1. 自动驾驶实战:基于Paddle3D的点云障碍物检测
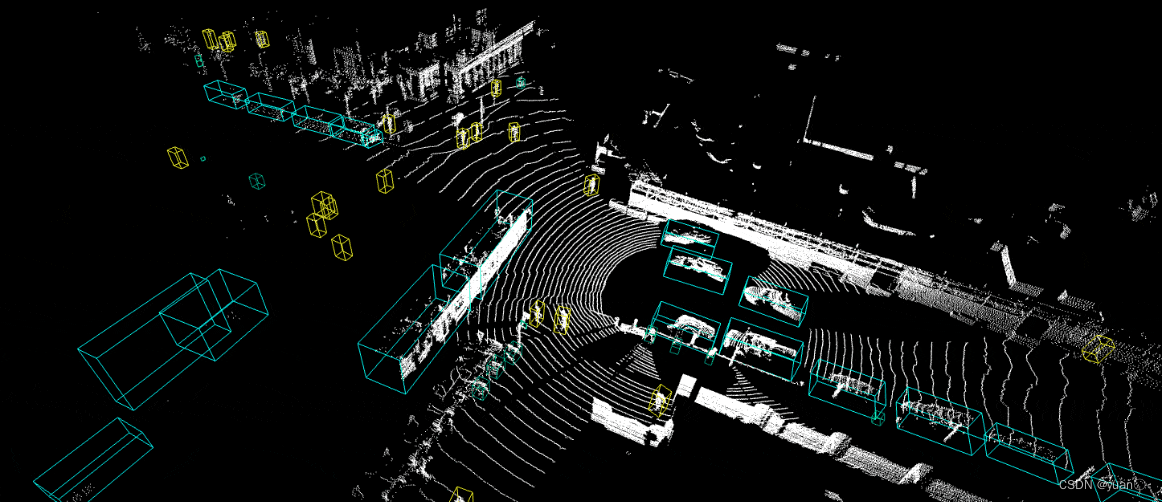
项目地址——自动驾驶实战:基于Paddle3D的点云障碍物检测 课程地址——自动驾驶感知系统揭秘
1.1 环境信息
硬件信息 CPU: 2核 AI加速卡: v100 总显存: 16GB 总内存: 16 GB 总硬盘:100 GB 环境配置 Python:3.7.4 框架信息 框架版本: PaddlePaddle 2.4.0(项目默认框架版本为2.3.2,但由于某些库更新了,原版本代码无法正常运行——2022.12.20)
1.2 准备点云数据
????Paddle3D支持按照KITTI数据集格式构建自己的数据集,可参考文档自定义数据集格式说明进行准备。
????为了能快速演示整个流程,本项目使用数量为300帧的KITTI小数据集。该小数据集是从从KITTI训练集中随机抽取了250帧点云、验证集中随机抽取50帧点云。完整的KITTI数据集可至官网下载。
解压小数据集:
!tar xvzf data/data165771/kitti300frame.tar.gz
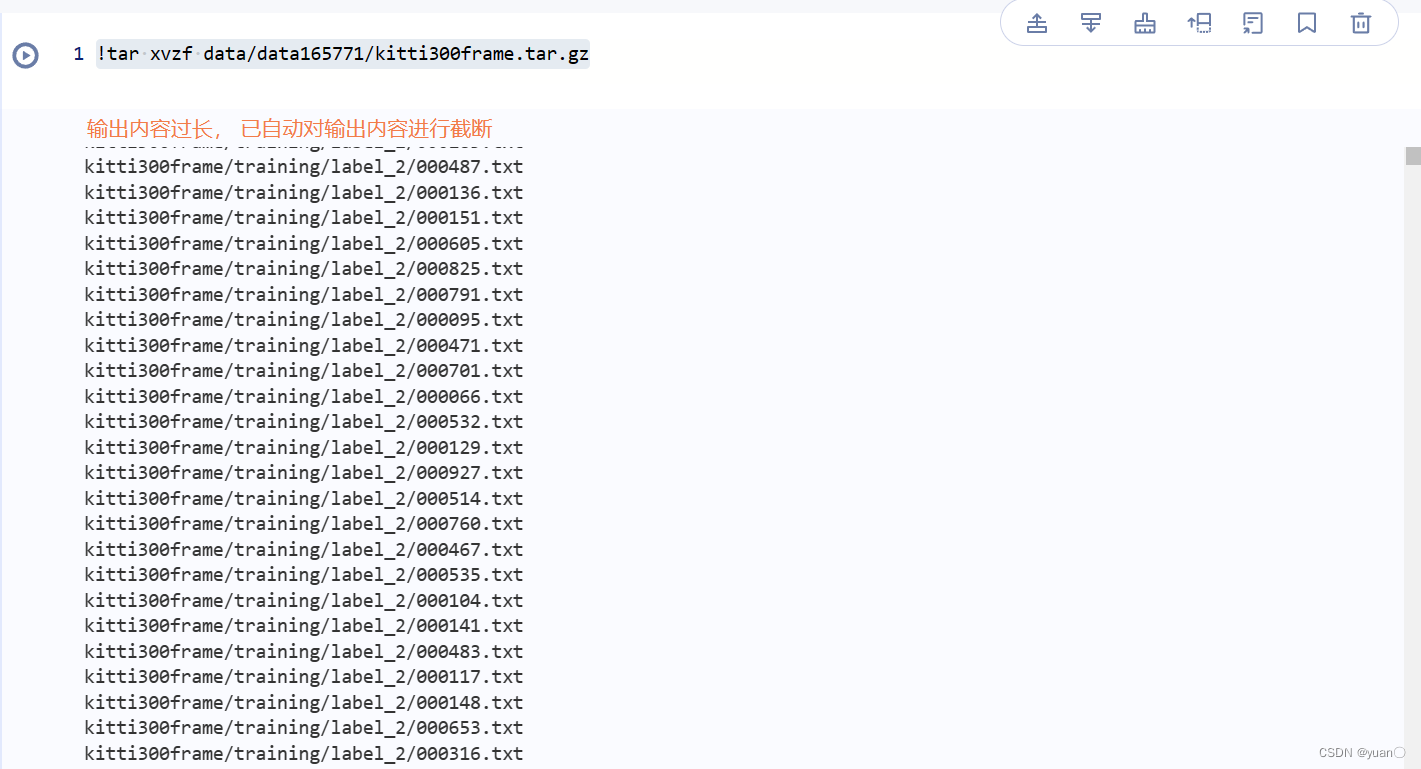
1.3 安装Paddle3D
????克隆Paddle3D源码,基于develop分支完成安装:
下载Paddle3D源码:
!git clone https://github.com/PaddlePaddle/Paddle3D
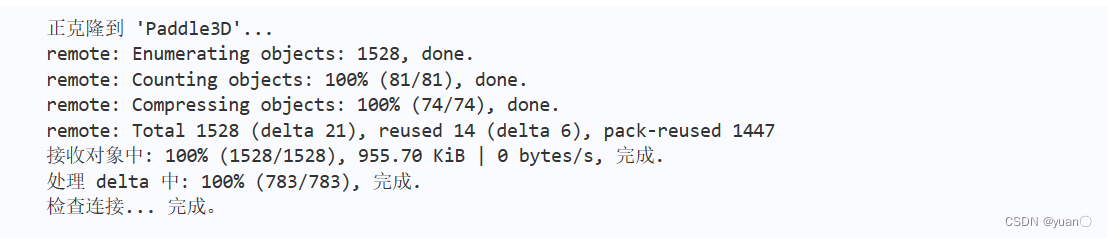 更新pip
!pip install --upgrade pip
进入Paddle3D所在路径:
cd /home/aistudio/Paddle3D
安装Paddle3D依赖项:
!python -m pip install -r requirements.txt
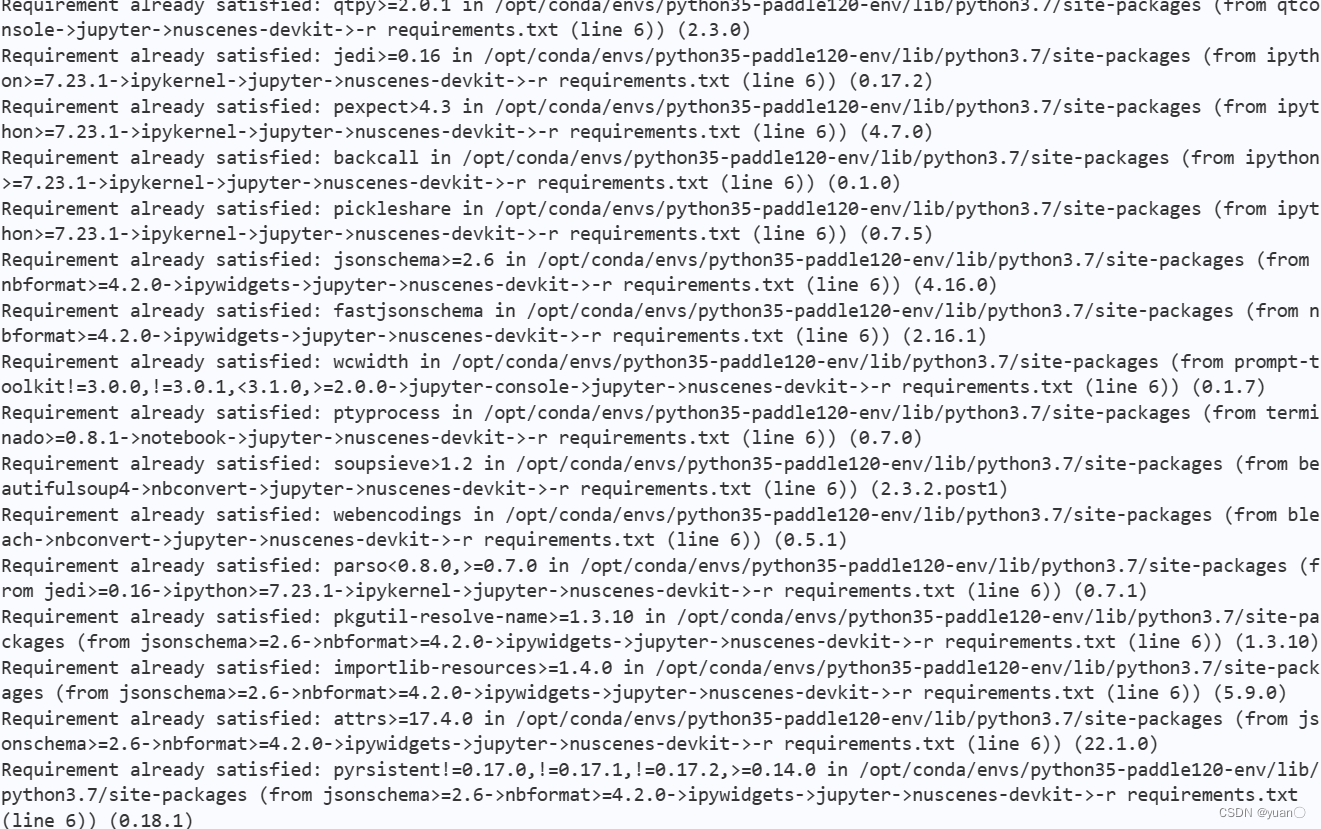 安装Paddle3D源码:
!python setup.py install
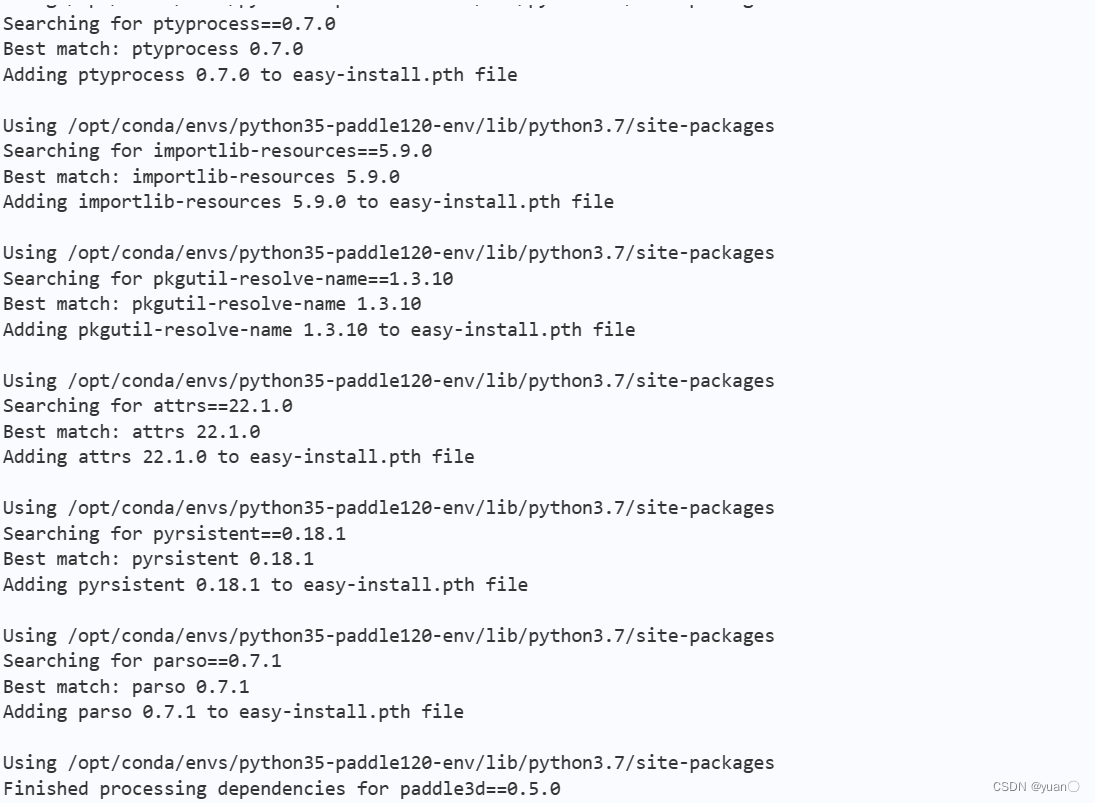
1.4 模型训练
????CenterPoint以点云作为输入,基于关键点检测的方式回归物体的尺寸、方向和速度。面向物体尺寸多样不一的场景时其精度表现更高,简易的模型设计使其在性能上也表现更加高效。
????目前Paddle3D对CenterPoint性能做了极致优化,因此本项目选择CenterPoint完成点云障碍物检测。
(1) 创建数据集软链
!mkdir datasets
!ln -s /home/aistudio/kitti300frame ./datasets
!mv ./datasets/kitti300frame ./datasets/KITTI
(2) 生成训练时数据增强所需的真值库
!python tools/create_det_gt_database.py --dataset_name kitti --dataset_root ./datasets/KITTI --save_dir ./datasets/KITTI
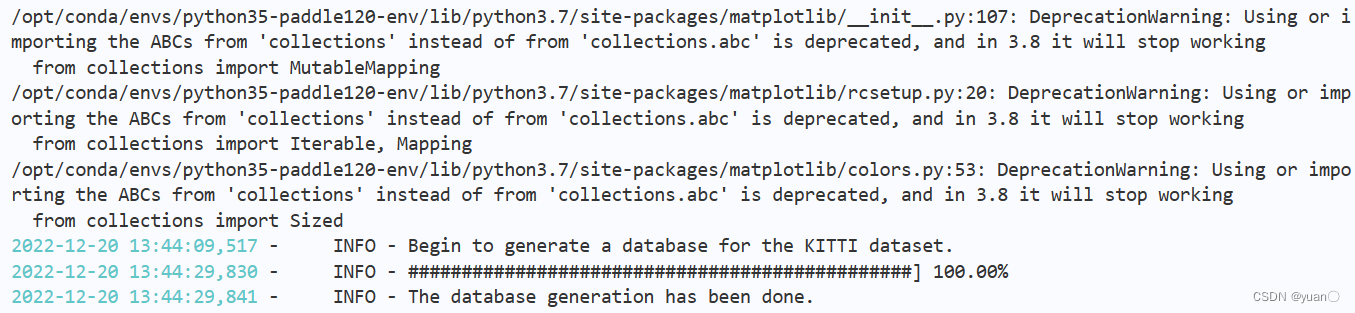 (3) 修改配置文件 ????Paddle3D中CenterPoint提供的KITTI baseline是基于8卡32G V100训练,此处只有1张16G V100显卡,所以需要将学习率和批大小修改成针对本地单卡的。注意:要修改两个文件。
!cp configs/centerpoint/centerpoint_pillars_016voxel_kitti.yml configs/centerpoint/centerpoint_pillars_016voxel_minikitti.yml
(4)启动训练
