语义分割
Language-driven semantic segmentation
Language-driven semantic segmentation (LSeg)
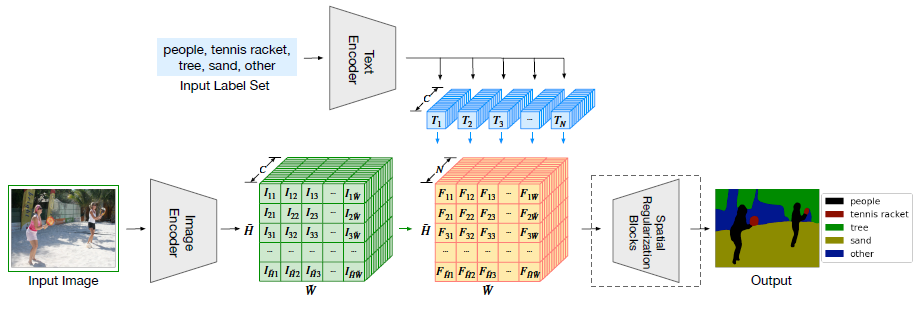
- Text encoder:text encoder 将
N
N
N 个 labels 嵌入到向量空间
R
C
\R^C
RC 中,得到
N
N
N 个向量
T
1
,
.
.
.
,
T
n
∈
R
C
T_1,...,T_n\in\R^C
T1?,...,Tn?∈RC. 作者这里直接使用 CLIP 中的 text encoder,并且在训练中冻结 text encoder 的参数
- Image encoder:作者使用 dense prediction transformers (DPT) 结构 (ViT + decoder) 抽取出
I
∈
R
H
~
×
W
~
×
C
I\in\R^{\tilde H\times\tilde W\times C}
I∈RH~×W~×C 的图像特征,其中
H
~
=
H
/
s
,
W
~
=
W
/
s
\tilde H=H/s,\tilde W=W/s
H~=H/s,W~=W/s,
s
=
2
s=2
s=2 为降采样系数,
I
i
j
I_{ij}
Iij? 可以看作 pixel
(
i
,
j
)
(i, j)
(i,j) 的 embedding
- Word-pixel correlation tensor:计算
N
N
N 个 label embedding 和
H
~
×
W
~
\tilde H\times\tilde W
H~×W~ 个 pixel embedding 间的内积,得到 Word-pixel correlation tensor
F
∈
R
H
~
×
W
~
×
N
F\in\R^{\tilde H\times\tilde W\times N}
F∈RH~×W~×N
F
i
j
=
(
f
i
j
1
,
.
.
.
,
f
i
j
N
)
T
F_{ij}=(f_{ij1},...,f_{ijN})^T
Fij?=(fij1?,...,fijN?)T 表示 pixel
(
i
,
j
)
(i,j)
(i,j) 和各个 label 间的相似度,在训练时对每个像素
F
i
j
F_{ij}
Fij? 用 softmax + cross entropy 使得 pixel embedding 和 GT label embedding 尽可能接近即可 其中,
t
t
t 为温度参数 - Spatial regularization:regularizes and upsamples the predictions to the original input resolution. During this process, we have to ensure that all operations stay equivariant with respect to the labels. In other words, there should be no interactions between the input channels, whose order is defined by the order of the words and can thus be arbitrary. We evaluate two functions that fulfill this property:
In a final step we use bilinear interpolation to recover predictions at the original resolution.
Experiments
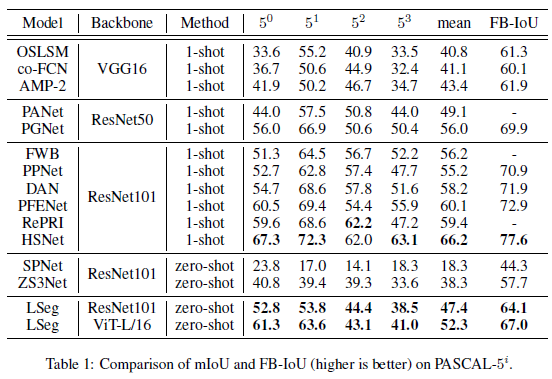
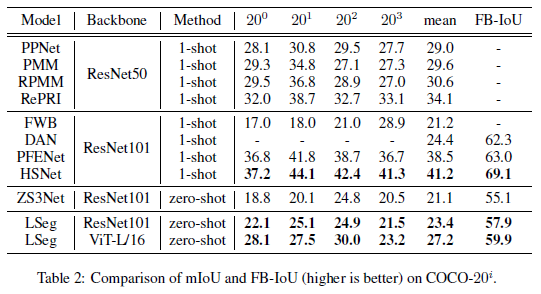
可以看到,LSeg 比之前的 zero-shot 方法有所进步,但性能仍显著低于有监督方法 (1-shot)
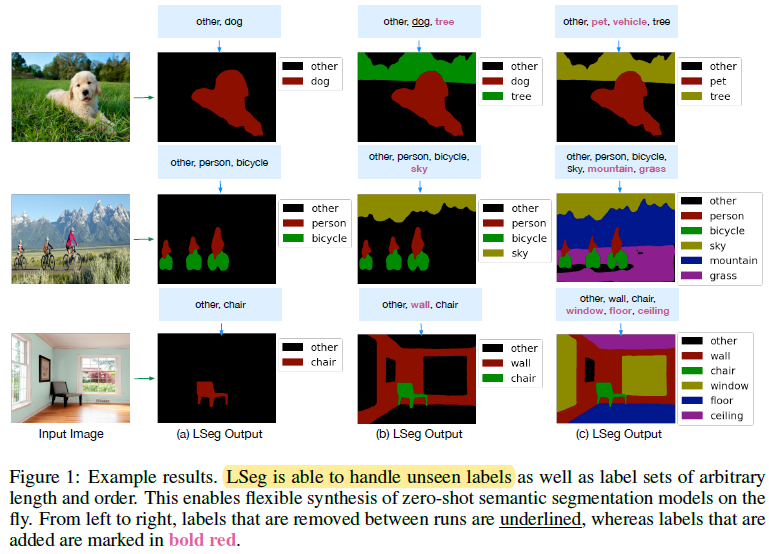
Failure cases
- 当测试时图片某个像素不属于任何给定标签时,LSeg 就会把该像素分类给与 pixel embed 最接近的 label embed 对应的标签。如左图所示,模型将 dog 分配给了 label “toy”
- The model focuses on a single most likely object when multiple explanations are consistent with the label set. In this specific example, the windows of the house are labeled as “house” instead of window, even thought the label “window” is available as a choice.
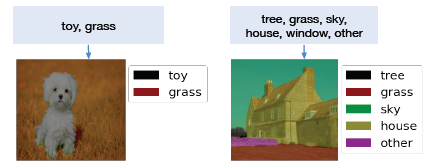
GroupViT: Semantic Segmentation Emerges From Text Supervision
- LSeg 虽然用了 CLIP 的 text encoder,但它仍然需要依赖于数据集标签 Segmentation mask,无法将文本作为监督信号来进行无监督训练。GroupViT 则不需要借助 Segmentation mask,它可以利用图像文本对来进行无监督训练
Architecture
- Grouping Block:Grouping 是无监督分割中的一种技术。假设图像上有一些聚类中心,grouping 的思想就是从这些聚类中心发散出去,把周围相似的点逐渐扩充为一个 group 来组成 segmentation mask,从而自下而上地进行分割。作者就是使用了 grouping 的思想,引入了可训练的 group tokens 和 grouping block,其中 group tokens 就可以看作是当前的聚类中心。grouping block 输入为 image segment tokens (i.e. image tokens) 和 group tokens,它首先通过计算 image segment tokens
{
s
^
i
l
}
\{\hat s_i^l\}
{s^il?} 和 group tokens
{
g
^
i
l
}
\{\hat g_i^l\}
{g^?il?} 之间的相似度来将每个 image segment token 分配给一个 group token,也就是分配给一个簇。具体而言,由 投影+内积+Softmax 的方法可以得到
s
^
j
l
\hat s_j^l
s^jl? 属于
g
^
i
l
\hat g_i^l
g^?il? 的非归一化概率
exp
?
