【量化交易】永久投资组合,海龟交易法阅读,回测与讨论
首先和大家分享一个真实的故事。 根据网上北京市,1991年至2021年平均工资年鉴。 北京市1991年平均工资为2877元,2021年平均工资为145766元。 30年差了50.66倍。 也就是说91年,你每月能赚240元,在北京就算中等收入水平。而30年后,你收入每月赚的是91年的10倍,2400元,在北京依然算是特别落后的一部分。 由此可见通货膨胀的可怕, 和资本是如何通过,通货膨胀,剥削榨取普通人的资产的。 因此,通过理财手段,抵消通货膨胀带来的负面影响。 可以说是普通人想完成阶级跨越,或者说保持原有阶级不变的必要手段。 正所谓“你不理财,财不理你。”古人诚不欺我。
理财的类型,主要分为: 1.定期存款 2.银行理财 3.货币基金 4.国债 5.股票基金 6.黄金贵金属 当然,像投资房产,古董等,都和黄金类似,都有很好的抗通胀属性。 不过正所谓“鸡蛋不要放在一个篮子里。”,我们还是要考虑到,例如21年房地产行业整体下行的情况。如果把所有资产都投入到一个地方。当风险来临时,如何抵御风险。 下面给大家介绍一下一种投资组合,永久投资组合。 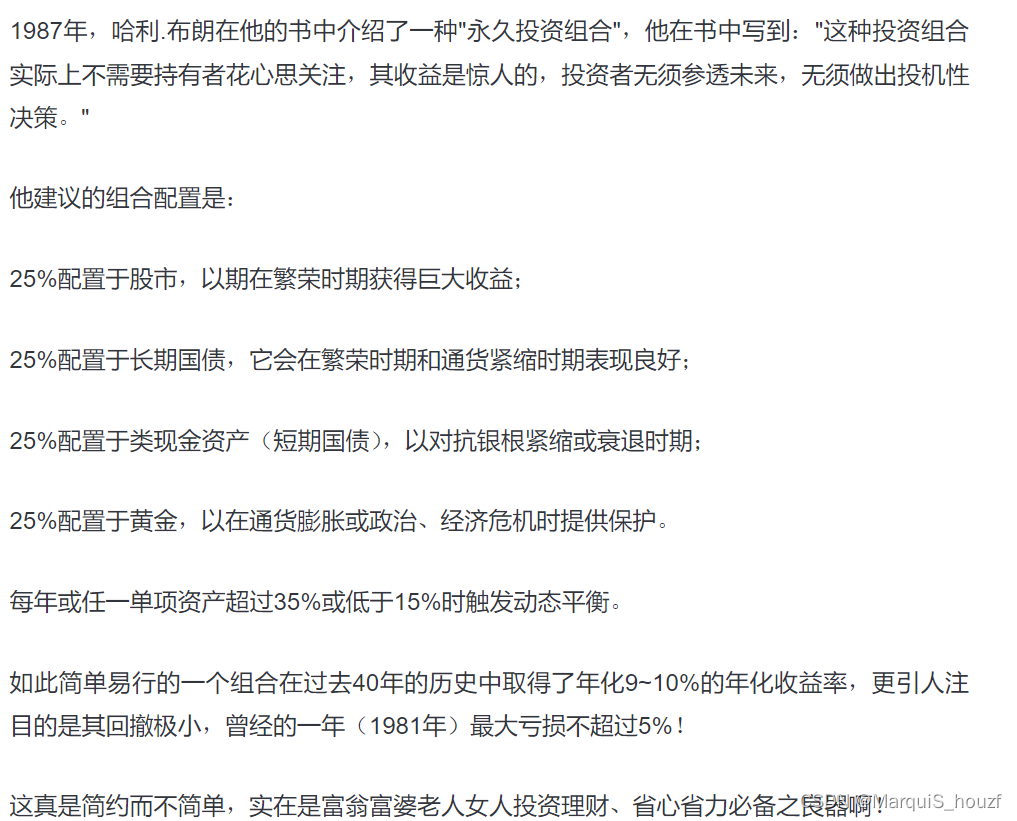 如果不是想通过投机(赌博)致富。就可以参考这个投资组合。由于里面做了一些对冲,导致可以有良好的持有体验。
下面是其他人对永久投资组合做的回测: 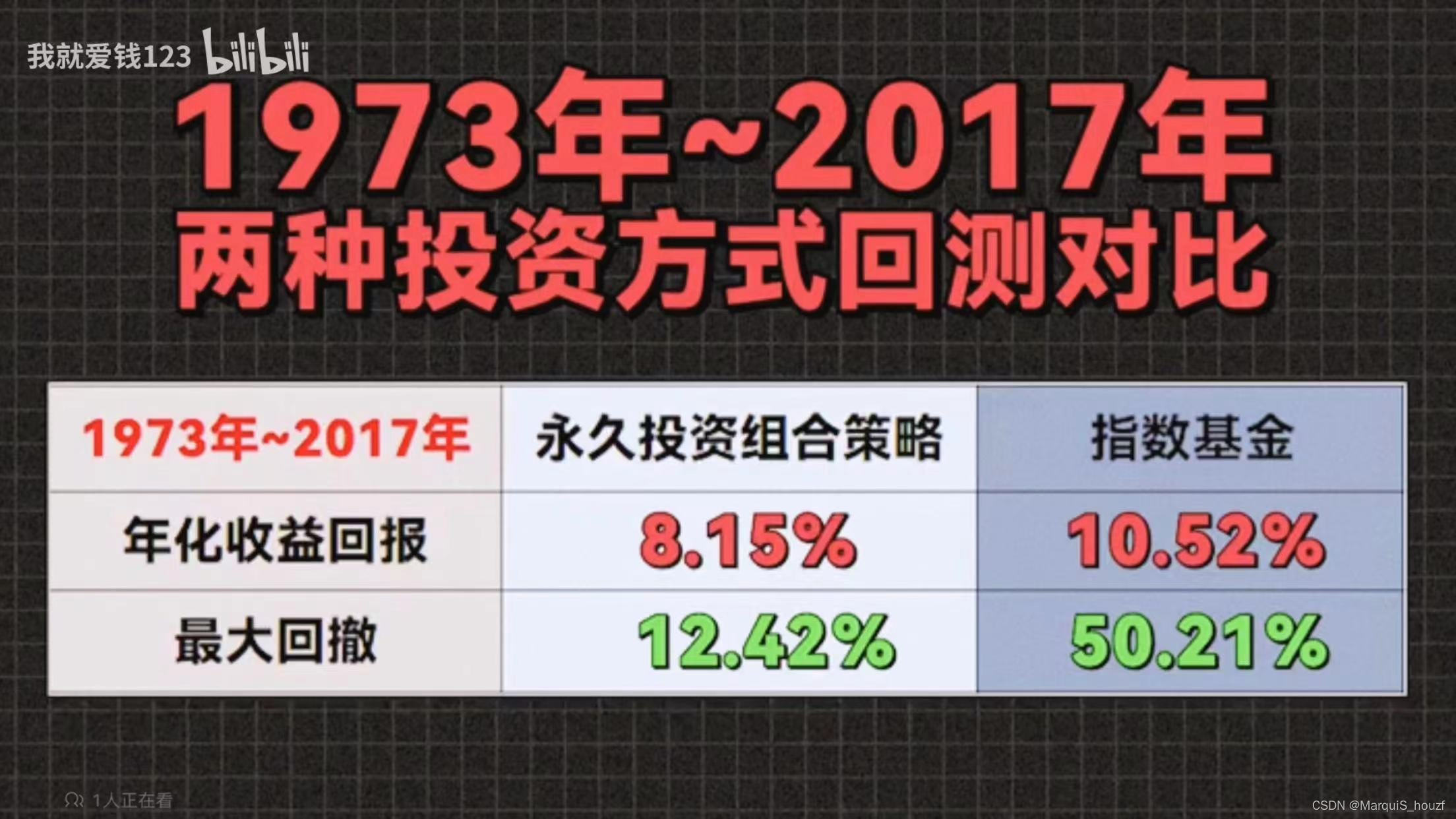 显然指数基金虽然收益回报赚的多一点,但是最大回撤(也就是暂时赔的钱)比例却大很多。当然持有体验不如永久投资组合,只适合心里承受能力很强的人去考虑。 当然,永久投资组合还是有很多可以优化的空间。 例如,长期国债和黄金投资都有很好的,抵抗通货膨胀属性。 以笔者为参考,长期国债相较于黄金,普通人更难购买。 显然,可以选择将黄金比例提高,或者用指数基金可以做一些相似性代替。 然后其中收益最高,风险也最大的股票投资部分。则可以考虑,通过今天的主题,海龟交易法则,进行优化。 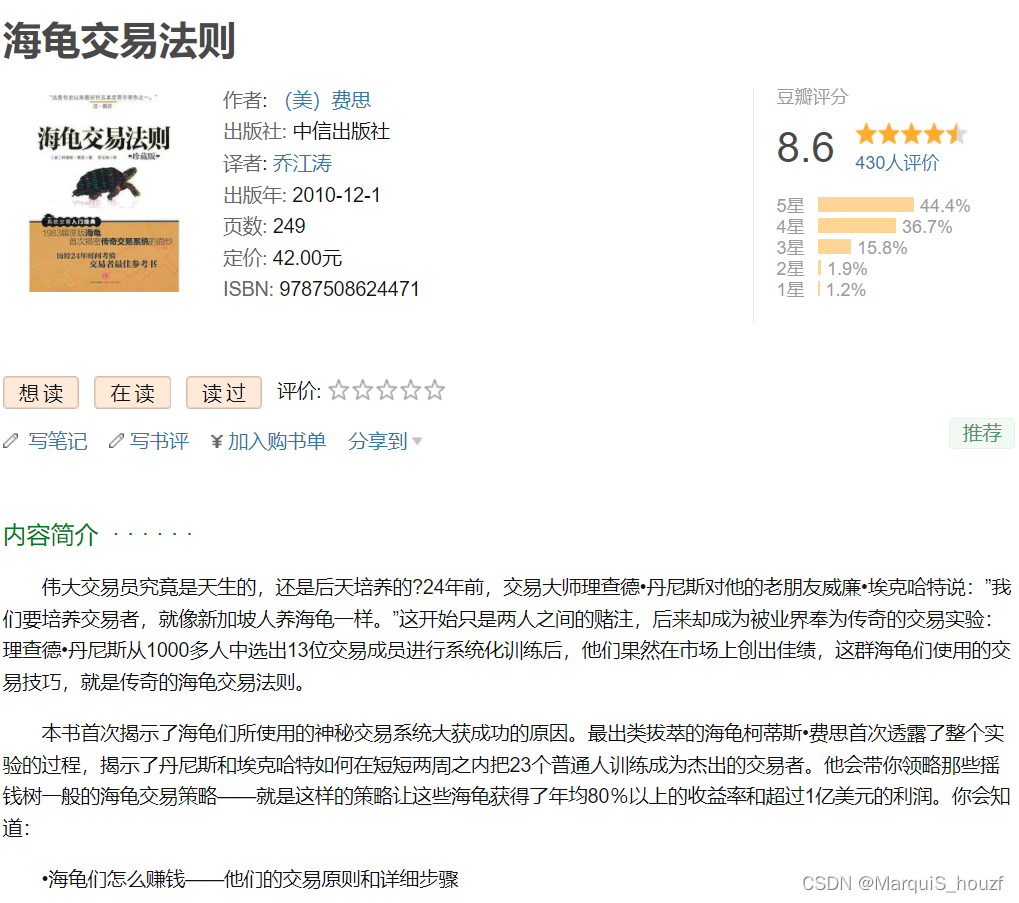