通过指定--model https://bj.bcebos.com/paddle3d/models/centerpoint//centerpoint_pillars_016voxel_kitti/model.pdparams 基于预训练模型进行Fintune: 训练时长约3小时(epoch = 160)。
!python tools/train.py --config configs/centerpoint/centerpoint_pillars_016voxel_minikitti.yml --save_dir ./output_kitti --num_workers 3 --save_interval 5 --model https://bj.bcebos.com/paddle3d/models/centerpoint//centerpoint_pillars_016voxel_kitti/model.pdparams
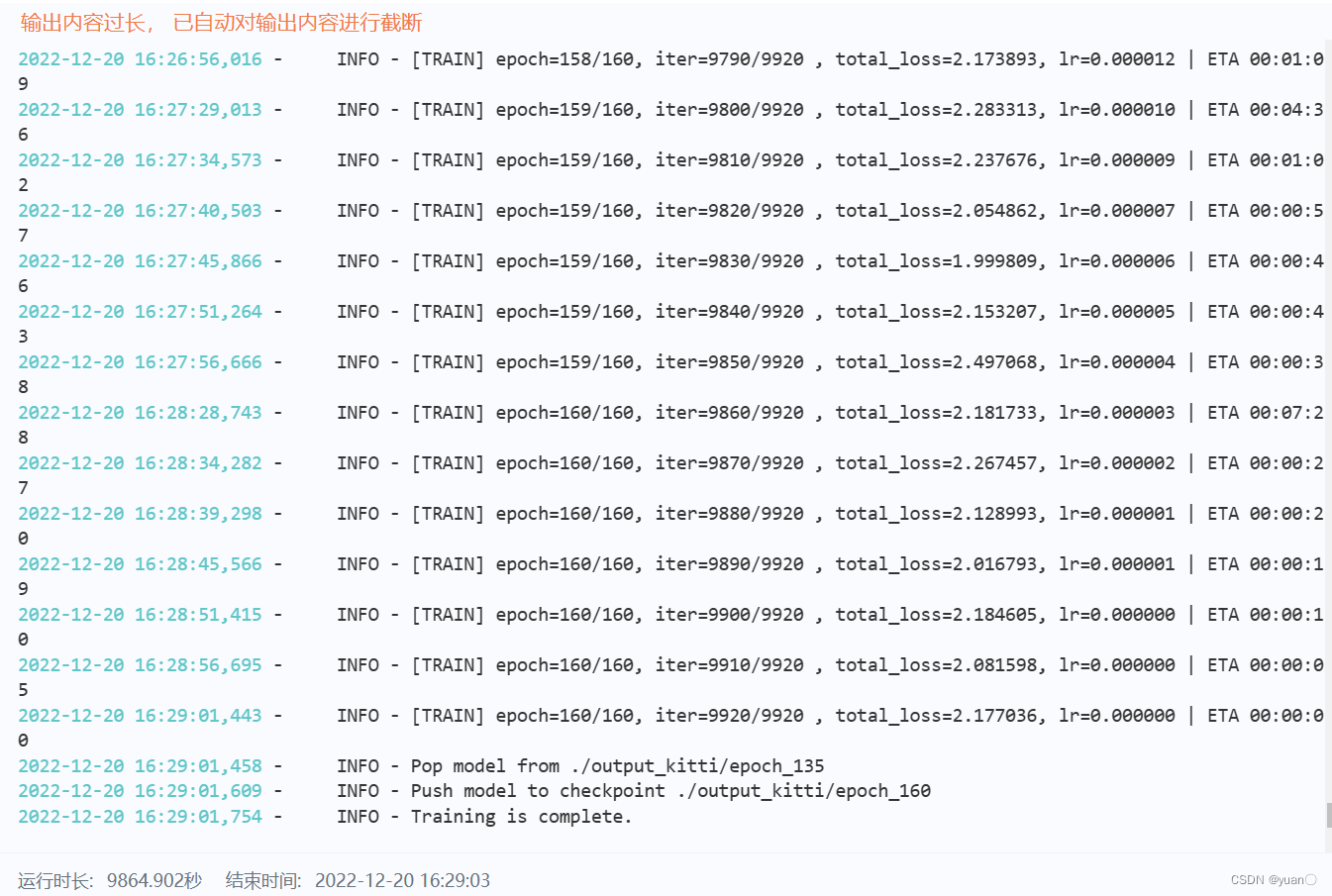
1.5 模型评估
????模型训练完成后,可以评估模型的精度:
!python tools/evaluate.py --config configs/centerpoint/centerpoint_pillars_016voxel_minikitti.yml --model https://bj.bcebos.com/paddle3d/models/centerpoint//centerpoint_pillars_016voxel_kitti/model.pdparams --batch_size 1 --num_workers 3
????可以看到,模型对于车的识别结果较好,对于行人、自行车的识别效果较差。
1.6 模型导出
epoch = 160
!python tools/export.py --config configs/centerpoint/centerpoint_pillars_016voxel_minikitti.yml --model ./output_kitti/epoch_160/model.pdparams --save_dir ./output_kitti_inference
epoch = 20
!python tools/export.py --config configs/centerpoint/centerpoint_pillars_016voxel_minikitti.yml --model ./output_kitti/epoch_20/model.pdparams --save_dir ./output_kitti_inference
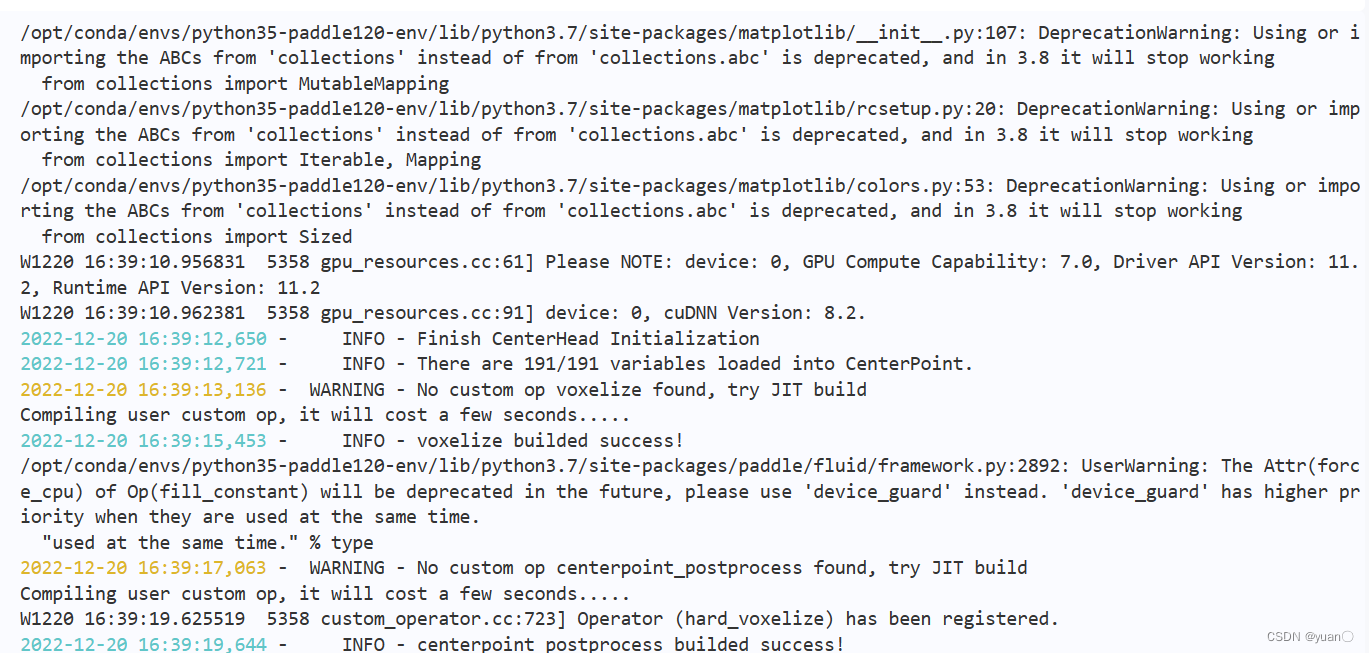
1.7 模型部署
????CenterPoint支持使用C++和Python语言部署,C++部署方式可以参考Paddle3D CenterPoint C++部署文档。本项目采用基于Paddle Inference推理引擎,使用Python语言进行部署。
进入python部署代码所在目录:
cd deploy/centerpoint/python
指定模型文件所在路径、待预测点云文件所在路径,执行预测:
!python infer.py --model_file /home/aistudio/Paddle3D/output_kitti_inference/centerpoint.pdmodel --params_file /home/aistudio/Paddle3D/output_kitti_inference/centerpoint.pdiparams --lidar_file /home/aistudio/Paddle3D/datasets/KITTI/training/velodyne/000104.bin --num_point_dim 4
预测数据,保存为pred.txt文件