(
W
q
g
^
i
l
?
W
k
s
^
j
l
)
\exp(W_q\hat g_i^l\cdot W_k\hat s_j^l)
exp(Wq?g^?il??Wk?s^jl?),我们要做的就是根据
s
^
j
l
\hat s_j^l
s^jl? 属于各个 group token 的概率进行采样,根据采样结果选择要分配的簇,但采样操作是不可导的,因此需要使用 Gumbel Softmax,取
τ
=
1
\tau=1
τ=1 后,由 Gumbel softmax 采样得到的
s
^
j
l
\hat s_j^l
s^jl? 属于
g
^
i
l
\hat g_i^l
g^?il? 的概率
A
i
,
j
l
A_{i,j}^l
Ai,jl? 为
其中,
{
γ
i
}
~
G
u
m
b
e
l
(
0
,
1
)
\{\gamma_i\}\sim Gumbel(0,1)
{γi?}~Gumbel(0,1). 在经过 argmax + one-hot 操作后可以将 gumbel softmax 得到的概率采样向量近似为 one-hot 的采样向量。在反向传播时则可以使用 straight through trick: 这样前向传播时,image segment tokens 都被各自分配给了一个 group token (i.e. hard assignment),反向传播时梯度则为
A
l
A^l
Al 的梯度。在分配完毕后,grouping block 将所有属于同一个簇的 image segment tokens 进行合并得到一个新的 image segment token
s
l
+
1
s^{l+1}
sl+1,对于簇
i
i
i,合并方法如下: 其中,
∑
j
M
l
?
1
A
^
i
,
j
l
\sum_{j}^{M_{l-1}}\hat A_{i,j}^l
∑jMl?1??A^i,jl? 为属于簇
i
i
i 的 image segment token 数,
∑
j
M
l
?
1
A
^
i
,
j
l
W
v
s
^
j
l
\sum_{j}^{M_{l-1}}\hat A_{i,j}^lW_v\hat s_j^l
∑jMl?1??A^i,jl?Wv?s^jl? 为对所有属于簇
i
i
i 的 image segment token 投影后进行求和 (除了 hard assignment,也可以用 soft assignment,即用 softmax 得到的采样向量
A
l
A^l
Al 替代上式中的
A
^
l
\hat A^l
A^l,但实验证明 hard assignment 效果更好). 最终,grouping block 通过融合具有相似语义的 image segment tokens 来得到
M
l
?
1
M_{l-1}
Ml?1? 个融合后的 image segment tokens,其中
M
l
?
1
M_{l-1}
Ml?1? 为输入的 group token 数 (It merges all the segment tokens that are assigned to the same group token into a single new image segment, based on similarity in the embedding space.) 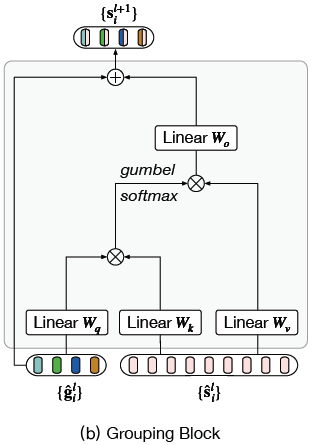 - Multi-stage Grouping:主体框架采用 ViT,将输入图像处理为
N
N
N 个 image tokens (i.e. patch embeds)
{
s
i
1
}
i
=
1
N
\{s_i^1\}_{i=1}^N
{si1?}i=1N?,同时还设置了 2 个 grouping stages,这两个 stage 中分别设置 64 和 8 个 group tokens 作为聚类中心 (The lower stage groups pixels into object parts, e.g., noses and legs of elephants; and the higher stage further merges them into entire objects, e.g., the whole elephant and the background forest.). 经过 avg pooling 和 MLP 后可以得到 image embed
z
I
z^I
zI
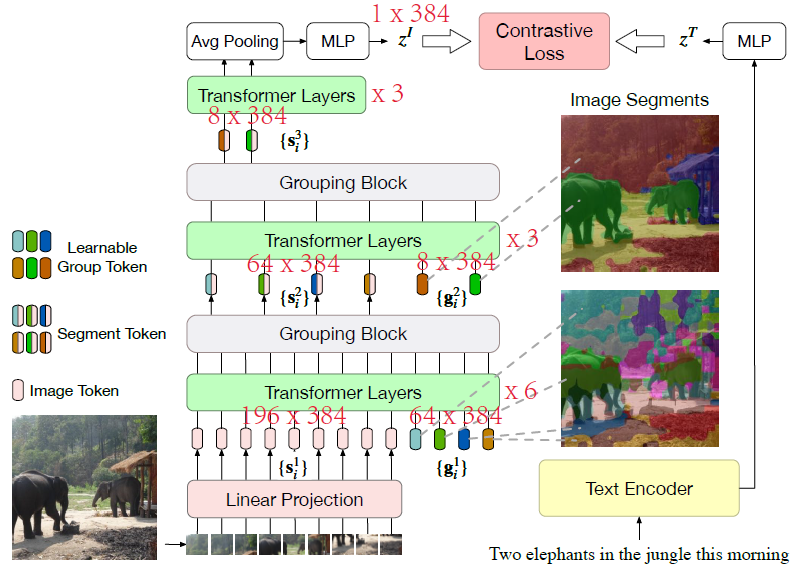
Learning from Image-Text Pairs
- Image-Text Contrastive Loss:
(1) image-to-text contrastive loss (2) text-to-image contrastive loss  - Multi-Label Image-Text Contrastive Loss:使用 prompting engineering,从原始文本
x
i
T
x_i^T
xiT? 中抽取出
K
K
K 个名词组成新的文本
{
x
i
T
k
}
k
=
1
K
\{x_i^{T_k}\}_{k=1}^K
{xiTk??}k=1K? (“A photo of a {noun}”),这样一个 batch 里就有
K
K
K 个正样本对和
K
(
B
?
