论文地址:https://arxiv.org/pdf/2010.11929.pdf
声明
本文来源于 Reference 列出的大佬讲解,以及部分碎片知识,仅供本人学习并在后期有新的感悟进行知识更新,再此表达对大佬最诚挚的敬意,如有侵权,本人立即删除!
一、引言
- transformer 中首先需要将字符分词后转化为数字,然后每个词用一个向量表示,那么图片呢?
- 在整合最后输出信息的时候,有多种?式:?种是使?
CLS token ,另?种就是对所有 tokens 的输出做?个平均
- 为什么需要位置编码?
RNN 考虑了输入时序的问题,而注意力机制是并行输入,不存在时许问题,不融入位置关系就会导致,比如 我 爱 你, 我 你 爱, 爱 我 你 等不同顺序输入后输出相同的结果。 - VIT 与 Transformer 结构有何不同
Transformer 中在多头注意力以及前馈神经网络之后做 LN, 而 VIT 是在之前做 LN
二、模型详解
-
模型由三个模块组成:
- Linear Projection of Flattened Patches (Embedding层)
- Transformer Encoder (图右侧有给出更加详细的结构)
- MLP Head(最终用于分类的层结构)
-
Embedding 层结构详解 对于标准的 Transformer 模块,要求输入的是 token(向量)序列,即二维矩阵 [num_token, token_dim],如下图,token0-9 对应的都是向量,以 ViT-B/16 为例,每个 token 向量长度为 768。 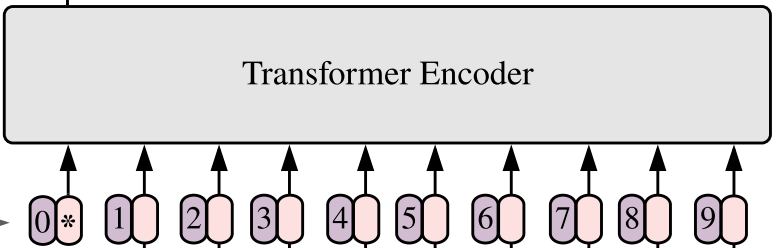 对于图像数据而言,其数据格式为 [H, W, C] 是三维矩阵明显不是 Transformer 想要的。所以需要先通过一个Embedding 层来对数据做个变换。如下图所示,首先将一张图片按给定大小分成一堆 Patches。以 ViT-B/16 为例,将输入图片 (224x224) 按照 16x16 大小的 Patch 进行划分,划分后会得到 ( 224 / 16 ) 2 = 196 个 Patches。接着通过线性映射将每个 Patch 映射到一维向量中,以 ViT-B/16 为例,每个 Patche 数据 shape 为 [16, 16, 3] 通过映射得到一个长度为 768 的向量(后面都直接称为 token )。[16, 16, 3] -> [768] 在代码实现中,直接通过一个卷积层来实现。 以 ViT-B/16 为例,直接使用一个卷积核大小为 16x16 ,步距为 16 ,卷积核个数为 768 的卷积来实现。通过卷积 [224, 224, 3] -> [14, 14, 768] ,然后把 H 以及 W 两个维度展平即可 [14, 14, 768] -> [196, 768] ,此时正好变成了一个二维矩阵,正是 Transformer 想要的。 在输入 Transformer Encoder 之前注意需要加上 [class] token 以及 Position Embedding。 