Score: 0.8801702857017517 Label: 0 Box(x_c, y_c, z_c, w, l, h, -rot): 15.949016571044922 3.401707649230957 -0.838792085647583 1.6645100116729736 4.323172092437744 1.5860841274261475 1.9184210300445557
Score: 0.8430783748626709 Label: 0 Box(x_c, y_c, z_c, w, l, h, -rot): 4.356827735900879 7.624661922454834 -0.7098685503005981 1.625045657157898 3.905561685562134 1.6247363090515137 1.9725804328918457
Score: 0.8185914158821106 Label: 0 Box(x_c, y_c, z_c, w, l, h, -rot): 37.213130950927734 -4.919034481048584 -1.0293656587600708 1.6997096538543701 3.982091188430786 1.504879355430603 1.9469859600067139
Score: 0.7745243906974792 Label: 0 Box(x_c, y_c, z_c, w, l, h, -rot): 23.25118637084961 -7.758514404296875 -1.1013673543930054 1.665223240852356 4.344735145568848 1.5877608060836792 -1.17690110206604
Score: 0.7038797736167908 Label: 0 Box(x_c, y_c, z_c, w, l, h, -rot): 28.963340759277344 -1.4896349906921387 -0.902091383934021 1.7479376792907715 4.617205619812012 1.563733458518982 1.8184967041015625
Score: 0.17345771193504333 Label: 0 Box(x_c, y_c, z_c, w, l, h, -rot): 43.669837951660156 -14.53625774383545 -0.8703321218490601 1.6278570890426636 3.9948198795318604 1.514023780822754 0.8482679128646851
Score: 0.13678330183029175 Label: 0 Box(x_c, y_c, z_c, w, l, h, -rot): -0.004944105166941881 -0.15849240124225616 -1.0278022289276123 1.6501120328903198 3.8041915893554688 1.5048600435256958 -1.235048770904541
Score: 0.1301172822713852 Label: 0 Box(x_c, y_c, z_c, w, l, h, -rot): 57.76295471191406 3.359720468521118 -0.6944454908370972 1.5720959901809692 3.5915873050689697 1.4879614114761353 0.9269833564758301
Score: 0.11003818362951279 Label: 0 Box(x_c, y_c, z_c, w, l, h, -rot): 31.166778564453125 4.625081539154053 -1.1666207313537598 1.5735297203063965 3.7136919498443604 1.4804550409317017 1.9523953199386597