1
)
K(B-1)
K(B?1) 个负样本对,损失函数如下:
   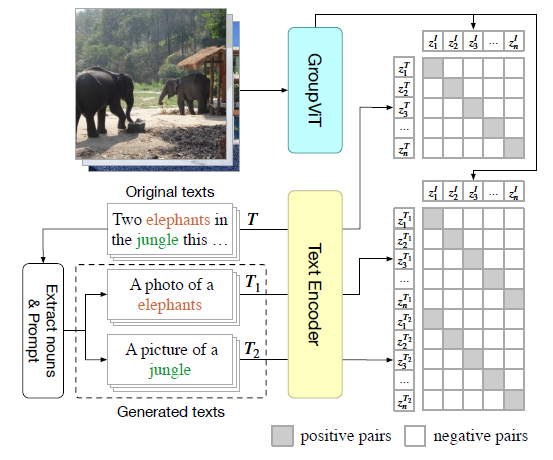
Zero-Shot Transfer to Semantic Segmentation
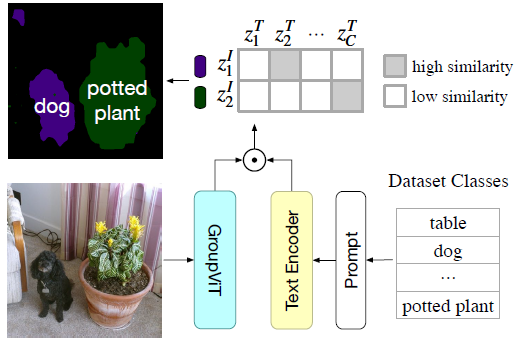
- 在做 zero-shot 分割的时候,作者直接计算 avg pooling 前的 8 个 image segment tokens 和各个类别对应文本之间的相似度来决定这 8 个 image segment tokens 分别属于哪一类 (如果相似度小于阈值,就将 image segment token 分为背景类)。而每个 segment token 又对应图像中的 arbitrarily-shaped region,具体对应哪些区域可以由
∏
l
=
1
L
A
^
l
\prod_{l=1}^L\hat A^l
∏l=1L?A^l 得到,其中
A
^
l
\hat A^l
A^l 为
l
l
l-th grouping stage 的 assignment matrix (缺点也很明显,就是同一张图里最多只能分割出 8 个类别)
Experiments 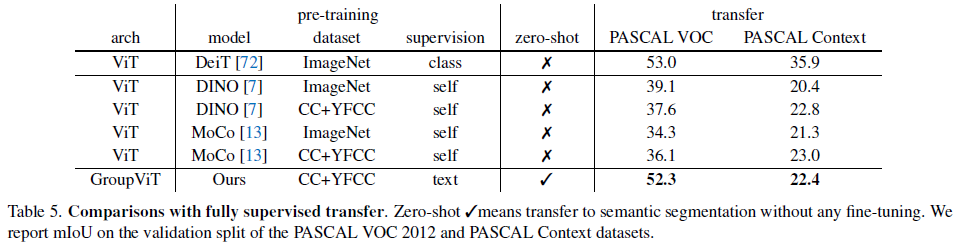
- 可以看到,group tokens 确实起到了聚类中心的作用,并且 stage 越深,group token 关注的范围也就越大
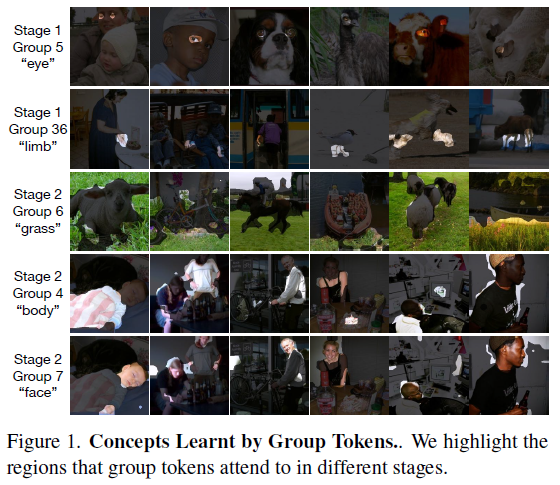
目标检测
Open-vocabulary object detection via vision and language knowledge distillation
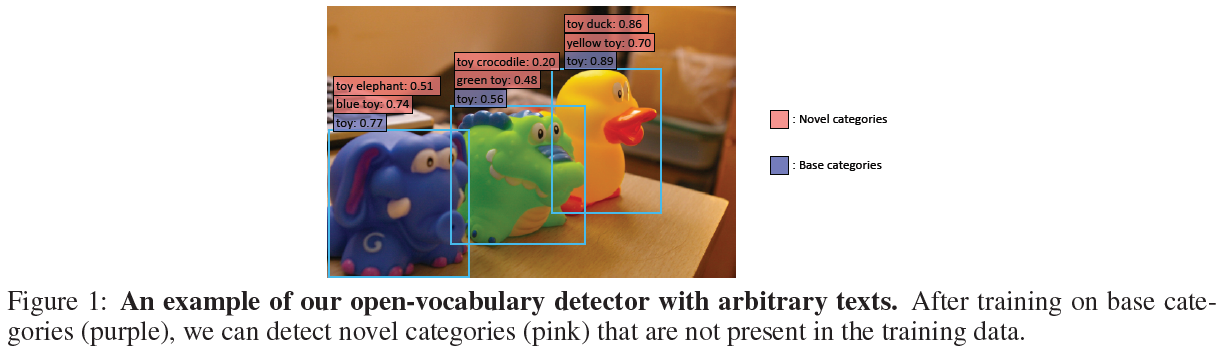
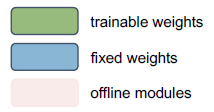
- Vanilla detector (baseline):The classification head of a vanilla two-stage detector, e.g., Mask R-CNN.
N
N
N proposals 为 RPN 阶段生成的
N
N
N 个 proposals,Head 为每个 proposal 生成 region embed,最后送入 classifier 计算分类和回归损失
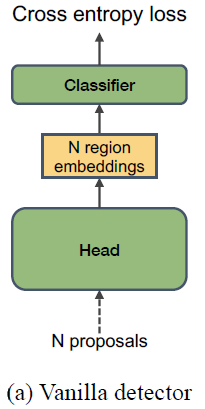 - ViLD-text:ViLD-text 将 classifier 替换为了 fixed text embeddings 和一个 learnable background embedding (背景类包含了所有不在基础类别中的类),其中 fixed text embeddings 是由数据集中的类别经过 prompt engineering 和 clip 的 text encoder 后得到的。也就是说,ViLD-text 是在数据集上利用基础类别进行有监督训练,它的主要目的是把图像特征和文本特征联系到一起
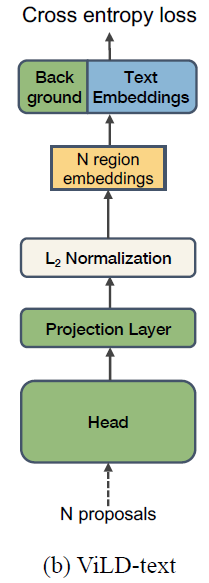 - ViLD-image:把 CLIP 的 text encoder 当作 teacher,用知识蒸馏的方法让模型抽取出更好的图像特征。其中训练时输入的 proposal 以及经过 CLIP 前向传播后的结果是提前计算好的,可以用来节省训练时间