有本书叫《祖鲁法则》是讲关于选股的,上面有一个观点和就像美国股神巴菲特一样,讲的是要在熟悉的领域进行操作。 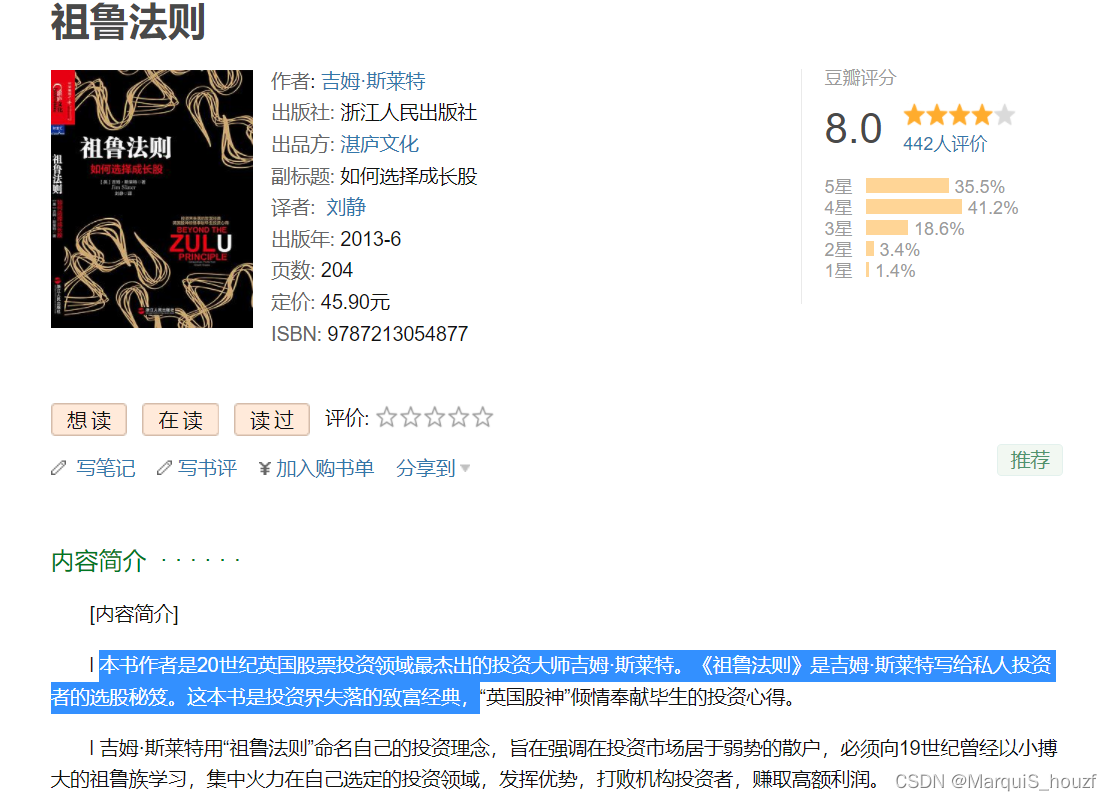 显然,海龟交易法则测试的发起人,理查德.丹尼斯,以400美元作为本金,10多年时间,赚取2个多亿的投资大师。就是对于投机领域的专业人士。 海龟交易法则,简单的说,就是以,股票这种零和博弈的本质就是反人性的。所以要用绝对理性的纪律,来实践计划的数学模型。这样才能减少感性主观判断带来的失误操作。
具体的交易原则,笔者总结后为: 1.配资策略:只能有四个头寸单位,关联品种头寸单位不能超过6个; 2.入场策略:突破20日(MA20,短线)或者50日(MA50,长线)平均价格的最高点,入场做多: (操作细节: 1.使用限价单,不用市价单。限价单可以根据市价取低点,这样完成更多的低卖,可以实现盈利最大化。 2.对于基金股票类N+1天交易类型,最好在尾盘操作,避免盘中波动风险。 ) 3.止损策略:止损点位是10日或者20日波动幅度平均值(ATR)。 (例如,20日波动幅度平均值(ATR)n为2,则2n为4。20日(MA20)平均价格为20,止损点位则为16。) 4.出场策略:过去20天最低价就要出场;
数据建模代码如下:
from jqdata import *
import numpy
def initialize(context):
set_params()
set_variables()
set_backtest()
run_daily(daily, time='every_bar')
def set_params():
g.security = '600519.XSHG'
g.short_in_date = 20
g.short_out_date = 10
g.dollars_per_share = 1
g.loss = 0.1
g.number_days = 20
g.unit_limit = 4