在原论文中,作者说参考 BERT,在刚刚得到的一堆 tokens 中插入一个专门用于分类的 [class] token,这个 [class] token 是一个可训练的参数,数据格式和其他 token 一样都是一个向量,以 ViT-B/16 为例,就是一个长度为 768 的向量,与之前从图片中生成的 tokens 拼接在一起,Cat([1, 768], [196, 768]) -> [197, 768] 。然后关于 Position Embedding 就是之前 Transformer 中讲到的 Positional Encoding,这里的 Position Embedding 采用的是一个可训练的参数(1D Pos. Emb.),是直接叠加在 tokens 上的(add),所以 shape 要一样。以 ViT-B/16 为例,刚刚拼接 [class] token 后 shape 是 [197, 768],那么这里的 Position Embedding 的 shape 也是 [197, 768] 。 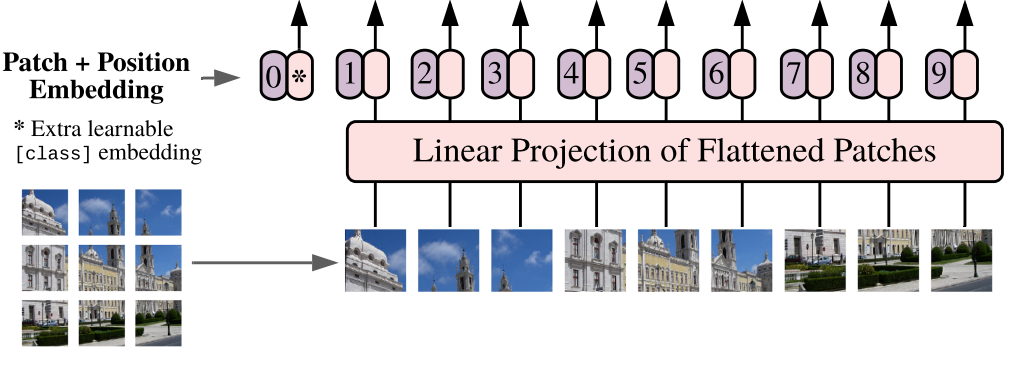 对于 Position Embedding 作者也有做一系列对比试验,在源码中默认使用的是1D Pos. Emb. ,对比不使用 Position Embedding 准确率提升了大概 3 个点,和 2D Pos. Emb. 比起来没太大差别。 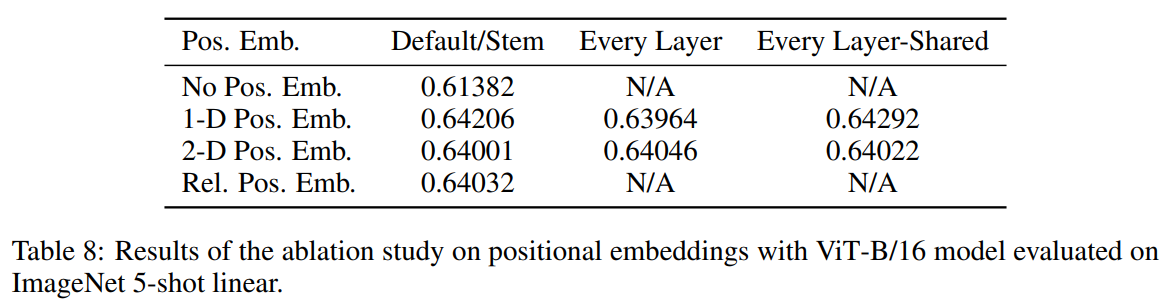
Patch 代码实现
class PatchEmbed(nn.Module):
"""
2D Image to Patch Embedding
"""
def __init__(self, img_size=224, patch_size=16, in_c=3, embed_dim=768, norm_layer=None):
super().__init__()
img_size = (img_size, img_size)
patch_size = (patch_size, patch_size)
self.img_size = img_size
self.patch_size = patch_size
self.grid_size = (img_size[0] // patch_size[0], img_size[1] // patch_size[1])
self.num_patches = self.grid_size[0] * self.grid_size[1]
self.proj = nn.Conv2d(in_c, embed_dim, kernel_size=patch_size, stride=patch_size)
self.norm = norm_layer(embed_dim) if norm_layer else nn.Identity()
def forward(self, x):
B, C, H, W = x.shape
assert H == self.img_size[0] and W == self.img_size[1], \
f"Input image size ({H}*{W}) doesn't match model ({self.img_size[0]}*{self.img_size[1]})."