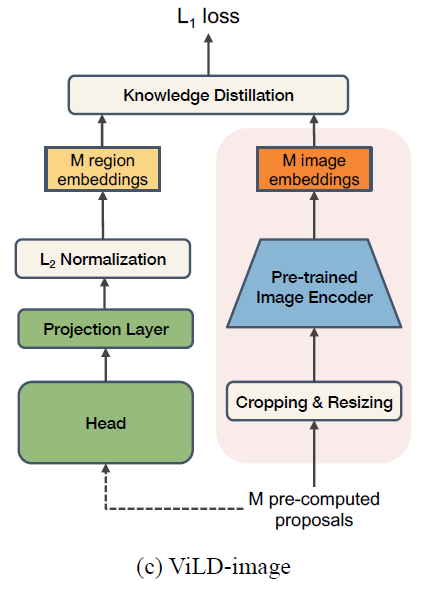 - ViLD:ViLD-text + ViLD-image
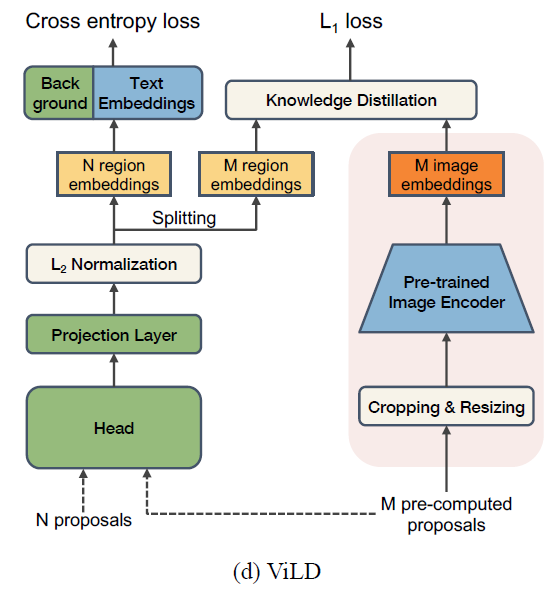
An overview of using ViLD for open-vocabulary object detection
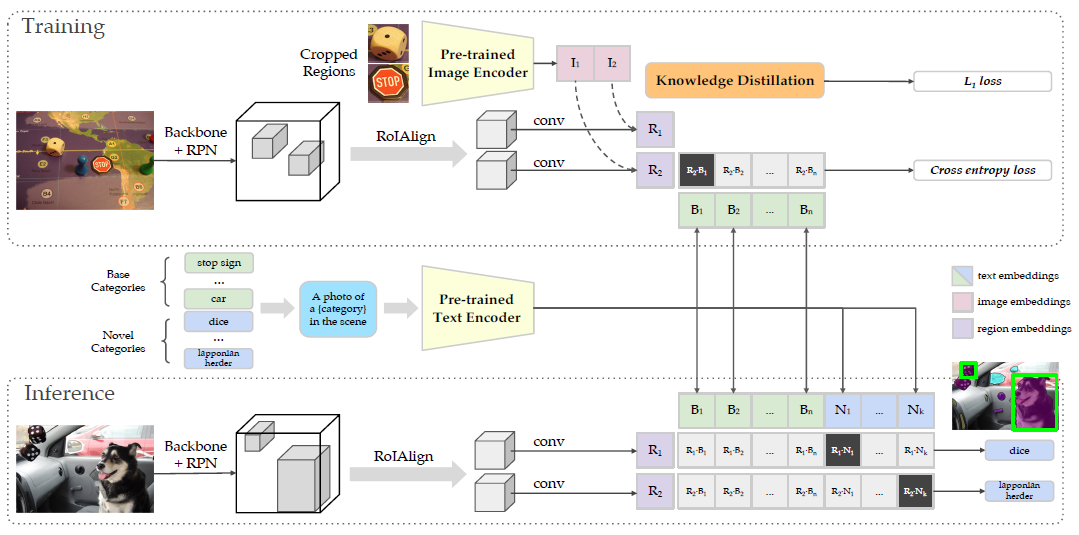
Experiments
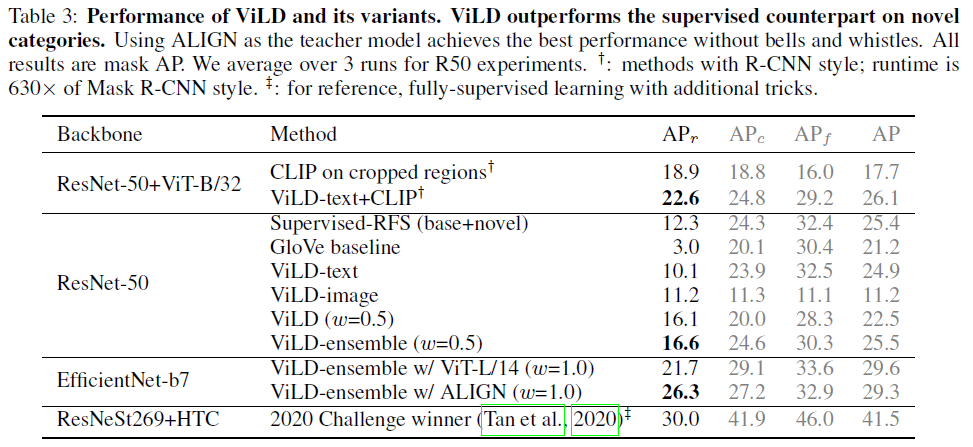
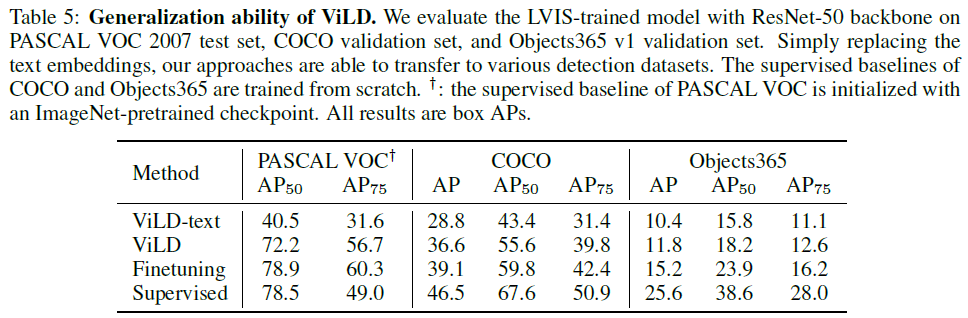
Grounded Language-Image Pre-training (GLIP)
Abstract
- GLIP 在预训练阶段结合了 object detection 和 phrase grounding (i.e. visual grounding) 两个任务,这使得 GLIP 能同时从 detection 和 grounding 数据集中进行学习,同时还能利用大量的 image-text pairs,以自监督的方式生成 grounding boxes (bootstrap a good grounding model)
- GLIP 在 27M grounding data, including 3M human-annotated and 24M web-crawled image-text pairs 上进行训练,在 COCO 和 LVIS 上仅 zero-shot 就分别达到了 49.8 AP 和 26.9 AP,这在 COCO 上超过了一系列有监督的强 baseline,例如 Faster-RCNN w/ResNet50 (40.2) or ResNet101 (42.0), and DyHead w/ Swin-Tiny (49.7). 在 COCO 上 fine-tune 之后则达到了 60.8 AP on val and 61.5 AP on test-dev,直接达到了 SOTA
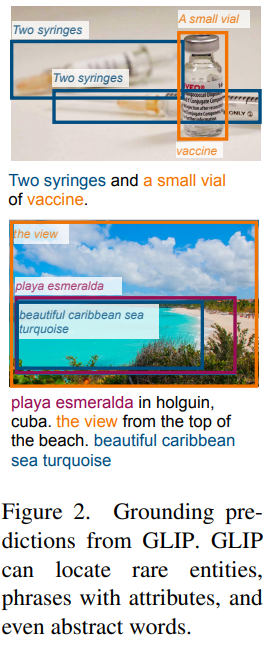
Unified Formulation (把 detection 和 visual grounding 统一到同一个框架下)
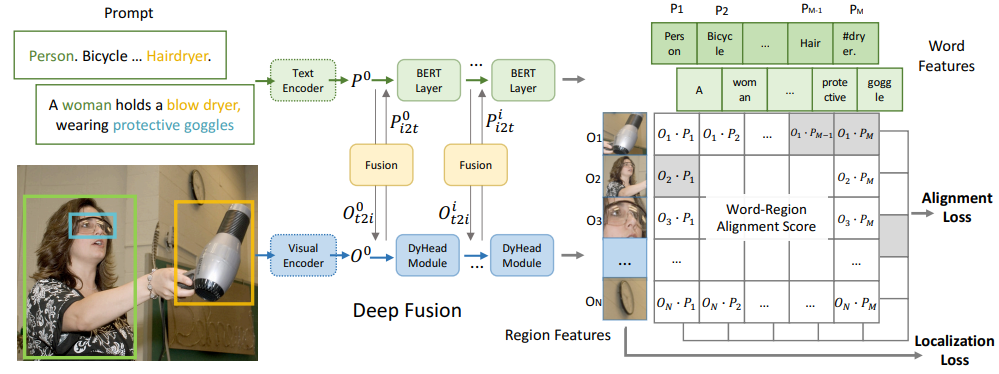
- Background: object detection:典型的 detection model 一般是由 visual encoder
E
n
c
I
Enc_I
EncI? 抽取出图像中的 region/box features
O
∈
R
N
×
d
O\in\R^{N\times d}
O∈RN×d,每个 region/box feature 由 box classifier
C
\mathcal C
C 和 box regressor
R
\mathcal R
R 计算分类损失
L
c
l
s
\mathcal L_{cls}
Lcls? 和 Localization loss
L
l
o
c
\mathcal L_{loc}
Lloc?