Score: 0.1025351956486702 Label: 0 Box(x_c, y_c, z_c, w, l, h, -rot): 25.748493194580078 17.13875389099121 -1.334594964981079 1.497322916984558 3.33937406539917 1.375497579574585 1.2643632888793945
Score: 0.10173971205949783 Label: 0 Box(x_c, y_c, z_c, w, l, h, -rot): 40.83097457885742 -14.601551055908203 -0.8294260501861572 1.5711668729782104 4.121697902679443 1.4682270288467407 1.7342313528060913
Score: 0.10121338814496994 Label: 0 Box(x_c, y_c, z_c, w, l, h, -rot): 58.73474884033203 -13.881253242492676 -0.9767248630523682 1.7061117887496948 4.040306091308594 1.5399994850158691 2.1176371574401855
Score: 0.14155955612659454 Label: 1 Box(x_c, y_c, z_c, w, l, h, -rot): 25.353525161743164 -15.547738075256348 -0.19825123250484467 0.6376267075538635 1.7945102453231812 1.7785474061965942 2.360872507095337
Score: 0.12169421464204788 Label: 1 Box(x_c, y_c, z_c, w, l, h, -rot): 29.0213565826416 18.047924041748047 -0.8930382132530212 0.39424917101860046 1.6417008638381958 1.6697943210601807 -1.8017770051956177
????对预测结果进行可视化,更为直观地展示预测效果。我们将预测结果保存至文件pred.txt中,便于可视化脚本加载。通过指定–draw_threshold可以过滤掉低分预测框:
!python /home/aistudio/show_lidar_pred_on_image.py --calib_file /home/aistudio/Paddle3D/datasets/KITTI/training/calib/000104.txt --image_file /home/aistudio/Paddle3D/datasets/KITTI/training/image_2/000104.png --label_file /home/aistudio/Paddle3D/datasets/KITTI/training/label_2/000104.txt --pred_file /home/aistudio/pred.txt --save_dir ./ --draw_threshold 0.16
效果
图片保存的路径 /home/aistudio/Paddle3D/deploy/centerpoint/python/000104.png 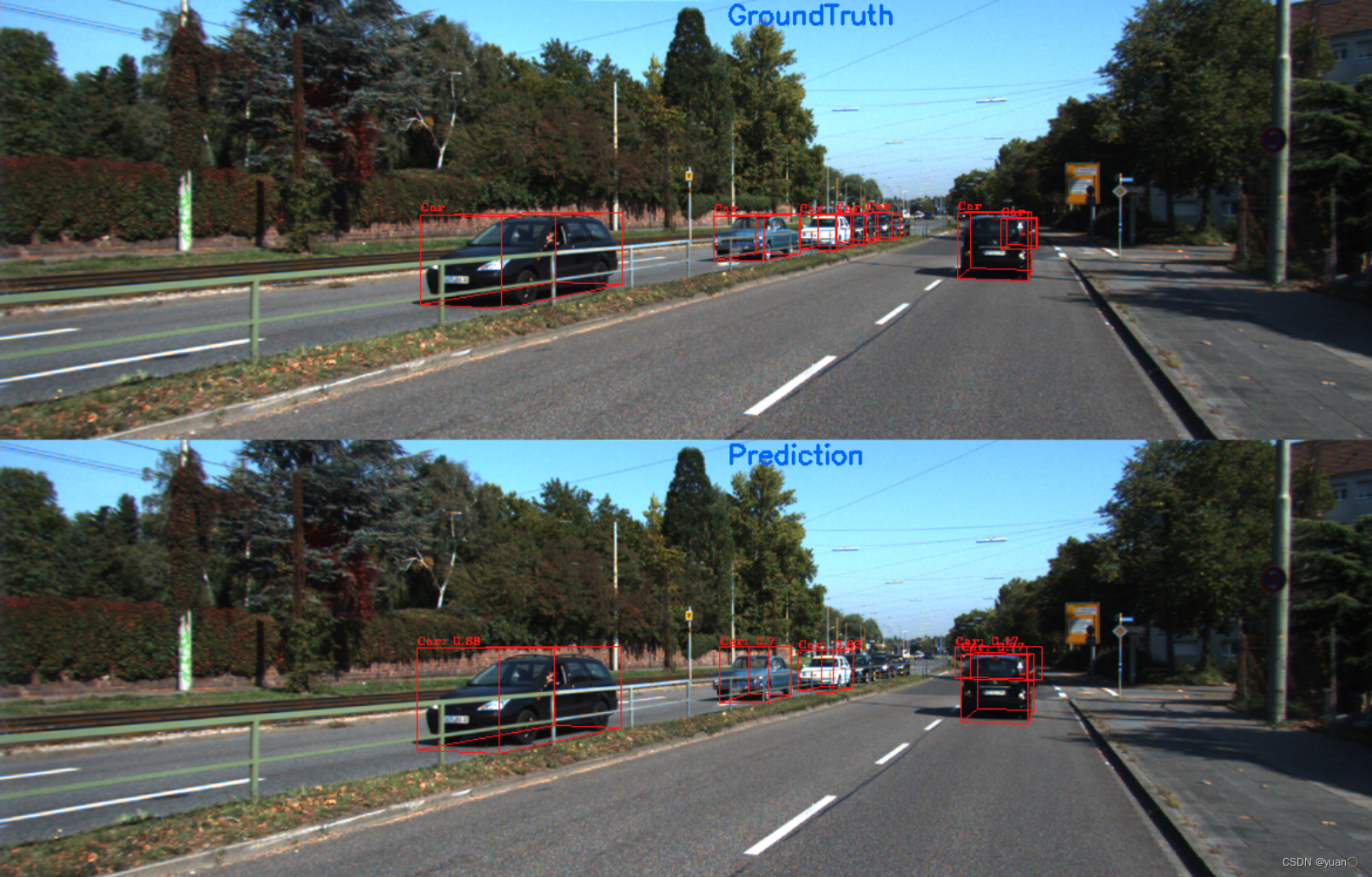
三维点云的投影
附录
show_lidar_pred_on_image.py
import argparse