x = self.proj(x).flatten(2).transpose(1, 2)
x = self.norm(x)
return x
Attention 代码实现
class Attention(nn.Module):
def __init__(self,
dim,
num_heads=8,
qkv_bias=False,
qk_scale=None,
attn_drop_ratio=0.,
proj_drop_ratio=0.):
super(Attention, self).__init__()
self.num_heads = num_heads
head_dim = dim // num_heads
self.scale = qk_scale or head_dim ** -0.5
self.qkv = nn.Linear(dim, dim * 3, bias=qkv_bias)
self.attn_drop = nn.Dropout(attn_drop_ratio)
self.proj = nn.Linear(dim, dim)
self.proj_drop = nn.Dropout(proj_drop_ratio)
def forward(self, x):
B, N, C = x.shape
qkv = self.qkv(x).reshape(B, N, 3, self.num_heads, C // self.num_heads).permute(2, 0, 3, 1, 4)
q, k, v = qkv[0], qkv[1], qkv[2]
attn = (q @ k.transpose(-2, -1)) * self.scale
attn = attn.softmax(dim=-1)
attn = self.attn_drop(attn)
x = (attn @ v).transpose(1, 2).reshape(B, N, C)
x = self.proj(x)
x = self.proj_drop(x)
return x
图中的 Encoder block 代码实现
class Block(nn.Module):
def __init__(self,
dim,
num_heads,
mlp_ratio=4.,
qkv_bias=False,
qk_scale=None,
drop_ratio=0.,
attn_drop_ratio=0.,
drop_path_ratio=0.,
act_layer=nn.GELU,
norm_layer=nn.LayerNorm):
super(Block, self).__init__()
self.norm1 = norm_layer(dim)
self.attn = Attention(dim, num_heads=num_heads, qkv_bias=qkv_bias, qk_scale=qk_scale,
attn_drop_ratio=attn_drop_ratio, proj_drop_ratio=drop_ratio)
self.drop_path = DropPath(drop_path_ratio) if drop_path_ratio > 0. else nn.Identity()
self.norm2 = norm_layer(dim)
mlp_hidden_dim = int(dim * mlp_ratio)
self.mlp = Mlp(in_features=dim, hidden_features=mlp_hidden_dim, act_layer=act_layer, drop=drop_ratio)
def forward(self, x):
x = x + self.drop_path(self.attn(self.norm1(x)))
x = x + self.drop_path(self.mlp(self.norm2(x)))
return x
-
MLP Head 详解 上面通过 Transformer Encoder 后输出的 shape 和输入的 shape 是保持不变的,以 ViT-B/16 为例,输入的是[197, 768] 输出的还是 [197, 768] 。注意,在 Transformer Encoder 后其实还有一个 Layer Norm 没有画出来,后面有作者 [1] 画的 ViT 的模型可以看到详细结构。这里只需要分类的信息,所以只需要提取出 [class] token 生成的对应结果就行,即 [197, 768] 中抽取出 [class] token 对应的 [1, 768] 。接着我们通过 MLP Head 得到我们最终的分类结果。MLP Head 原论文中说在训练 ImageNet21K 时是由 Linear+tanh 激活函数 + Linear 组成。但是迁移到ImageNet1K 上或者你自己的数据上时,只用一个 Linear 即可。
MLP 代码实现
class Mlp(nn.Module):
"""
MLP as used in Vision Transformer, MLP-Mixer and related networks
"""
def __init__(self, in_features, hidden_features=None, out_features=None, act_layer=nn.GELU, drop=0.):
super().__init__()
out_features = out_features or in_features
hidden_features = hidden_features or in_features
self.fc1 = nn.Linear(in_features, hidden_features)
self.act = act_layer()
self.fc2 = nn.Linear(hidden_features, out_features)
self.drop = nn.Dropout(drop)
def forward(self, x):
x = self.fc1(x)
x = self.act(x)
x = self.drop(x)
x = self.fc2(x)
x = self.drop(x)
return x
综上处理后 Vision Transformer 代码实现
class VisionTransformer(nn.Module):
def __init__(self, img_size=224, patch_size=16, in_c=3, num_classes=1000,
embed_dim=768, depth=12, num_heads=12, mlp_ratio=4.0, qkv_bias=True,
qk_scale=None, representation_size=None, distilled=False, drop_ratio=0.,
attn_drop_ratio=0., drop_path_ratio=0., embed_layer=PatchEmbed, norm_layer=None,
act_layer=None):
"""
Args:
img_size (int, tuple): input image size
patch_size (int, tuple): patch size
in_c (int): number of input channels
num_classes (int): number of classes for classification head