分类损失为 其中,
W
∈
R
c
×
d
,
S
c
l
s
∈
R
N
×
c
W\in\R^{c\times d},S_{cls}\in\R^{N\times c}
W∈Rc×d,Scls?∈RN×c 为分类 logits,
c
c
c 为类别数,
T
∈
{
0
,
1
}
N
×
c
T\in\{0,1\}^{N\times c}
T∈{0,1}N×c 为 target matching,
l
o
s
s
(
S
;
T
)
loss(S; T )
loss(S;T) 为 cross-entropy loss - Object detection as phrase grounding:grounding/aligning each region to
c
c
c phrases in a text prompt. Prompt 方式如下 (结尾还有一个特殊 token [NoObj]):
alignment scores
S
g
r
o
u
n
d
S_{ground}
Sground? 计算方式如下: 其中,
O
∈
R
N
×
d
O\in\R^{N\times d}
O∈RN×d,
P
∈
R
M
×
d
P\in \R^{M\times d}
P∈RM×d. 在训练时,只需将分类损失中的
S
c
l
s
S_{cls}
Scls? 替换为
S
g
r
o
u
n
d
S_{ground}
Sground? 即可。另外对于 target
T
T
T 也要做适当的修改。
M
M
M 是 prompt engineering 后的 (sub)-word tokens 数,因此需要讲
T
∈
{
0
,
1
}
N
×
c
T\in\{0,1\}^{N\times c}
T∈{0,1}N×c 扩展为
T
∈
{
0
,
1
}
N
×
M
T\in\{0,1\}^{N\times M}
T∈{0,1}N×M,其中只有要检测的物体对应的所有子词标记为 positive match,其余 token 均为 negative match,在推理时只需取物体对应的所有 tokens 的 average token probabilities 即可 - Language-Aware Deep Fusion:上述过程中,image encoder 和 text encoder 只在最后计算相似度时进行了特征交互,这称为 late-fusion. 为了加强跨模态特征交互,作者增加了 deep fusion,给 image encoder 和 text encoder 分别加了几个新的 encoding layers 用来融合文本和图像信息。用于多模态交互的 cross-modality multi-head attention module (X-MHA) 如下所示:
新添加的
L
L
L 个交互层如下 (使用 DyHead 作为 image encoder,BERT 作为 text encoder): 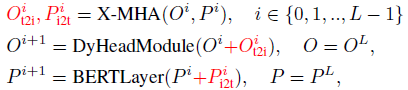
Transfer to Established Benchmarks
- A detailed list of GLIP model variants. self-training:为了扩大预训练数据集,作者用训练好的 GLIP-T ? 给 Caption 数据集中的图像文本对生成伪标签给 GLIP-T 和 GLIP-L 训练
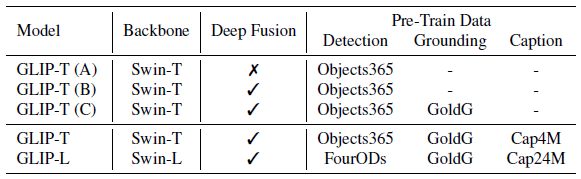 - Zero-shot domain transfer and fine-tuning on COCO
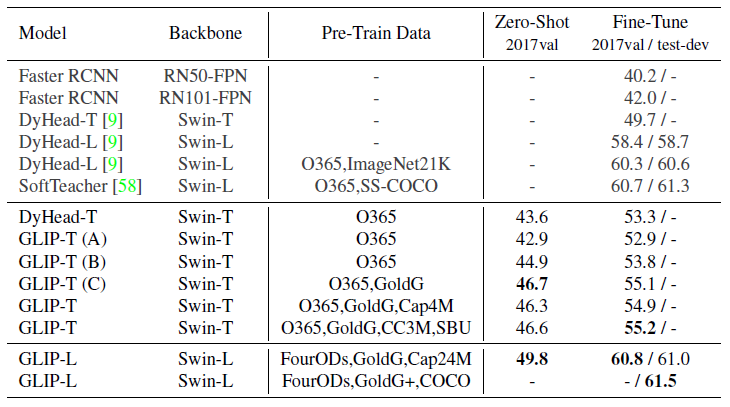
图像生成
CLIPasso: Semantically-Aware Object Sketching
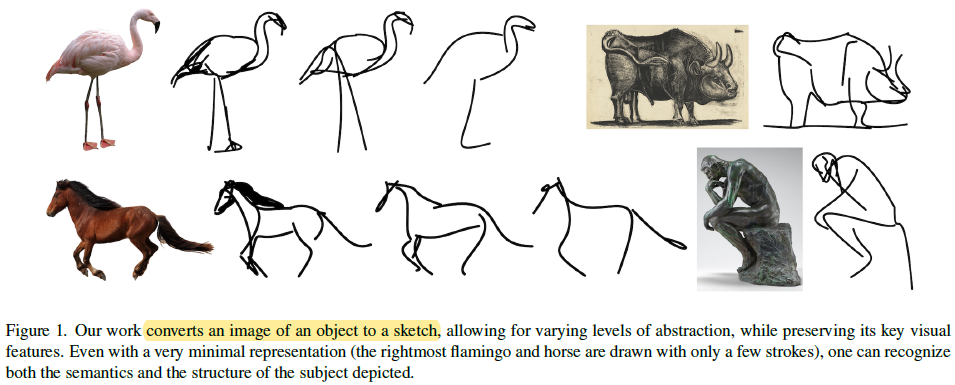
- 任务:在白纸上画贝塞尔/贝兹曲线形成简笔画,每条曲线由 4 个点去控制它的形状,即
s
i
=
{
p
i
j
}
j
=
1
4
=
{
(
x
i
,
y
i
)
j
}
j
=
1
4
s_i=\{p_i^j\}_{j=1}^4=\{(x_i,y_i)^j\}_{j=1}^4
si?={pij?}j=14?={(xi?,yi?)j}j=14? (这里
s
s
s 表示 stroke,即 “笔画”),因此只要调整 4 个点的位置再通过光栅化器 (Rasterizier) 计算相应的贝兹曲线 (这一过程是可导的),就能生成不同形状的曲线。模型的训练目标就是生成
n
n
n 个笔画,也就是
4
n
4n
4n 个点
- 本文的主要贡献点就在于如何更好地初始化以及提出一个更好的损失函数
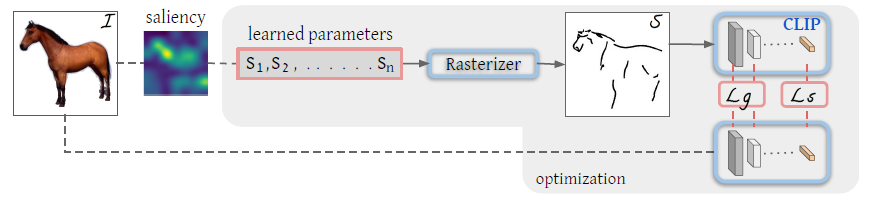
Loss Function
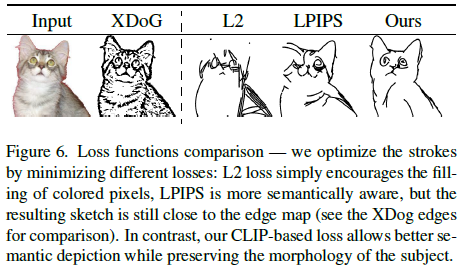
- Semantic loss:CLIP 的 image encoder 具有稳健性,也就是说,不管图像是什么风格,CLIP 都能编码出图像的视觉语义信息。因此作者就使用 CLIP 的 image encoder 分别对原画和简笔画进行编码,然后使它们的语义编码尽量接近。本质上可以看作是把 CLIP 的 image encoder 当作 teacher 来蒸馏自己的模型:
其中,  - Geometric loss:为了使得简笔画的 pose 和 structure 与原图接近,作者还加入了几何损失,即 L2 distance between intermediate level activations of CLIP
作者在实际中使用了 ResNet101 CLIP 的 layers 3 and 4 进行中间特征图的蒸馏 - Total loss
其中,
w
s
=
0.1
w_s = 0.1
ws?=0.1
Strokes Initialization
Results
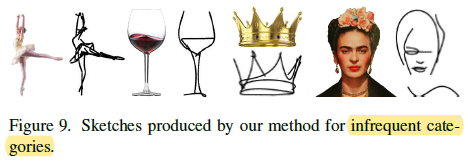 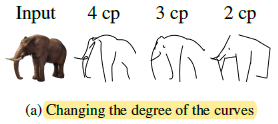 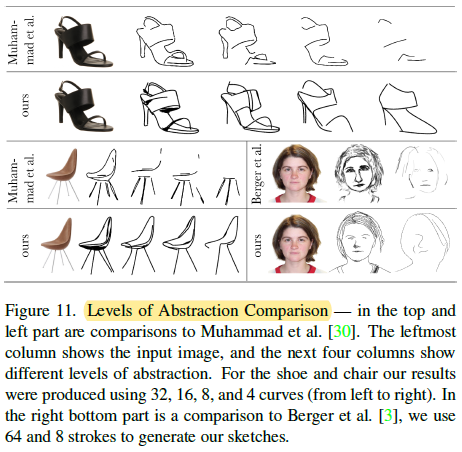
Limitations
- (1) For images with background, our method’s performance is reduced at higher abstraction levels. This limitation can be addressed by using an automatic mask (类似于分割模型,直接把前景分割出来生成它的简笔画).