import os
import os.path as osp
import cv2
import numpy as np
from paddle3d.datasets.kitti.kitti_utils import box_lidar_to_camera
from paddle3d.geometries import BBoxes3D, CoordMode
from paddle3d.sample import Sample
classmap = {0: 'Car', 1: 'Cyclist', 2: 'Pedestrain'}
def parse_args():
parser = argparse.ArgumentParser()
parser.add_argument(
'--calib_file', dest='calib_file', help='calibration file', type=str)
parser.add_argument(
'--image_file', dest='image_file', help='image file', type=str)
parser.add_argument(
'--label_file', dest='label_file', help='label file', type=str)
parser.add_argument(
'--pred_file',
dest='pred_file',
help='prediction results file',
type=str)
parser.add_argument(
'--save_dir',
dest='save_dir',
help='the path to save visualized result',
type=str)
parser.add_argument(
'--draw_threshold',
dest='draw_threshold',
help=
'prediction whose confidence is lower than threshold would not been shown',
type=float)
return parser.parse_args()
class Calib:
def __init__(self, dict_calib):
super(Calib, self).__init__()
self.P0 = dict_calib['P0'].reshape(3, 4)
self.P1 = dict_calib['P1'].reshape(3, 4)
self.P2 = dict_calib['P2'].reshape(3, 4)
self.P3 = dict_calib['P3'].reshape(3, 4)
self.R0_rect = dict_calib['R0_rect'].reshape(3, 3)
self.Tr_velo_to_cam = dict_calib['Tr_velo_to_cam'].reshape(3, 4)
self.Tr_imu_to_velo = dict_calib['Tr_imu_to_velo'].reshape(3, 4)
class Object3d:
def __init__(self, content):
super(Object3d, self).__init__()
lines = content.split()
lines = list(filter(lambda x: len(x), lines))
self.name, self.truncated, self.occluded, self.alpha = lines[0], float(
lines[1]), float(lines[2]), float(lines[3])
self.bbox = [lines[4], lines[5], lines[6], lines[7]]
