318316]] db2 = [[0.06589489]]
问题:实现参数更新。 使用梯度下降,你必须使用(dW1,db1,dW2,db2)才能更新(W1,b1,W2,b2)。
一般的梯度下降规则:
θ
=
θ
?
α
?
J
?
θ
\theta = \theta - \alpha \frac{\partial J }{ \partial \theta }
θ=θ?α?θ?J?其中α是学习率,而θ 代表一个参数。
图示:具有良好的学习速率(收敛)和较差的学习速率(发散)的梯度下降算法。 图片由Adam Harley提供。
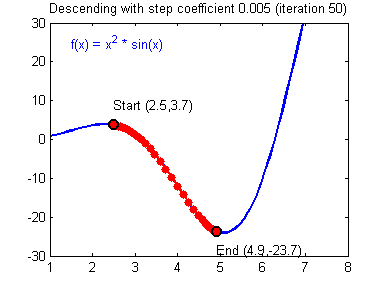
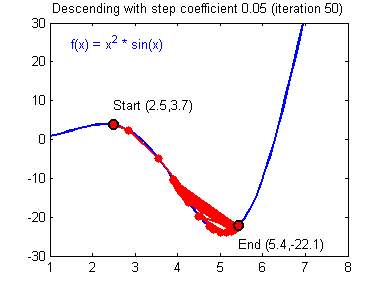
In [17]:
def update_parameters(parameters, grads, learning_rate = 1.2):
"""
Updates parameters using the gradient descent update rule given above
Arguments:
parameters -- python dictionary containing your parameters
grads -- python dictionary containing your gradients
Returns:
parameters -- python dictionary containing your updated parameters
"""
W1 = parameters["W1"]
b1 = parameters["b1"]
W2 = parameters["W2"]
b2 = parameters["b2"]
dW1 = grads["dW1"]
db1 = grads["db1"]
dW2 = grads["dW2"]
db2 = grads["db2"]
W1 = W1 - learning_rate * dW1
b1 = b1 - learning_rate * db1
W2 = W2 - learning_rate * dW2
b2 = b2 - learning_rate * db2
parameters = {"W1": W1,
"b1": b1,
"W2": W2,
"b2": b2}
return parameters
In [18]:
parameters, grads = update_parameters_test_case()
parameters = update_parameters(parameters, grads)
print("W1 = " + str(parameters["W1"]))
print("b1 = " + str(parameters["b1"]))
print("W2 = " + str(parameters["W2"]))
print("b2 = " + str(parameters["b2"]))
W1 = [[-0.00643025 0.01936718]
[-0.02410458 0.03978052]
[-0.01653973 -0.02096177]
[ 0.01046864 -0.05990141]]
b1 = [[-1.02420756e-06]
[ 1.27373948e-05]
[ 8.32996807e-07]
[-3.20136836e-06]]
W2 = [[-0.01041081 -0.04463285 0.01758031 0.04747113]]
b2 = [[0.00010457]]
预期输出: dW1 = [[ 0.01018708 -0.00708701] [ 0.00873447 -0.0060768 ] [-0.00530847 0.00369379] [-0.02206365 0.01535126]] db1 = [[-0.00069728] [-0.00060606] [ 0.000364 ] [ 0.00151207]] dW2 = [[ 0.00363613 0.03153604 0.01162914 -0.01318316]] db2 = [[0.06589489]]
4.4- 在nn_model()中集成4.1、4.2和4.3部分中的函数
问题:在nn_model()中建立你的神经网络模型。
说明:神经网络模型必须以正确的顺序组合先前构建的函数。
In [19]:
def nn_model(X, Y, n_h, num_iterations = 10000, print_cost=False):
"""
Arguments:
X -- dataset of shape (2, number of examples)
Y -- labels of shape (1, number of examples)
n_h -- size of the hidden layer
num_iterations -- Number of iterations in gradient descent loop
print_cost -- if True, print the cost every 1000 iterations
Returns:
parameters -- parameters learnt by the model. They can then be used to predict.