def set_slip_fee(context):
set_slippage(FixedSlippage(0))
dt=context.current_dt
def set_variables():
g.unit = 1000
g.N = []
g.break_price = 0
g.sys = 0
g.high=[]
g.low=[]
def set_backtest():
set_benchmark(g.security)
set_option('use_real_price',True)
log.set_level('order','error')
def before_trading_start(context):
set_slip_fee(context)
calculate_N()
tqatds()
tqatdx()
dt=context.current_dt
if dt>datetime.datetime(2013,1, 1):
set_commission(PerTrade(buy_cost=0.0003, sell_cost=0.0013, min_cost=5))
elif dt>datetime.datetime(2011,1, 1):
set_commission(PerTrade(buy_cost=0.001, sell_cost=0.002, min_cost=5))
elif dt>datetime.datetime(2009,1, 1):
set_commission(PerTrade(buy_cost=0.002, sell_cost=0.003, min_cost=5))
else:
set_commission(PerTrade(buy_cost=0.003, sell_cost=0.004, min_cost=5))
def daily(context):
price=attribute_history(g.security, 1, '1m', 'close')
current_price=price['close'][-1]
value=context.portfolio.portfolio_value
cash=context.portfolio.cash
Dollar_Volatility=g.N[-1]*1
g.unit=value*0.01/Dollar_Volatility
if g.sys==0:
market_in(context,current_price,g.short_in_date)
else:
stop_loss(current_price)
market_add(context,current_price,g.short_in_date)
market_out(current_price, g.short_out_date)
def tqatds():
price=attribute_history(g.security,20,'1d',('high','low','close'))
g.high.append(max(price['high']))
return g.high
def tqatdx():
price=attribute_history(g.security,20,'1d',('high','low','close'))
g.low.append(min(price['low']))
return g.low
def calculate_N():
if len(g.N)==0:
price=attribute_history(g.security,21,'1d',('high','low','close'))
st1=[]
for i in range(1,21):
hl=price['high'][i]-price['low'][i]
hc=price['high'][i]-price['close'][i-1]
cl=price['close'][i-1]-price['low'][i]
True_Range=max(hl,hc,cl)
st1.append(True_Range)