embed_dim (int): embedding dimension
depth (int): depth of transformer
num_heads (int): number of attention heads
mlp_ratio (int): ratio of mlp hidden dim to embedding dim
qkv_bias (bool): enable bias for qkv if True
qk_scale (float): override default qk scale of head_dim ** -0.5 if set
representation_size (Optional[int]): enable and set representation layer (pre-logits) to this value if set
distilled (bool): model includes a distillation token and head as in DeiT models
drop_ratio (float): dropout rate
attn_drop_ratio (float): attention dropout rate
drop_path_ratio (float): stochastic depth rate
embed_layer (nn.Module): patch embedding layer
norm_layer: (nn.Module): normalization layer
"""
super(VisionTransformer, self).__init__()
self.num_classes = num_classes
self.num_features = self.embed_dim = embed_dim
self.num_tokens = 2 if distilled else 1
norm_layer = norm_layer or partial(nn.LayerNorm, eps=1e-6)
act_layer = act_layer or nn.GELU
self.patch_embed = embed_layer(img_size=img_size, patch_size=patch_size, in_c=in_c, embed_dim=embed_dim)
num_patches = self.patch_embed.num_patches
self.cls_token = nn.Parameter(torch.zeros(1, 1, embed_dim))
self.dist_token = nn.Parameter(torch.zeros(1, 1, embed_dim)) if distilled else None
self.pos_embed = nn.Parameter(torch.zeros(1, num_patches + self.num_tokens, embed_dim))
self.pos_drop = nn.Dropout(p=drop_ratio)
dpr = [x.item() for x in torch.linspace(0, drop_path_ratio, depth)]
self.blocks = nn.Sequential(*[
Block(dim=embed_dim, num_heads=num_heads, mlp_ratio=mlp_ratio, qkv_bias=qkv_bias, qk_scale=qk_scale,
drop_ratio=drop_ratio, attn_drop_ratio=attn_drop_ratio, drop_path_ratio=dpr[i],
norm_layer=norm_layer, act_layer=act_layer)
for i in range(depth)
])
self.norm = norm_layer(embed_dim)
if representation_size and not distilled:
self.has_logits = True
self.num_features = representation_size
self.pre_logits = nn.Sequential(OrderedDict([
("fc", nn.Linear(embed_dim, representation_size)),
("act", nn.Tanh())
]))
else:
self.has_logits = False
self.pre_logits = nn.Identity()
self.head = nn.Linear(self.num_features, num_classes) if num_classes > 0 else nn.Identity()
self.head_dist = None
if distilled:
self.head_dist = nn.Linear(self.embed_dim, self.num_classes) if num_classes > 0 else nn.Identity()
nn.init.trunc_normal_(self.pos_embed, std=0.02)
if self.dist_token is not None:
nn.init.trunc_normal_(self.dist_token, std=0.02)
nn.init.trunc_normal_(self.cls_token, std=0.02)
self.apply(_init_vit_weights)
def forward_features(self, x):
x = self.patch_embed(x)
cls_token = self.cls_token.expand(x.shape[0], -1, -1)
if self.dist_token is None:
x = torch.cat((cls_token, x), dim=1)
else:
x = torch.cat((cls_token, self.dist_token.expand(x.shape[0], -1, -1), x), dim=1)
x = self.pos_drop(x + self.pos_embed)
x = self.blocks(x)
x = self.norm(x)
if self.dist_token is None:
return self.pre_logits(x[:, 0])
else:
return x[:, 0], x[:, 1]
def forward(self, x):
x = self.forward_features(x)
if self.head_dist is not None:
x, x_dist = self.head(x[0]), self.head_dist(x[1])
if self.training and not torch.jit.is_scripting():
return x, x_dist
else:
return (x + x_dist) / 2
else:
x = self.head(x)
return x
Vision Transformer 网络结构细节回顾
Reference
[1] Vision Transformer 详解 [2] Vision Transformer(ViT)PyTorch代码全解析(附图解)
|