"""
np.random.seed(3)
n_x = layer_sizes(X, Y)[0]
n_y = layer_sizes(X, Y)[2]
parameters = initialize_parameters(n_x, n_h, n_y)
W1 = parameters["W1"]
b1 = parameters["b1"]
W2 = parameters["W2"]
b2 = parameters["b2"]
for i in range(0, num_iterations):
A2, cache = forward_propagation(X, parameters)
cost = compute_cost(A2, Y, parameters)
grads = backward_propagation(parameters, cache, X, Y)
parameters = update_parameters(parameters, grads)
if print_cost and i % 1000 == 0:
print ("Cost after iteration %i: %f" %(i, cost))
return parameters
In [20]:
X_assess, Y_assess = nn_model_test_case()
parameters = nn_model(X_assess, Y_assess, 4, num_iterations=10000, print_cost=False)
print("W1 = " + str(parameters["W1"]))
print("b1 = " + str(parameters["b1"]))
print("W2 = " + str(parameters["W2"]))
print("b2 = " + str(parameters["b2"]))
/opt/conda/lib/python3.6/site-packages/ipykernel_launcher.py:20: RuntimeWarning: divide by zero encountered in log
/home/kesci/work/planar_utils.py:34: RuntimeWarning: overflow encountered in exp
s = 1/(1+np.exp(-x))
W1 = [[-4.18503197 5.33214315]
[-7.52988635 1.24306559]
[-4.19302427 5.32627154]
[ 7.52984762 -1.24308746]]
b1 = [[ 2.32926944]
[ 3.79460252]
[ 2.33002498]
[-3.79466751]]
W2 = [[-6033.83668723 -6008.12983227 -6033.10091631 6008.06624417]]
b2 = [[-52.66610924]]
预期输出: W1 = [[-4.18503197 5.33214315] [-7.52988635 1.24306559] [-4.19302427 5.32627154] [ 7.52984762 -1.24308746]] b1 = [[ 2.32926944] [ 3.79460252] [ 2.33002498] [-3.79466751]] W2 = [[-6033.83668723 -6008.12983227 -6033.10091631 6008.06624417]] b2 = [[-52.66610924]]
4.5- 预测
问题:使用你的模型通过构建predict()函数进行预测。 使用正向传播来预测结果。
提示:
y
p
r
e
d
i
c
t
i
o
n
=
1
activation?>?0.5
=
{
1
if?
a
c
t
i
v
a
t
i
o
n
>
0.5
0
otherwise
y_{prediction} = \mathbb 1 \text{{activation > 0.5}} = \begin{cases} 1 & \text{if}\ activation > 0.5 \\ 0 & \text{otherwise} \end{cases}