self.bbox = np.array([float(x) for x in self.bbox])
self.dimensions = [lines[8], lines[9], lines[10]]
self.dimensions = np.array([float(x) for x in self.dimensions])
self.location = [lines[11], lines[12], lines[13]]
self.location = np.array([float(x) for x in self.location])
self.rotation_y = float(lines[14])
if len(lines) == 16:
self.score = float(lines[15])
def rot_y(rotation_y):
cos = np.cos(rotation_y)
sin = np.sin(rotation_y)
R = np.array([[cos, 0, sin], [0, 1, 0], [-sin, 0, cos]])
return R
def parse_gt_info(calib_path, label_path):
with open(calib_path) as f:
lines = f.readlines()
lines = list(filter(lambda x: len(x) and x != '\n', lines))
dict_calib = {}
for line in lines:
key, value = line.split(":")
dict_calib[key] = np.array([float(x) for x in value.split()])
calib = Calib(dict_calib)
with open(label_path, 'r') as f:
lines = f.readlines()
lines = list(filter(lambda x: len(x) and x != '\n', lines))
obj = [Object3d(x) for x in lines]
return calib, obj
def predictions_to_kitti_format(pred):
num_boxes = pred.bboxes_3d.shape[0]
names = np.array([classmap[label] for label in pred.labels])
calibs = pred.calibs
if pred.bboxes_3d.coordmode != CoordMode.KittiCamera:
bboxes_3d = box_lidar_to_camera(pred.bboxes_3d, calibs)
else:
bboxes_3d = pred.bboxes_3d
if bboxes_3d.origin != [.5, 1., .5]:
bboxes_3d[:, :3] += bboxes_3d[:, 3:6] * (
np.array([.5, 1., .5]) - np.array(bboxes_3d.origin))
bboxes_3d.origin = [.5, 1., .5]
loc = bboxes_3d[:, :3]
dim = bboxes_3d[:, 3:6]
contents = []
for i in range(num_boxes):
content = "{} 0 0 0 0 0 0 0 {} {} {} {} {} {} {} {}".format(
names[i], dim[i, 2], dim[i, 1], dim[i, 0], loc[i, 0], loc[i, 1],
loc[i, 2], bboxes_3d[i, 6], pred.confidences[i])