- (2) Our sketches are not created sequentially and all strokes are optimized simultaneously, which differs from the conventional way of sketching.
- (3) The number of strokes must be determined in advance to achieve the desired level of abstraction. Another possible extension could be to make this a learned parameter, as different images might require different numbers of strokes to reach similar levels of abstraction.
另外我还有一个疑问,这个模型是没有训练环节,在输入图片的时候才在线训练 2000 个 iterations 吗?为什么在 demo 里上传一张图片就要训练 2000 次,感觉好煎熬啊…
视频
CLIP4Clip: An Empirical Study of CLIP for End to End Video Clip Retrieval and Captioning
任务是由文本检索视频
Main structure
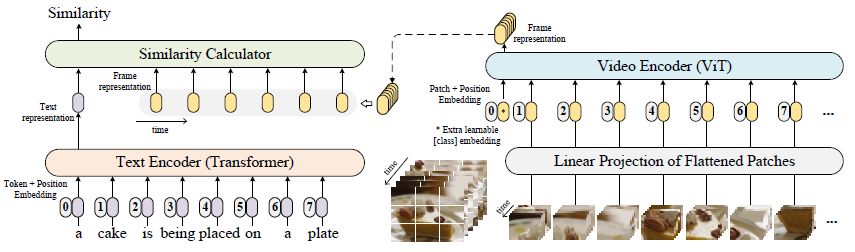
Similarity calculator

- Parameter-free type:uses the mean pooling to aggregate the feature of all frames to obtain an ‘average frame’
其中,
w
j
w_j
wj? 为 caption representation,
z
^
i
=
mean-pooling
(
z
i
1
,
z
i
2
,
.
.
.
,
z
i
∣
v
i
∣
)
\hat z_i=\text{mean-pooling}(z_i^1,z_i^2,...,z_i^{|v_i|})
z^i?=mean-pooling(zi1?,zi2?,...,zi∣vi?∣?). 这种计算相似度的方式虽然没有额外参数,但却忽略了视频帧之间的时序信息 - Sequential type:作者实验了 LSTM 和 Transformer encoder with position embedding
P
P
P 两种结构,在得到带有时序信息的编码后使用 mean pooling 得到视频特征与文本特征计算余弦相似度
- Tight type:直接用 Transformer Encoder 进行 video 和 caption 的多模态交互
其中,
P
P
P 为 position embedding,
T
T
T 为 type embedding,最终的相似度得分为 
Experiments
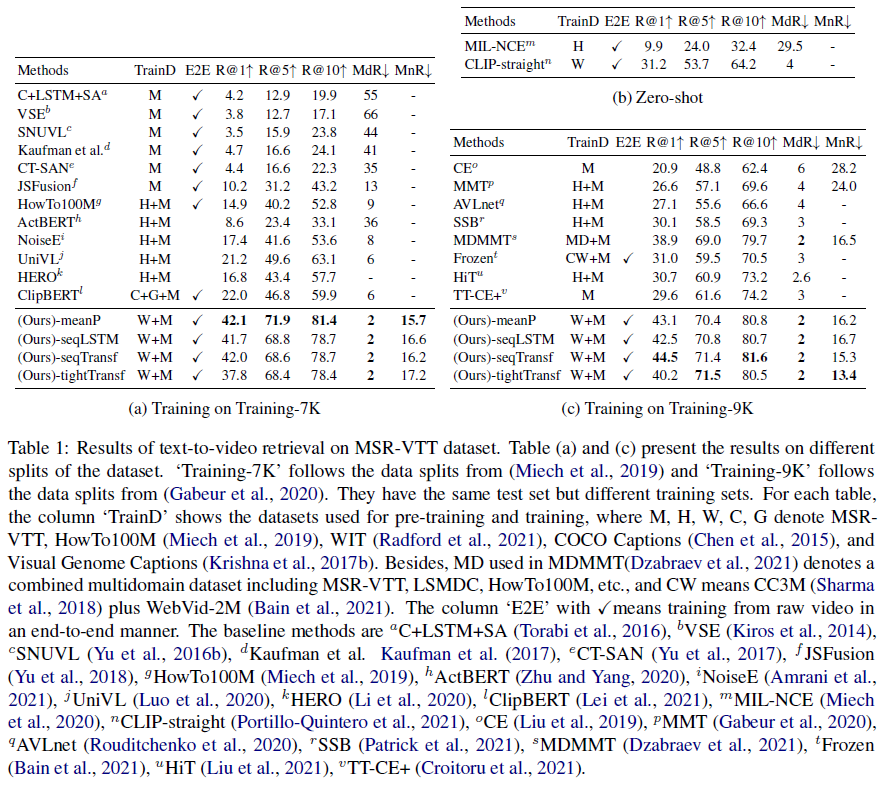
可以看到,训练数据比较少的时候,Parameter-free type 的效果最好,只有当增加训练数据时,其他方法的优势才能体现出来。这说明当我们的下游数据集比较小的时候,最好不要去微调 CLIP,直接用的效果往往是最好的
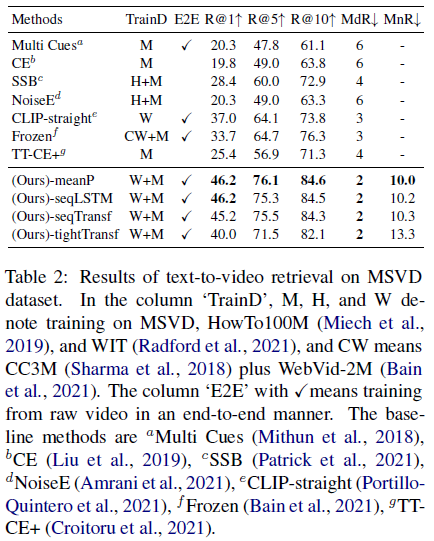 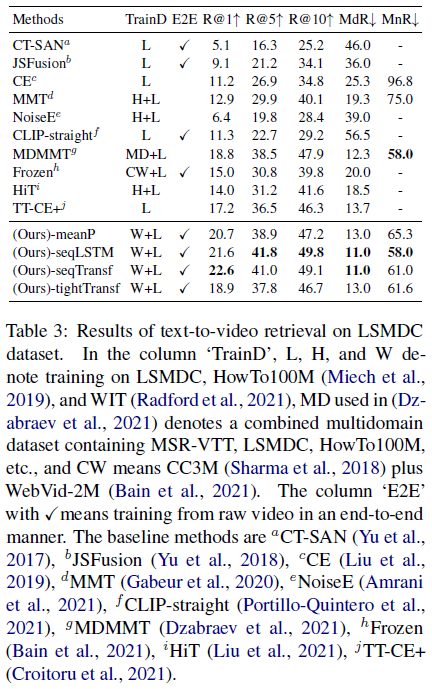 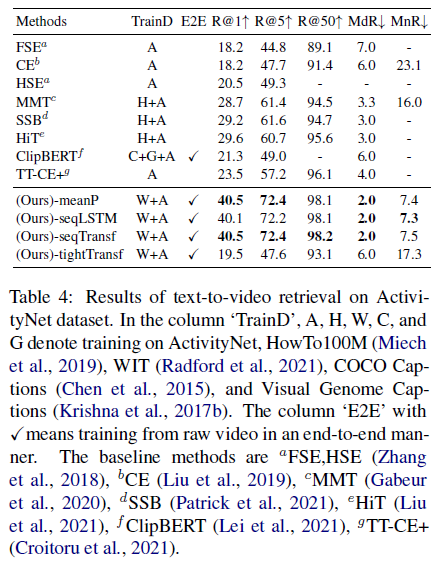 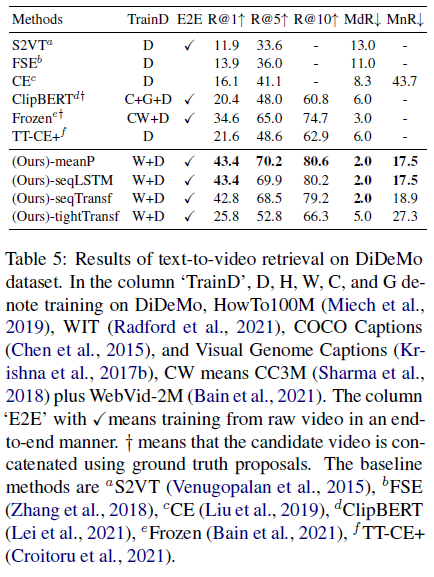
Actionclip: A new paradigm for video action recognition
Traditional unimodal framework
- 传统的视频动作分类方法将其视为一个单标签分类问题,将动作标签标记为序号。这种做法在标记时不太方便,例如 “open” 可以描述 “开门”、“打开一本书” 等很多动作,也就是说同一个动作对应的标签空间是接近无穷的,标记的类别太多不仅标注成本高,而且类别数太多时分类效果也会变差,而标记类别太少又会影响模型的泛化能力
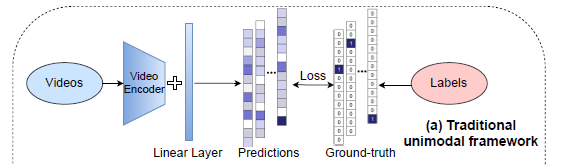
Multimodal Learning Framework
- 作者认为,one-hot 分类的方法完全忽略了动作标签文本具有的语义信息,因此作者引入了 CLIP 去解决 video-text multimodal learning problem.
视频到标签和标签到视频的相似性得分为 (作者这里计算的相似度是先取 1 个 batch 内的
N
N
N 个视频-标签对,然后计算所有视频和所有标签之间的相似度,而非
N
N
N 个视频和所有标签的相似度,训练方式与 CLIP 类似): 其中