yprediction?=1activation?>?0.5={10?if?activation>0.5otherwise?
例如,如果你想基于阈值将矩阵X设为0和1,则可以执行以下操作: X_new = (X > threshold)
In [21]:
def predict(parameters, X):
"""
Using the learned parameters, predicts a class for each example in X
Arguments:
parameters -- python dictionary containing your parameters
X -- input data of size (n_x, m)
Returns
predictions -- vector of predictions of our model (red: 0 / blue: 1)
"""
A2, cache = forward_propagation(X, parameters)
predictions = np.round(A2)
return predictions
In [22]:
parameters, X_assess = predict_test_case()
predictions = predict(parameters, X_assess)
print("predictions mean = " + str(np.mean(predictions)))
predictions mean = 0.6666666666666666
预期输出: predictions mean = 0.6666666666666666
现在运行模型以查看其如何在二维数据集上运行。 运行以下代码以使用含有nh隐藏单元的单个隐藏层测试模型。
In [23]:
parameters = nn_model(X, Y, n_h = 4, num_iterations = 10000, print_cost=True)
plot_decision_boundary(lambda x: predict(parameters, x.T), X, Y)
plt.title("Decision Boundary for hidden layer size " + str(4))
Cost after iteration 0: 0.693048
Cost after iteration 1000: 0.288083
Cost after iteration 2000: 0.254385
Cost after iteration 3000: 0.233864
Cost after iteration 4000: 0.226792
Cost after iteration 5000: 0.222644
Cost after iteration 6000: 0.219731
Cost after iteration 7000: 0.217504
Cost after iteration 8000: 0.219467
Cost after iteration 9000: 0.218561
Out[23]:
Text(0.5, 1.0, 'Decision Boundary for hidden layer size 4')
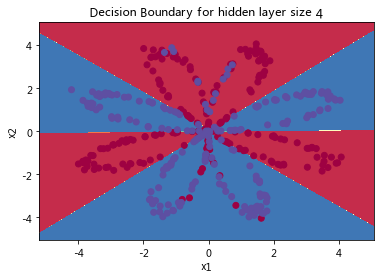
预期输出: Cost after iteration 9000: 0.218561
In [24]:
predictions = predict(parameters, X)
print ('Accuracy: %d' % float((np.dot(Y,predictions.T) + np.dot(1-Y,1-predictions.T))/float(Y.size)*100) + '%')
Accuracy: 90%
预期输出: Accuracy: 90%
与Logistic回归相比,准确性确实更高。 该模型学习了flower的叶子图案! 与逻辑回归不同,神经网络甚至能够学习非线性的决策边界。
现在,让我们尝试几种不同的隐藏层大小。
4.6- 调整隐藏层大小(可选练习)
运行以下代码(可能需要1-2分钟), 你将观察到不同大小隐藏层的模型的不同表现。
In [25]:
plt.figure(figsize=(16, 32))
