自定义排序WritableComparable 要想利用框架提供的排序机制,需要做两步:
第一步:把需要排序的数据放到mapper的keyout的位置,这样框架才会对我们所有的kv数据按照key进行排序。如果该数据是个自定义的bean对象,则需要进行第二步。
/***********************************************/
第二步:告知框架按照bean的哪个属性排序,按照升序还是按照降序。也就是让自定义的类实现WritableComparable接口重写compareTo方法,让该方法返回-1、1、或者0,就可以实现排序。
文本内容:
13329142740 5 60 8 3 76
13436755071 5 20 18 28 71
13436773954 12 51 12 40 115
13439205555 10 43 14 46 113
13535755061 2 30 58 25 115
13538774952 3 22 3 33 61
13539142240 3 6 8 1 18
13539282765 5 20 9 29 63
13636673964 3 32 5 50 90
13636744666 16 40 21 86 163
13636873563 5 10 2 23 40
13639215592 6 20 5 25 56
13736344595 6 22 2 22 52
13836764655 9 30 4 40 83
13933139985 3 15 8 8 34
13939119984 3 5 7 8 23
17612591478 8 20 8 16 52
17813591678 6 25 8 12 51
对文件中的最后一项数据,即手机总费用进行排序:
分析: 利用框架进行排序,要做三步:
1.把要排序的字段置于mapper的keyout,因为总花费位于bean中,因此,要让bean位于mapper的keyout。
2.让bean实现WritableComparable接口,重写compareTo方法,通过该方法告知框架我们要按照bean的总花费进行排序,按照升序排序。
3.既然bean作为keyout,那么手机号就要当valueout
a)Mapper端读取每行数据封装bean,context.write(bean<总话费>,手机号)
b)Phone类实现WritableComparable接口重写compareTo方法
c)Reduce无需做特殊处理,将kv原样写出即可。
写pojo类
package com.bigdata.paixu;
import java.io.DataInput;
import java.io.DataOutput;
import java.io.IOException;
import org.apache.hadoop.io.WritableComparable;
//1 实现writable接口
public class Phone1 implements WritableComparable<Phone1>{
//套餐基本费
private long baseFee;
//语音通信费
private long communicateFee;
//短信彩信费
private long msgFee;
//流量费
private long flowFee;
//总费用
private long sumFee;
//2 反序列化时,需要反射调用空参构造函数,所以必须有空参构造
public Phone1() {
super();
}
public Phone1(long baseFee, long communicateFee, long msgFee, long flowFee, long sumFee) {
super();
this.baseFee = baseFee;
this.communicateFee = communicateFee;
this.msgFee = msgFee;
this.flowFee = flowFee;
this.sumFee = sumFee;
}
//设置参数的便利方法
public void setFee(long baseFee, long communicateFee, long msgFee, long flowFee) {
this.baseFee = baseFee;
this.communicateFee = communicateFee;
this.msgFee = msgFee;
this.flowFee = flowFee;
this.sumFee = baseFee + communicateFee + msgFee + flowFee;
}
//3 写序列化方法
public void write(DataOutput out) throws IOException {
out.writeLong(baseFee);
out.writeLong(communicateFee);
out.writeLong(msgFee);
out.writeLong(flowFee);
out.writeLong(sumFee);
}
//4 反序列化方法
//5 反序列化方法读顺序必须和写序列化方法的写顺序必须一致
public void readFields(DataInput in) throws IOException {
baseFee = in.readLong();
communicateFee = in.readLong();
msgFee = in.readLong();
flowFee = in.readLong();
sumFee = in.readLong();
}
// 6 编写toString方法,方便后续打印到文本
public String toString() {
return baseFee+"\t"+communicateFee+"\t"+msgFee+"\t"+flowFee+"\t"+sumFee;
}
public long getBaseFee() {
return baseFee;
}
public void setBaseFee(long baseFee) {
this.baseFee = baseFee;
}
public long getCommunicateFee() {
return communicateFee;
}
public void setCommunicateFee(long communicateFee) {
this.communicateFee = communicateFee;
}
public long getMsgFee() {
return msgFee;
}
public void setMsgFee(long msgFee) {
this.msgFee = msgFee;
}
public long getFlowFee() {
