一、简介
针对伪装目标检测,本论文提出了Positioning and Focus Network (PFNet),该网络包含两个模块,定位(positioning module,PM)和聚焦(focus module,FM)。其中,定位模块从全局定位目标可能所在的位置,聚焦模块对区域进行识别并消除干扰来完善分割结果。
二、模型
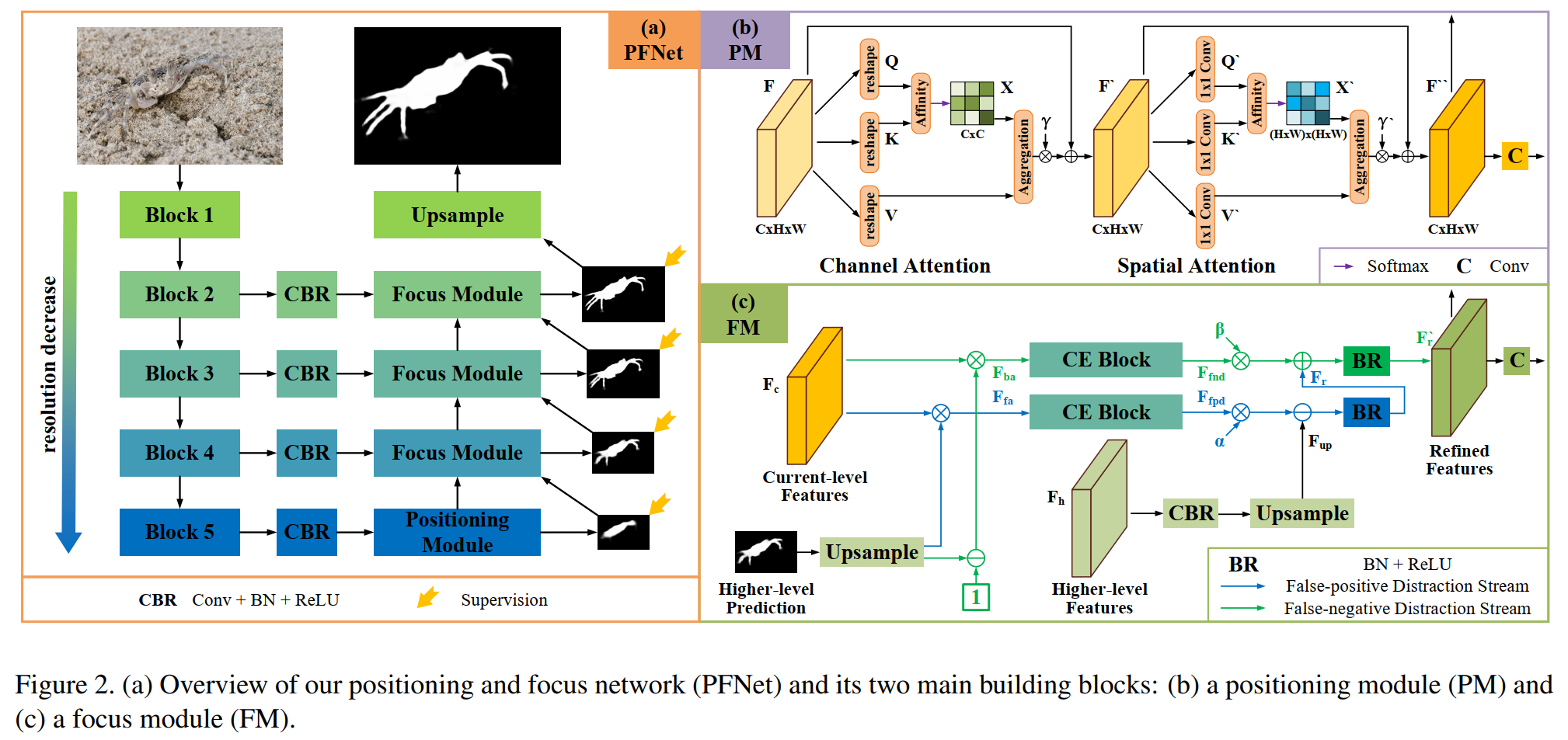 对于一张RGB图像,先用Resnet-50做backbone,提取出的多层特征,再送入四层卷积层减小通道数量;然后通过PM模块定位可能的目标位置;最后通过多个FM模块来发现和移除 false-positive 和 false-negative 的 distractions。
def __init__(self, backbone_path=None):
super(PFNet, self).__init__()
resnet50 = resnet.resnet50(backbone_path)
self.layer0 = nn.Sequential(resnet50.conv1, resnet50.bn1, resnet50.relu)
