2012年,AlexNet横空出世。这个模型的名字来源于论文第一作者的姓名Alex Krizhevsky 。AlexNet使用了8层卷积神经网络,并以很大的优势赢得了ImageNet 2012图像识别挑战赛。它首次证明了学习到的特征可以超越手工设计的特征,从而一举打破计算机视觉研究的前状。
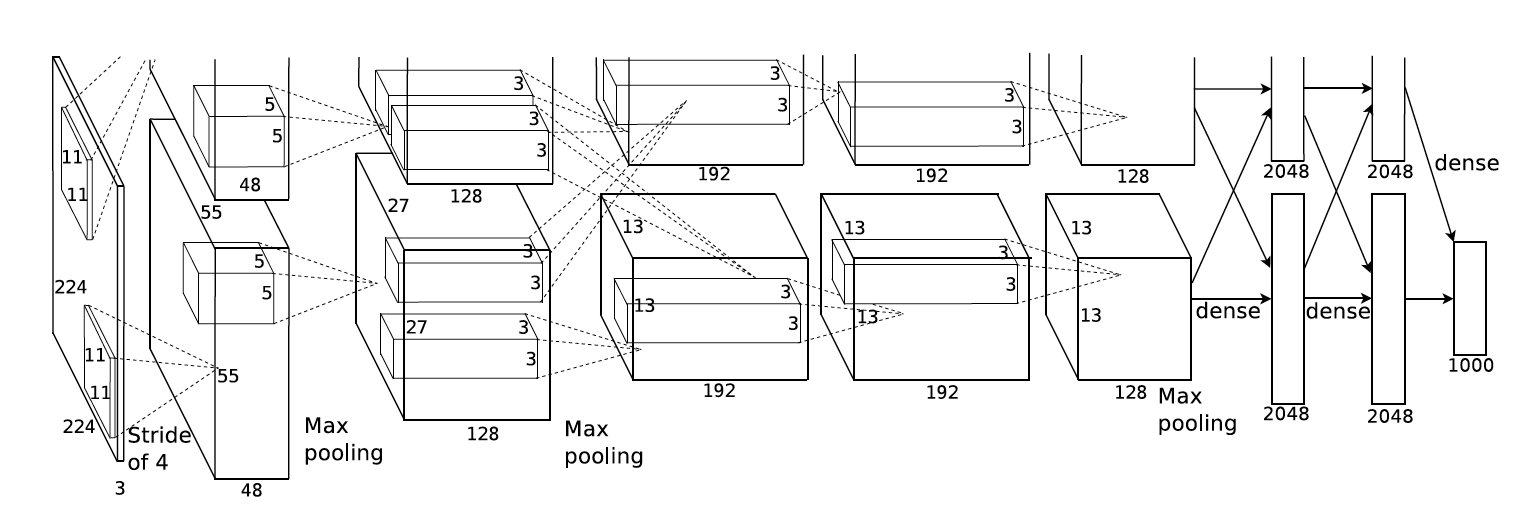
import torch
import torchvision
from torch import nn
from torchsummary import summary
print(torch.__version__)
?1.12.1+cpu
class AlexNet(nn.Module):
def __init__(self):
super(AlexNet, self).__init__()
self.conv = nn.Sequential(
nn.Conv2d(3, 96, 11, 4), # in_channels, out_channels, kernel_size, stride, padding
nn.ReLU(),
nn.MaxPool2d(3, 2), # kernel_size, stride
# 减小卷积窗口,使用填充为2来使得输入与输出的高和宽一致,且增大输出通道数
nn.Conv2d(96, 256, 5, 1, 2),
nn.ReLU(),
nn.MaxPool2d(3, 2),
# 连续3个卷积层,且使用更小的卷积窗口。除了最后的卷积层外,进一步增大了输出通道数。
# 前两个卷积层后不使用池化层来减小输入的高和宽
nn.Conv2d(256, 384, 3, 1, 1),
nn.ReLU(),
nn.Conv2d(384, 384, 3, 1, 1),
nn.ReLU(),
nn.Conv2d(384, 256, 3, 1, 1),
nn.ReLU(),
nn.MaxPool2d(3, 2)
)
# 这里全连接层的输出个数比LeNet中的大数倍。使用丢弃层来缓解过拟合
self.fc = nn.Sequential(
nn.Linear(256*5*5, 4096),
nn.ReLU(),
nn.Dropout(0.5),
nn.Linear(4096, 4096),
nn.ReLU(),
nn.Dropout(0.5),
nn.Linear(4096, 1000),
)
def forward(self, img):
feature = self.conv(img)
output = self.fc(feature.view(img.shape[0], -1))
return output
model = AlexNet()
print(model)
AlexNet(
(conv): Sequential(
(0): Conv2d(3, 96, kernel_size=(11, 11), stride=(4, 4))
(1): ReLU()
(2): MaxPool2d(kernel_size=3, stride=2, padding=0, dilation=1, ceil_mode=False)
(3): Conv2d(96, 256, kernel_size=(5, 5), stride=(1, 1), padding=(2, 2))
(4): ReLU()
(5): MaxPool2d(kernel_size=3, stride=2, padding=0, dilation=1, ceil_mode=False)
(6): Conv2d(256, 384, kernel_size=(3, 3), stride=(1, 1), padding=(1, 1))
(7): ReLU()
(8): Conv2d(384, 384, kernel_size=(3, 3), stride=(1, 1), padding=(1, 1))
(9): ReLU()
(10): Conv2d(384, 256, kernel_size=(3, 3), stride=(1, 1), padding=(1, 1))
(11): ReLU()
(12): MaxPool2d(kernel_size=3, stride=2, padding=0, dilation=1, ceil_mode=False)
)
(fc): Sequential(
(0): Linear(in_features=6400, out_features=4096, bias=True)
(1): ReLU()
(2): Dropout(p=0.5, inplace=False)
(3): Linear(in_features=4096, out_features=4096, bias=True)
(4): ReLU()
(5): Dropout(p=0.5, inplace=False)
(6): Linear(in_features=4096, out_features=1000, bias=True)
)
)
summary(model, (3, 224, 224))
?
----------------------------------------------------------------
Layer (type) Output Shape Param #
================================================================
Conv2d-1 [-1, 96, 54, 54] 34,944
ReLU-2 [-1, 96, 54, 54] 0
MaxPool2d-3 [-1, 96, 26, 26] 0
Conv2d-4 [-1, 256, 26, 26] 614,656
ReLU-5 [-1, 256, 26, 26] 0
MaxPool2d-6 [-1, 256, 12, 12] 0
Conv2d-7 [-1, 384, 12, 12] 885,120
ReLU-8 [-1, 384, 12, 12] 0
Conv2d-9 [-1, 384, 12, 12] 1,327,488
ReLU-10 [-1, 384, 12, 12] 0
Conv2d-11 [-1, 256, 12, 12] 884,992
ReLU-12 [-1, 256, 12, 12] 0
MaxPool2d-13 [-1, 256, 5, 5] 0
Linear-14 [-1, 4096] 26,218,496
ReLU-15 [-1, 4096] 0
Dropout-16 [-1, 4096] 0
Linear-17 [-1, 4096] 16,781,312
ReLU-18 [-1, 4096] 0
Dropout-19 [-1, 4096] 0
Linear-20 [-1, 1000] 4,097,000
================================================================
Total params: 50,844,008
Trainable params: 50,844,008
Non-trainable params: 0
----------------------------------------------------------------
Input size (MB): 0.57
Forward/backward pass size (MB): 10.18
Params size (MB): 193.95
Estimated Total Size (MB): 204.71
----------------------------------------------------------------
|