contents.append(content)
obj = [Object3d(x) for x in contents]
return obj
def parse_pred_info(pred_path, calib):
with open(pred_path, 'r') as f:
lines = f.readlines()
lines = list(filter(lambda x: len(x) and x != '\n', lines))
scores = []
labels = []
boxes_3d = []
for res in lines:
score = float(res.split("Score: ")[-1].split(" ")[0])
label = int(res.split("Label: ")[-1].split(" ")[0])
box_3d = res.split("Box(x_c, y_c, z_c, w, l, h, -rot): ")[-1].split(" ")
box_3d = [float(b) for b in box_3d]
scores.append(score)
labels.append(label)
boxes_3d.append(box_3d)
scores = np.stack(scores)
labels = np.stack(labels)
boxes_3d = np.stack(boxes_3d)
data = Sample(pred_path, 'lidar')
data.bboxes_3d = BBoxes3D(boxes_3d)
data.bboxes_3d.coordmode = 'Lidar'
data.bboxes_3d.origin = [0.5, 0.5, 0.5]
data.bboxes_3d.rot_axis = 2
data.labels = labels
data.confidences = scores
data.calibs = calib
return data
def visualize(image_path, calib, obj, title, draw_threshold=None):
img = cv2.imread(image_path)
for i in range(len(obj)):
if obj[i].name in ['Car', 'Pedestrian', 'Cyclist']:
if draw_threshold is not None and hasattr(obj[i], 'score'):
if obj[i].score < draw_threshold:
continue
R = rot_y(obj[i].rotation_y)
h, w, l = obj[i].dimensions[0], obj[i].dimensions[1], obj[
i].dimensions[2]
x = [l / 2, l / 2, -l / 2, -l / 2, l / 2, l / 2, -l / 2, -l / 2]
y = [0, 0, 0, 0, -h, -h, -h, -h]
z = [w / 2, -w / 2, -w / 2, w / 2, w / 2, -w / 2, -w / 2, w / 2]
corner_3d = np.vstack([x, y, z])
corner_3d = np.dot(R, corner_3d)
corner_3d[0, :] += obj[i].location[0]
corner_3d[1, :] += obj[i].location[1]
corner_3d[2, :] += obj[i].location[2]
corner_3d = np.vstack((corner_3d, np.zeros((1,
corner_3d.shape[-1]))))
corner_2d = np.dot(calib.P2, corner_3d)