hidden_layer_sizes = [1, 2, 3, 4, 5, 10, 20]
for i, n_h in enumerate(hidden_layer_sizes):
plt.subplot(5, 2, i+1)
plt.title('Hidden Layer of size %d' % n_h)
parameters = nn_model(X, Y, n_h, num_iterations = 5000)
plot_decision_boundary(lambda x: predict(parameters, x.T), X, Y)
predictions = predict(parameters, X)
accuracy = float((np.dot(Y,predictions.T) + np.dot(1-Y,1-predictions.T))/float(Y.size)*100)
print ("Accuracy for {} hidden units: {} %".format(n_h, accuracy))
Accuracy for 1 hidden units: 67.5 %
Accuracy for 2 hidden units: 67.25 %
Accuracy for 3 hidden units: 90.75 %
Accuracy for 4 hidden units: 90.5 %
Accuracy for 5 hidden units: 91.25 %
Accuracy for 10 hidden units: 90.25 %
Accuracy for 20 hidden units: 90.5 %
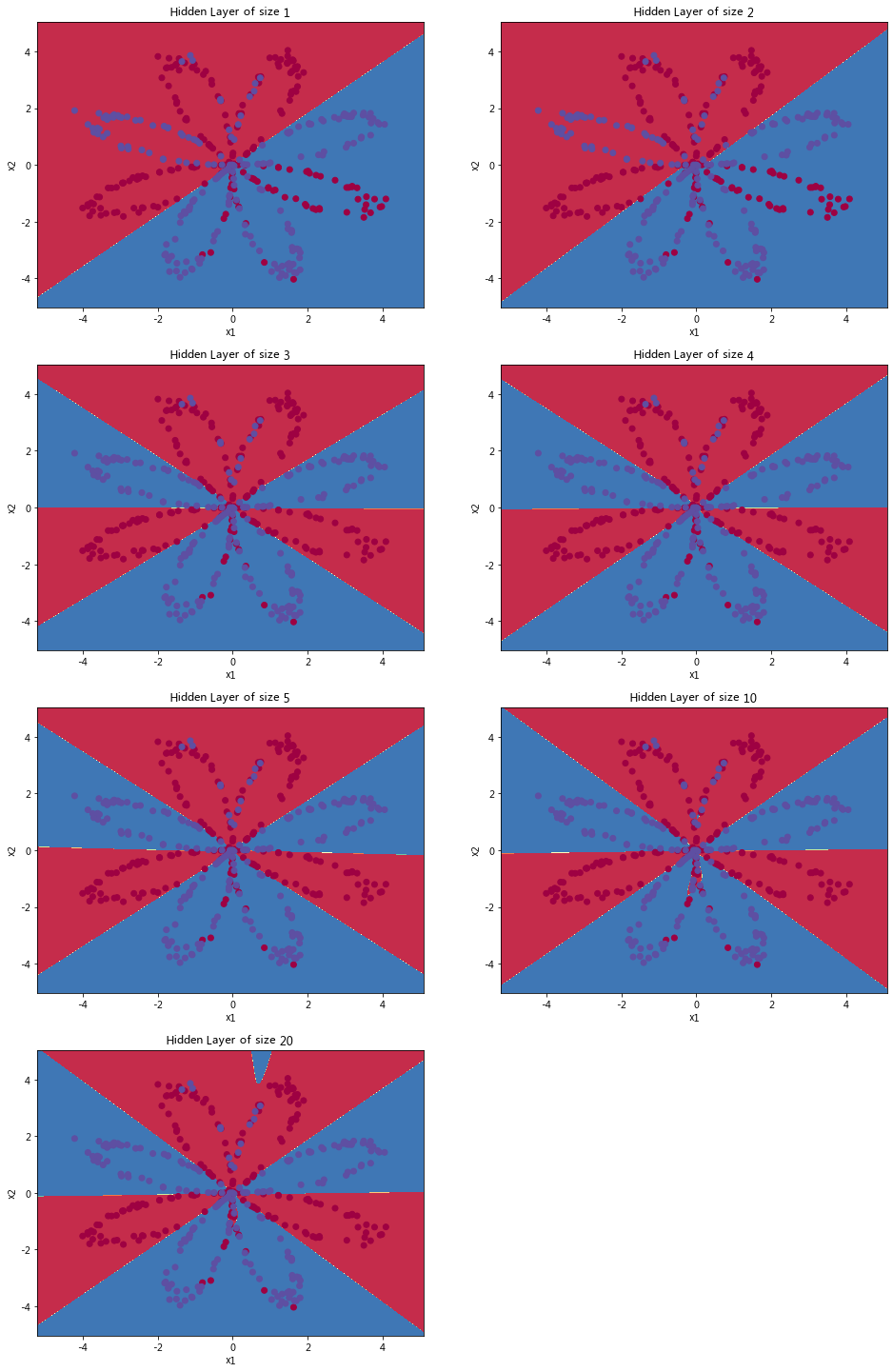
说明:
- 较大的模型(具有更多隐藏的单元)能够更好地拟合训练集,直到最终最大的模型过拟合数据为止。
- 隐藏层的最佳大小似乎在n_h = 5左右。的确,此值似乎很好地拟合了数据,而又不会引起明显的过度拟合。
- 稍后你还将学习正则化,帮助构建更大的模型(例如n_h = 50)而不会过度拟合。
可选问题:
注意:请单击右上角的"Submit Assignment"蓝色按钮以提交作业。
如果你愿意,可以探索一些可选的问题:
- 将tanh激活函数更改为sigmoid或ReLU会发生什么?
- 调整学习率会发生什么?
- 如果我们更改数据集该怎么办? (请参阅下面的第5部分!)
你学习了以下几点:
- 建立具有隐藏层的完整神经网络
- 善用非线性单位
- 实现正向传播和反向传播,并训练神经网络
- 了解不同隐藏层大小(包括过度拟合)的影响。
5- 模型在其他数据集上的性能
如果需要,可以为以下每个数据集重新运行整个笔记本(除去数据集部分)。
In [27]:
noisy_circles, noisy_moons, blobs, gaussian_quantiles, no_structure = load_extra_datasets()
datasets = {"noisy_circles": noisy_circles,
"noisy_moons": noisy_moons,
"blobs": blobs,
"gaussian_quantiles": gaussian_quantiles}
dataset = "gaussian_quantiles"
X, Y = datasets[dataset]
X, Y = X.T, Y.reshape(1, Y.shape[0])
if dataset == "blobs":
Y = Y%2
plt.scatter(X[0, :], X[1, :], c=Y.reshape(X[0,:].shape), s=40, cmap=plt.cm.Spectral);
![[外链图片转存中...(img-clk2FMrW-1662728706538)]](https://img-blog.csdnimg.cn/2f20b38ae6574a04839c3065d6c01c47.png)
恭喜你完成了此编程作业!
参考:
- http://scs.ryerson.ca/~aharley/neural-networks/
- http://cs231n.github.io/neural-networks-case-study/
|