self.layer1 = nn.Sequential(resnet50.maxpool, resnet50.layer1)
self.layer2 = resnet50.layer2
self.layer3 = resnet50.layer3
self.layer4 = resnet50.layer4
self.cr4 = nn.Sequential(nn.Conv2d(2048, 512, 3, 1, 1), nn.BatchNorm2d(512), nn.ReLU())
self.cr3 = nn.Sequential(nn.Conv2d(1024, 256, 3, 1, 1), nn.BatchNorm2d(256), nn.ReLU())
self.cr2 = nn.Sequential(nn.Conv2d(512, 128, 3, 1, 1), nn.BatchNorm2d(128), nn.ReLU())
self.cr1 = nn.Sequential(nn.Conv2d(256, 64, 3, 1, 1), nn.BatchNorm2d(64), nn.ReLU())
self.positioning = Positioning(512)
self.focus3 = Focus(256, 512)
self.focus2 = Focus(128, 256)
self.focus1 = Focus(64, 128)
for m in self.modules():
if isinstance(m, nn.ReLU):
m.inplace = True
2.1 Positioning Module
PM包括一个 channel attention block 和一个 spatial attention block 。
channel attention block 中: 通过输入的 C x H x W 的张量 F 获得 k、q、v,在 C 上做attention,i 和 j 的关系是C上的关系,输出得到 F’,对于 F‘ 乘上一个可学习的超参数,并累加一个identify mapping。   spatial attention block 中: 首先对 F’ 用1 x 1的卷积改变其通道数来获得q’ 和 k’,v‘ 的通道数不变,在空间上做attention,i 和 j 的关系是位置 N=H x W上的关系,接下来的操作同 CA 类似。