x
x
x 为 video,
y
y
y 为 label. 上图的 ground-truth 标签矩阵中,行可以看作是视频,列可以看作是标签,对角线上的视频-标签对为匹配的正样本,同时由于 1 个 batch 中可能有多个视频对应同一个标签,因此非对角线区域也存在正样本.
p
x
2
y
(
x
)
p^{x2y}(x)
px2y(x) 和
p
y
2
x
(
y
)
p^{y2x}(y)
py2x(y) 就可以看作是两个概率分布。同时我们可以计算出 ground-truth similarity scores
q
x
2
y
(
x
)
q^{x2y}(x)
qx2y(x) 和
q
y
2
x
(
y
)
q^{y2x}(y)
qy2x(y),其中如果标签和视频匹配就标记为 1,不匹配就标记为 0,可以计算 KL 散度作为损失函数: (注意这里 KL 散度函数内,
p
p
p 和
q
q
q 的位置应该是写反了,否则当
q
=
0
q=0
q=0 而
p
>
0
p>0
p>0 时计算出的 KL 散度将无穷大)
The New Paradigm
- Main Architecture:作者采用 “pre-train, prompt and fine-tune” 范式。对于 pre-train 而言,作者直接使用 CLIP 作为预训练模型;下面主要介绍 prompt. prompt 的含义为 adjusting and reformulating the downstream tasks to act more like the upstream pre-training tasks,意思就是使得下游任务与预训练任务更相近。为此,作者采用了 textual prompt 和 visual prompt (post/in/pre-network prompt),其中对于 visual prompt 而言,如果预训练模型是在视频-文本对上训练的,那么我们就不需要进行 visual prompt,但 CLIP 是在图像-文本对上训练的,因此我们需要进行 visual prompt 来使得模型学得视频的时序信息
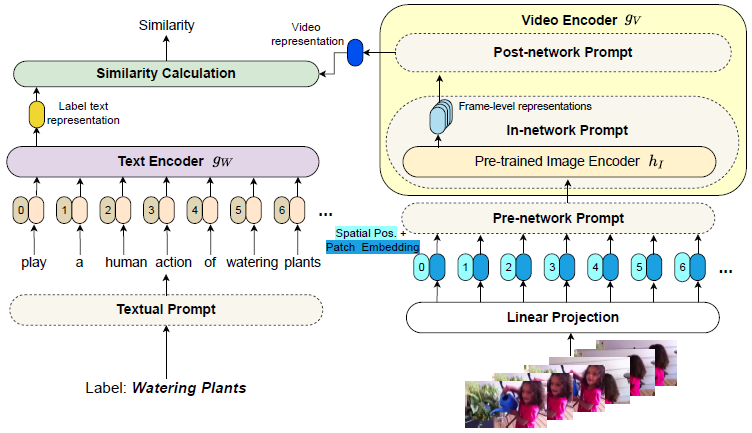
Note that in the new paradigm, the pre-trained model should not be largely modified due to catastrophic forgetting, where the pre-trained model loses its ability to do things that it was able to do in the pre-training.
- Textual Prompt Examples
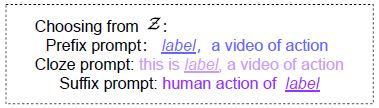 - Pre-network Prompt: Joint. 给定视频
x
x
x,Pre-network Prompt 将视频的所有帧都分别输入 visual encoder 来得到 frame-level representations. 另外,除了 spatial positional embedding,Pre-network Prompt 还加入了 temporal positional embedding 来表示视频帧的序号
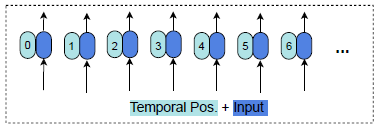 - In-network Prompt: Shift. 作者在每个 ViT layer 中间都加入了 temporal shift module (TSM) (which shifts part of the feature channels along the temporal dimension) (可参考 Ji Lin, Chuang Gan, and Song Han. Tsm: Temporal shift module for efficient video understanding. In Proceedings of the IEEE/CVF International Conference on Computer Vision, pages 7083–7093, 2019.),该模块没有额外参数并且能促进相邻视频帧之间的信息交换
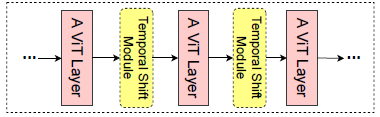 - Post-network Prompt:将多个单帧的特征表示 (frame-level representations) 融合为视频的特征表示 (video-level representations) (1) MeanP
(2) Conv1D/LSTM (3) Transf 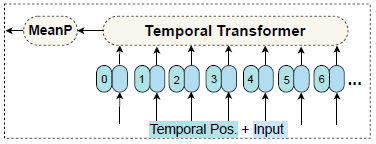
Experiments
- Is the “multimodal framework” helpful?