current_N=round(np.mean(np.array(st1)),3)
g.N.append(current_N)
else:
price = attribute_history(g.security, 2, '1d',('high','low','close'))
hl = price['high'][-1]-price['low'][-1]
hc = price['high'][-1]-price['close'][-2]
cl = price['close'][-2]-price['low'][-1]
True_Range = max(hl, hc, cl)
current_N = round((True_Range + (g.number_days-1)*(g.N)[-1])/g.number_days,3)
(g.N).append(current_N)
def market_in(context,current_price,in_date):
price=attribute_history(g.security,in_date,'1d', 'close')
if current_price > g.high[-1]:
cash=context.portfolio.available_cash
num_of_shares=cash/current_price
if num_of_shares>=g.unit and g.sys<int(g.unit_limit*g.unit):
log.info('SYS买入',g.unit)
order(g.security,int(g.unit))
g.sys+=int(g.unit)
g.break_price=current_price
def market_add(context,current_price,in_date):
if current_price>=g.break_price+0.5*g.N[-1]:
cash=context.portfolio.available_cash
num_of_shares=cash/current_price
if num_of_shares>=g.unit and g.sys<int(g.unit_limit*g.unit):
log.info('g.sys加仓{}:{}'.format(g.security,current_price))
order(g.security,int(g.unit))
g.sys+=int(g.unit)
g.break_price=current_price
def market_out(current_price,out_date):
price=attribute_history(g.security,out_date,'1d',('close','low'))
if current_price<g.low[-1] and g.sys>0:
log.info('SYS离场')
order_target(g.security, 0)
g.sys=0
def stop_loss(current_price):
if current_price<g.break_price-2*g.N[-1]:
log,info('SYS止损')
order_target(g.security, 0)
g.sys=0
海龟交易法则与贵州茅台股票回测图如下: 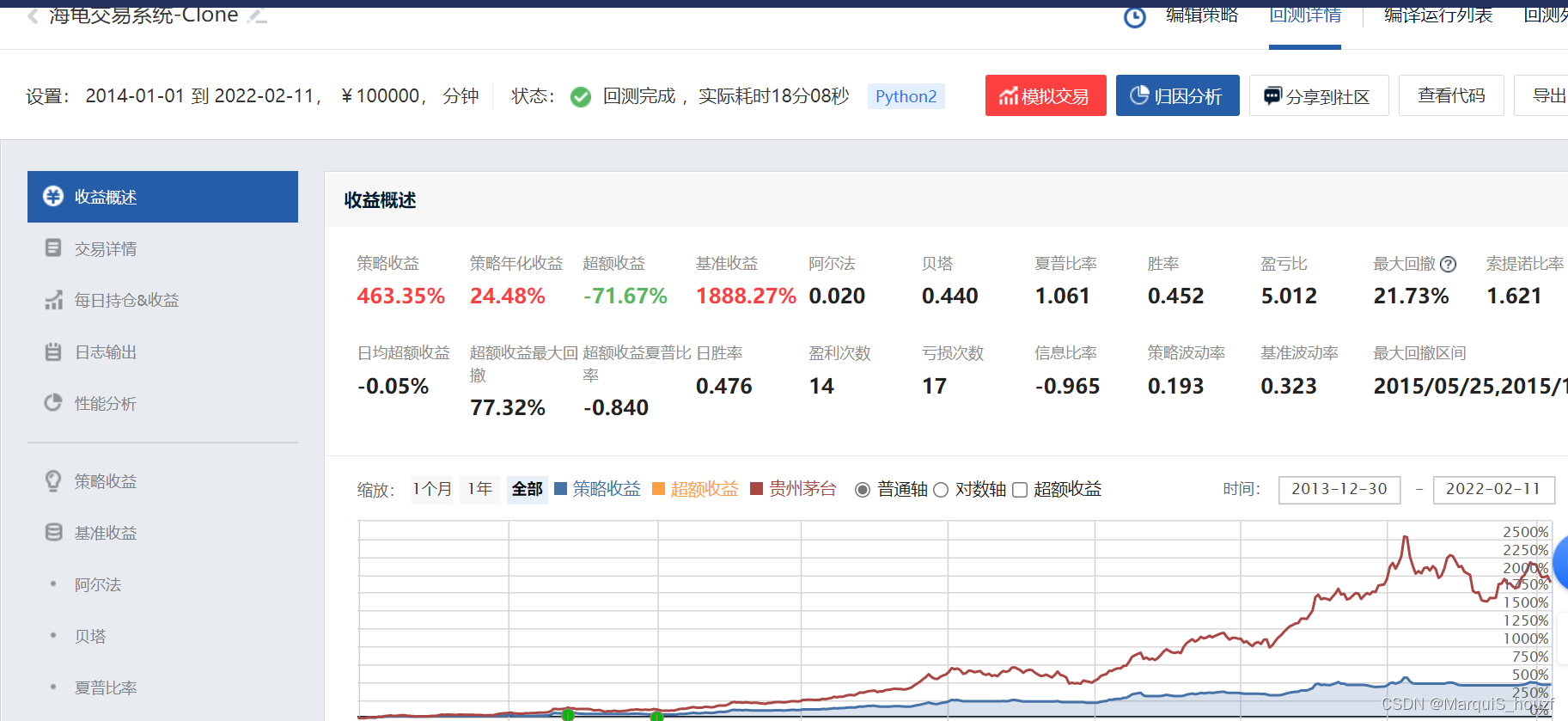 从回测图可以明显看出,海龟交易法则是跑不赢,图中对比的,贵州茅台股票的收益率。 所以说交易法则再好,也抵不过风向趋势。 像巴菲特说的“好机会不常来,来了请用桶接。” 其实实现人生转折,阶级跨越的机会是总会来的。只看你是否能发现并掌握住。 最后和大家分享一下, 美国十大财团之首,石油大亨,白手起家的洛克菲勒的作品。《洛克菲勒写给儿子的38封信》。古人说“富不过三代。”然而这本书使他的家族已经六代不衰败。 关键信息总结为: 1.信心是成功之父; 2.不断提升认知; 3.行动是关键; 当然本文都是笔者近期阅读过上面几本书,然后通过程序模拟回测之后的,一些个人看法。非常欢迎大家参与讨论。
|