2.2 Focus Module
FM的目的就是发现和消除 false-positive 和 false-negative 的错误预测。
具体步骤见如下代码及注释。
def forward(self, x, y, in_map):
up = self.up(y)
input_map = self.input_map(in_map)
f_feature = x * input_map
b_feature = x * (1 - input_map)
fp = self.fp(f_feature)
fn = self.fn(b_feature)
refine1 = up - (self.alpha * fp)
refine1 = self.bn1(refine1)
refine1 = self.relu1(refine1)
refine2 = refine1 + (self.beta * fn)
refine2 = self.bn2(refine2)
refine2 = self.relu2(refine2)
output_map = self.output_map(refine2)
return refine2, output_map
其中的Context Exploration Block如下所示。 DConv是膨胀卷积,目的是增加模型的感受野。 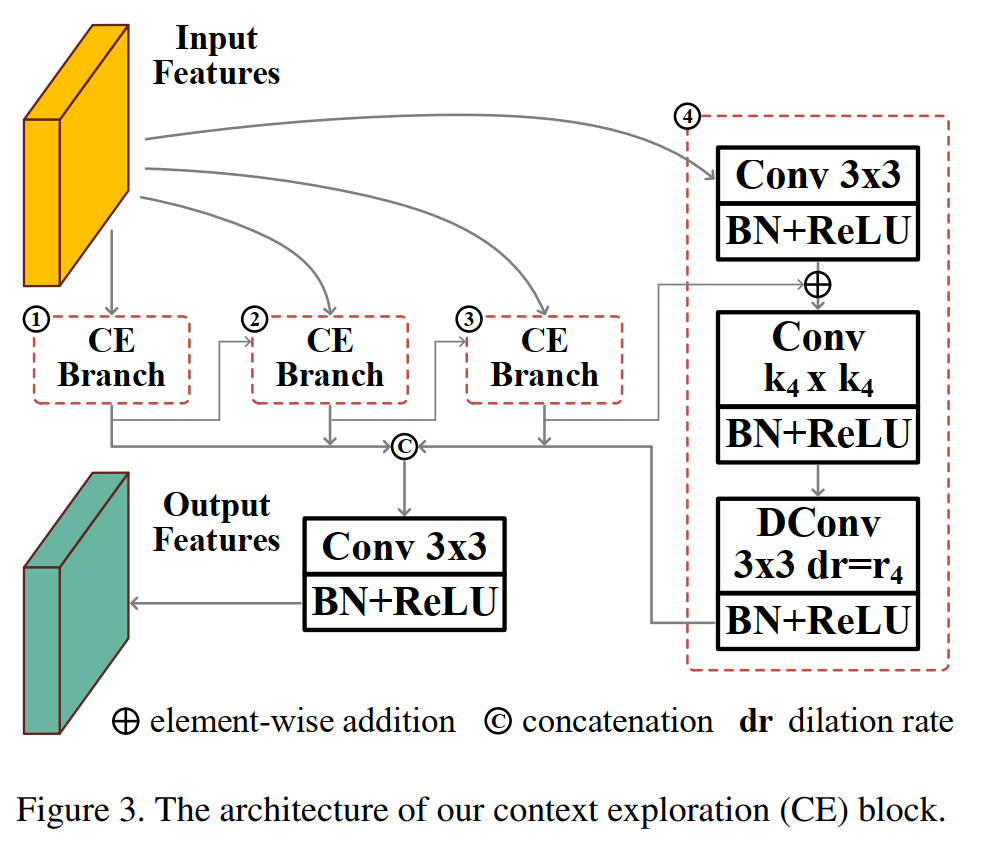 通过CE,模型能获得大尺度上感知上下文的特征的能力。
2.3 损失函数
在PFNet中有4个输出的预测值,一个是PM,另外的是FM,
对于PM模块,使用bce和iou损失。  对于FM模块,使用weighted bce和weighted iou损失。  最后的一个总损失即: 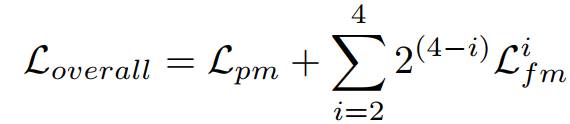
三、实验
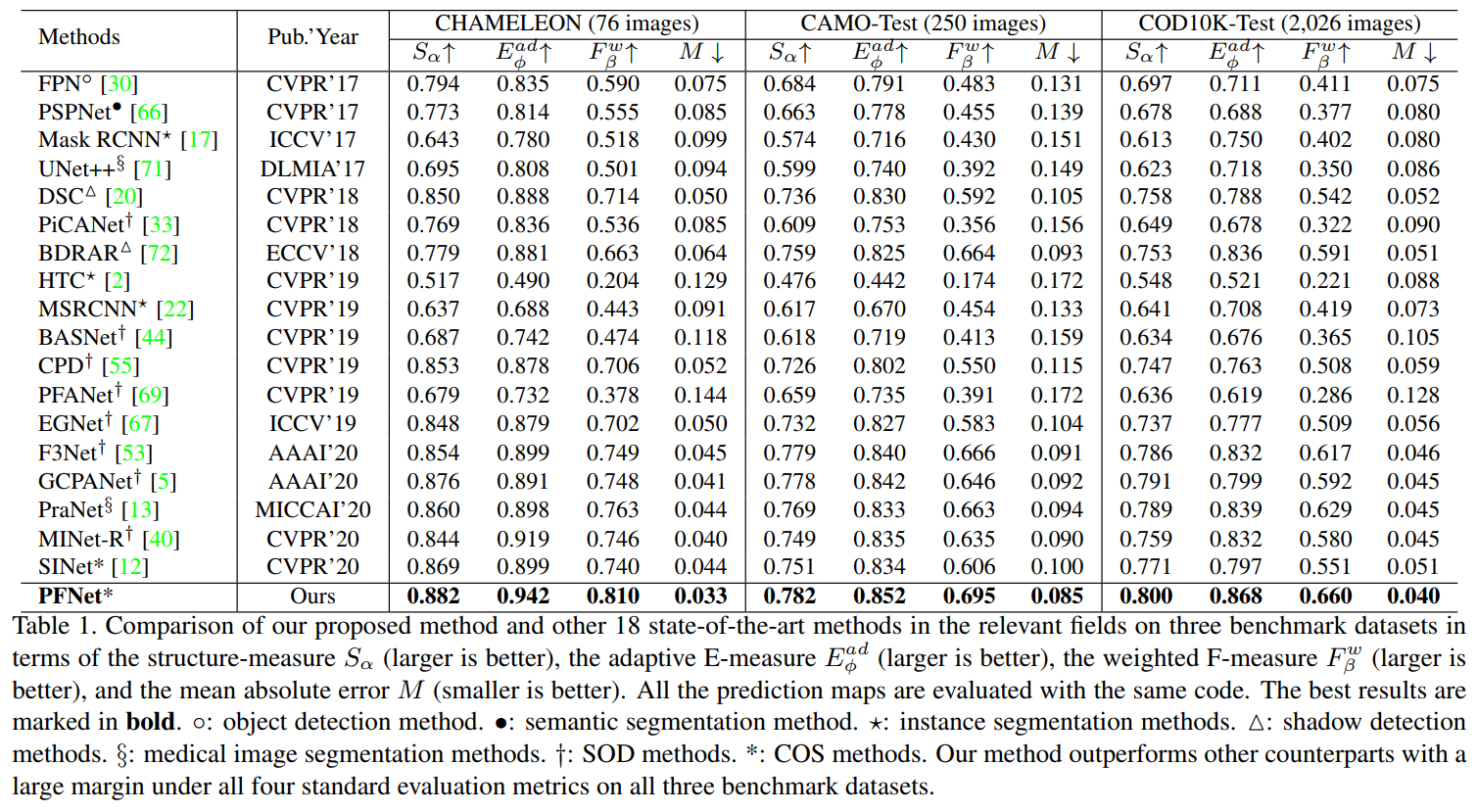 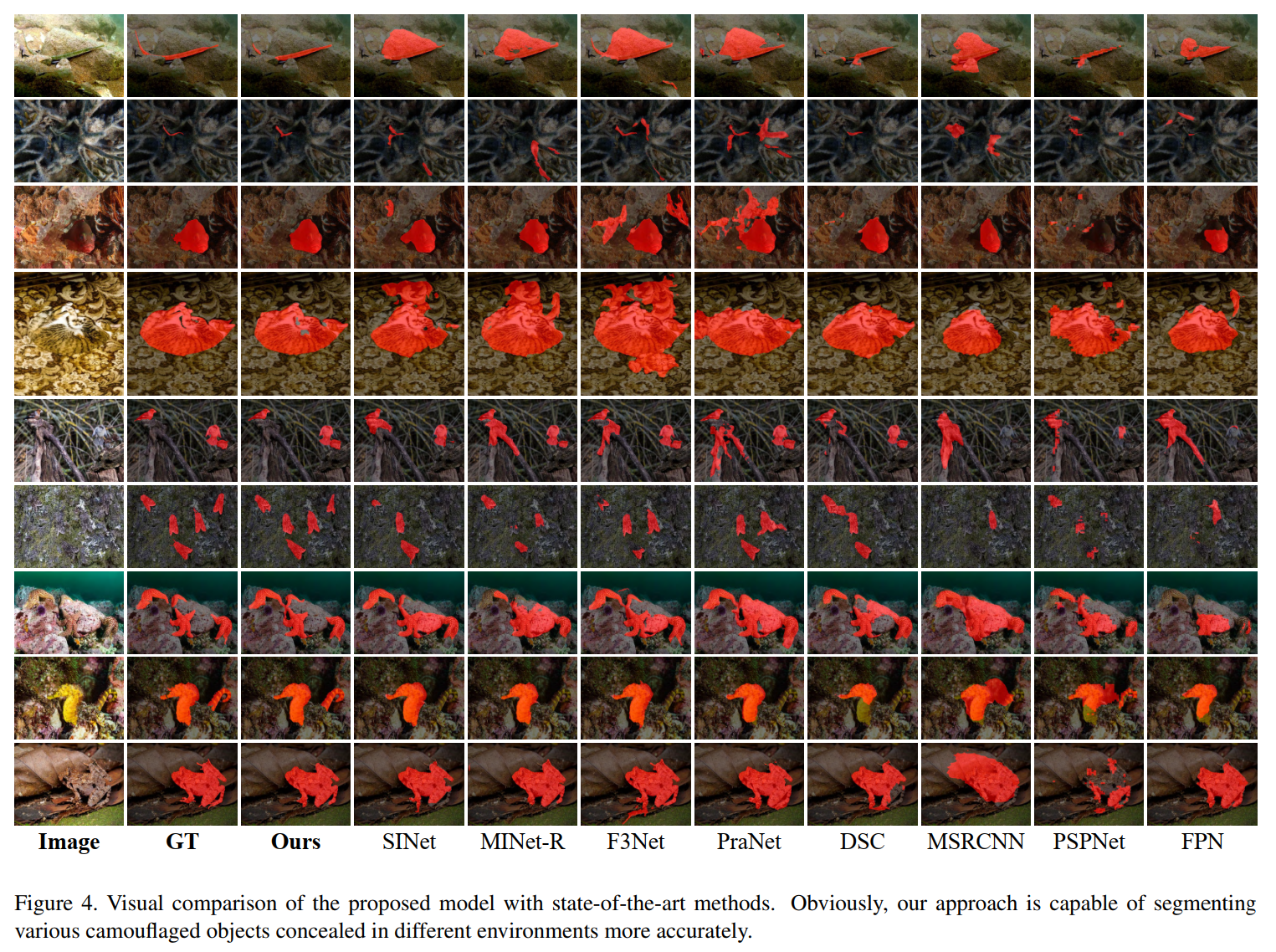 消融实验: 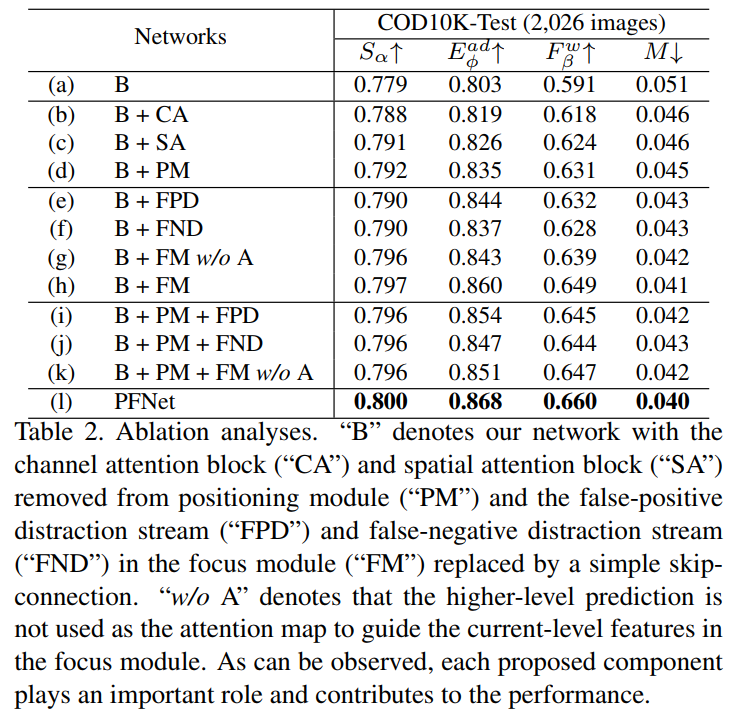
|