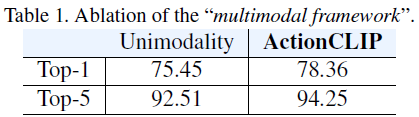 - Is the “pre-train” step important?
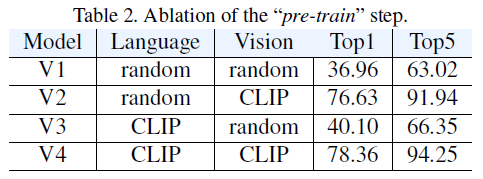 - Is the “prompt” step important?
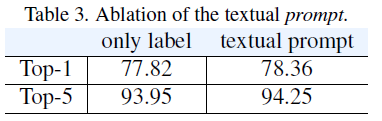 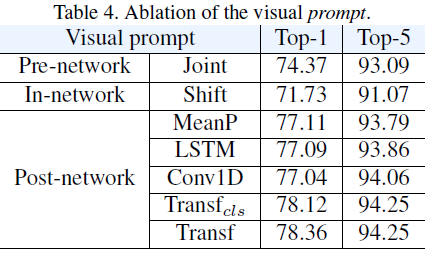 - Zero-shot/few-shot results
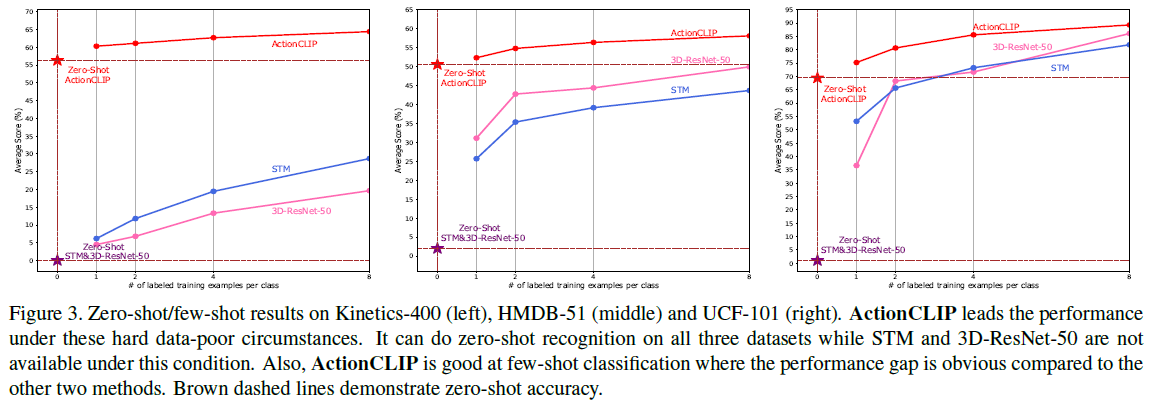
视觉-语言理解下游任务
How Much Can CLIP Benefit Vision-and-Language Tasks? (CLIP-ViL)
- To the best of our knowledge, we present the first large-scale empirical study on using CLIP as the visual encoder for diverse V&L tasks.
语音
Audioclip: Extending clip to image, text and audio
- 通过视频数据集,在图像和文本基础上增加了音频模态
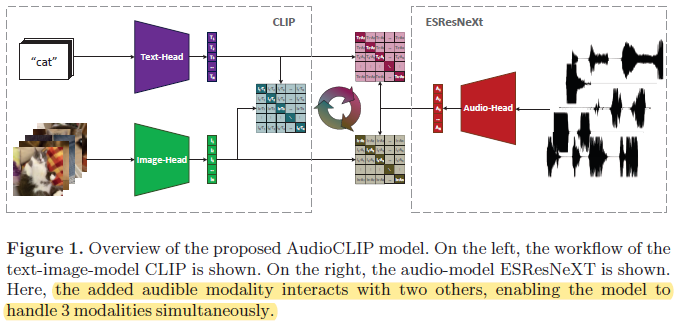
3D
Pointclip: Point cloud understanding by clip
- 通过将 3D 点云投影为 2D multi-view depth maps 来使用 CLIP
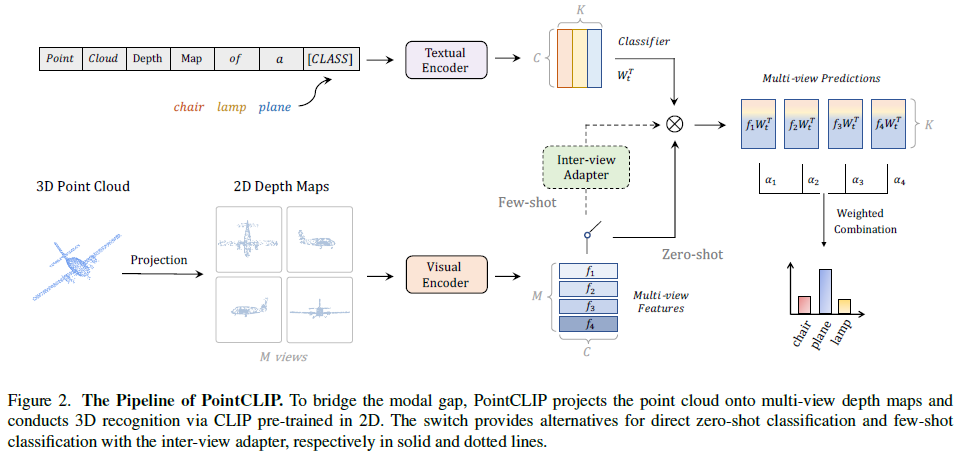
Can Language Understand Depth?
- 作者简化了深度估计问题,把它看成一个分类问题而非回归问题,然后使用 CLIP 进行深度估计
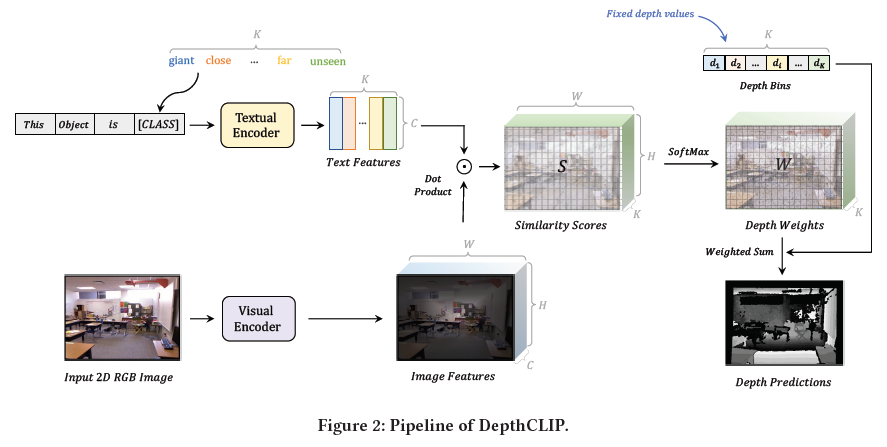
References
|