return flowFee;
}
public void setFlowFee(long flowFee) {
this.flowFee = flowFee;
}
public long getSumFee() {
return sumFee;
}
public void setSumFee(long sumFee) {
this.sumFee = sumFee;
}
public int compareTo(Phone1 o) {
int res = 0;
if(this.getSumFee() > o.getSumFee()){
res = 1;
}else if(this.getSumFee() < o.getSumFee()){
res = -1;
}
return res;
}
}
建Mapper类:
package com.bigdata.paixu;
import org.apache.hadoop.io.LongWritable;
import org.apache.hadoop.io.Text;
import org.apache.hadoop.mapreduce.Mapper;
import java.io.IOException;
public class PhoneMapper1 extends Mapper<LongWritable, Text, Phone1, Text> {
Phone1 k = new Phone1();
Text v = new Text();
@Override
protected void map(LongWritable key, Text value, Context context)
throws IOException, InterruptedException {
// 1 获取一行,转化成String类型
//13329142740 5 60 8 3 76
String line = value.toString();
// 2 按照tab键切分字段,
String[] split = line.split("\t");
// 3 挑出手机号、总套餐基本费、总语音通信费、总短信彩信费、总流量费,总话费,封装对象
String phoneNum = split[0];
v.set(phoneNum);
long baseFee = Long.parseLong(split[1]);
long communicateFee = Long.parseLong(split[2]);
long msgFee = Long.parseLong(split[3]);
long flowFee = Long.parseLong(split[4]);
k.setFee(baseFee,communicateFee,msgFee,flowFee);
// 4 将kv写出,以bean为keyout,以手机号为valueout
context.write(k, v);
}
}
建Reduce类:
package com.bigdata.paixu;
import java.io.IOException;
import org.apache.hadoop.io.Text;
import org.apache.hadoop.mapreduce.Reducer;
public class PhoneReduce1 extends Reducer<Phone1, Text, Text, Phone1>{
@Override
protected void reduce(Phone1 key, Iterable<Text> values, Context context)
throws IOException, InterruptedException {
//无需做额外操作,直接循环写出即可
for (Text text : values) {
context.write(text, key);
}
}
}
建driver类:
package com.bigdata.paixu;
import org.apache.hadoop.conf.Configuration;
import org.apache.hadoop.fs.FileSystem;
import org.apache.hadoop.fs.Path;
import org.apache.hadoop.io.Text;
import org.apache.hadoop.mapreduce.Job;
import org.apache.hadoop.mapreduce.lib.input.FileInputFormat;
import org.apache.hadoop.mapreduce.lib.output.FileOutputFormat;
public class PhoneDriver1 {
public static void main(String[] args) throws Exception {
//1,获取配置信息,组装job
Configuration conf = new Configuration();
Job job = Job.getInstance(conf);
//2,设置jar包的位置
job.setJarByClass(PhoneDriver1.class);
//3,设置mapper、reduce类
job.setMapperClass(PhoneMapper1.class);
job.setReducerClass(PhoneReduce1.class);
//4,设置mapper的keyout,valueout类型
job.setMapOutputKeyClass(Phone1.class);
job.setMapOutputValueClass(Text.class);
//5,设置最终keyout,valueout的类型
job.setOutputKeyClass(Text.class);
job.setOutputValueClass(Phone1.class);
//6,设置数据的输入路径,结果的输出路径
FileInputFormat.setInputPaths(job, new Path(args[0]));
Path outPath = new Path(args[1]);
FileSystem fs = FileSystem.get(conf);
if(fs.exists(outPath)){
fs.delete(outPath,true);
}
FileOutputFormat.setOutputPath(job, new Path(args[1]));
//7,提交任务
boolean b = job.waitForCompletion(true);
System.out.println("程序执行 :"+b);
}
}
输出结果: 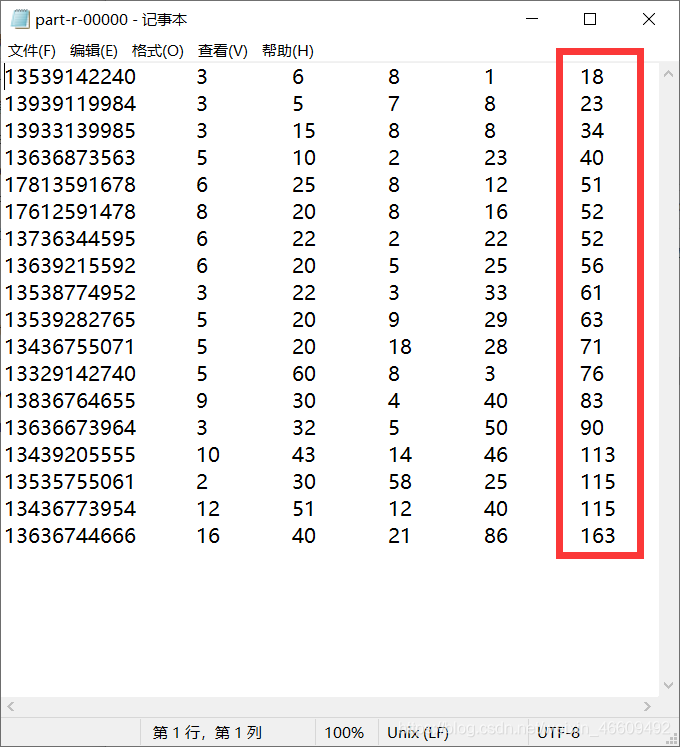
|