corner_2d[0, :] /= corner_2d[2, :]
corner_2d[1, :] /= corner_2d[2, :]
if obj[i].name == 'Car':
color = [20, 20, 255]
elif obj[i].name == 'Pedestrian':
color = [0, 255, 255]
else:
color = [255, 0, 255]
thickness = 1
for corner_i in range(0, 4):
ii, ij = corner_i, (corner_i + 1) % 4
corner_2d = corner_2d.astype('int32')
cv2.line(img, (corner_2d[0, ii], corner_2d[1, ii]),
(corner_2d[0, ij], corner_2d[1, ij]), color, thickness)
ii, ij = corner_i + 4, (corner_i + 1) % 4 + 4
cv2.line(img, (corner_2d[0, ii], corner_2d[1, ii]),
(corner_2d[0, ij], corner_2d[1, ij]), color, thickness)
ii, ij = corner_i, corner_i + 4
cv2.line(img, (corner_2d[0, ii], corner_2d[1, ii]),
(corner_2d[0, ij], corner_2d[1, ij]), color, thickness)
box_text = obj[i].name
if hasattr(obj[i], 'score'):
box_text += ': {:.2}'.format(obj[i].score)
cv2.putText(img, box_text,
(min(corner_2d[0, :]), min(corner_2d[1, :]) - 2),
cv2.FONT_HERSHEY_COMPLEX_SMALL, 0.5, color, 1)
cv2.putText(img, title, (int(img.shape[1] / 2), 20),
cv2.FONT_HERSHEY_SIMPLEX, 0.75, (255, 100, 0), 2)
return img
def main(args):
calib, gt_obj = parse_gt_info(args.calib_file, args.label_file)
gt_image = visualize(args.image_file, calib, gt_obj, title='GroundTruth')
pred = parse_pred_info(args.pred_file, [
calib.P0, calib.P1, calib.P2, calib.P3, calib.R0_rect,
calib.Tr_velo_to_cam, calib.Tr_imu_to_velo
])
preds = predictions_to_kitti_format(pred)
pred_image = visualize(
args.image_file,
calib,
preds,
title='Prediction',
draw_threshold=args.draw_threshold)
show_image = np.vstack([gt_image, pred_image])
cv2.imwrite(
osp.join(args.save_dir,
osp.split(args.image_file)[-1]), show_image)
if __name__ == '__main__':
args = parse_args()
main(args)
|