Yarn模式
Yarn是一个分布式集群资源管理框架,在Yarn集群上可以部署运行各种分布式式应用程序。例如:Mapreduce,Spark。Yarn框架为这些分布式应用程序运行提供了可靠的支持。
因为在生产开发环境中很少使用Standalone模式,Flink On Yarn模式用的稍微多一点,今天我们就来讲讲Flink On Yarn
第一种方式
在Yarn集群中初始化一个Flink集群,该Flink集群占用着指定的资源,以后的Flink作业都会向这个Flink集群提交,这个Flink作业会常驻于Yarn集群中 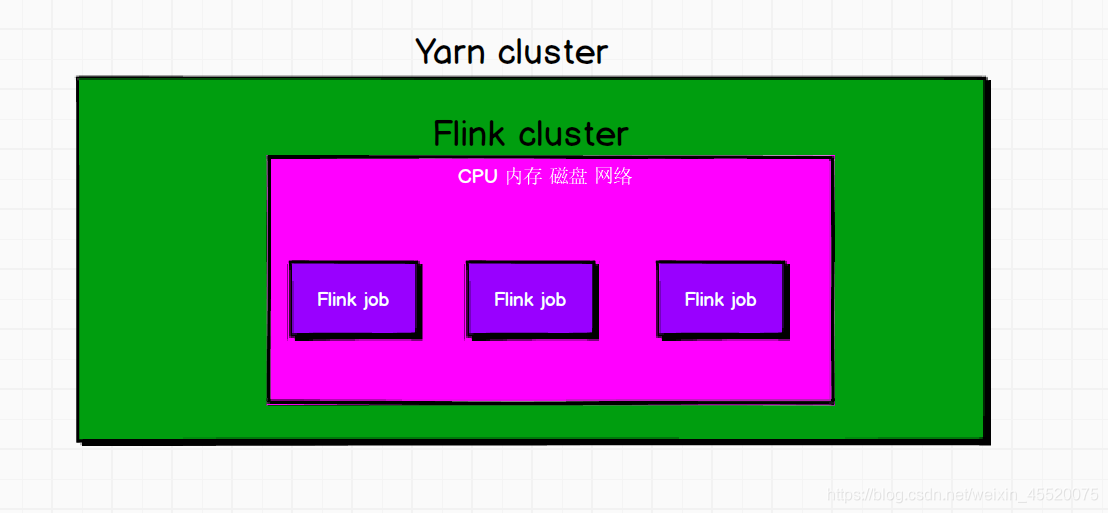
第二种方式
每次提交Flink作业的时候都会在Yarn集群上创建一个独立的Flink集群,与上面的不同的是,该提交的Flink作业之间相互独立不受影响,等到Filnk作业执行完毕之后就会释放资源 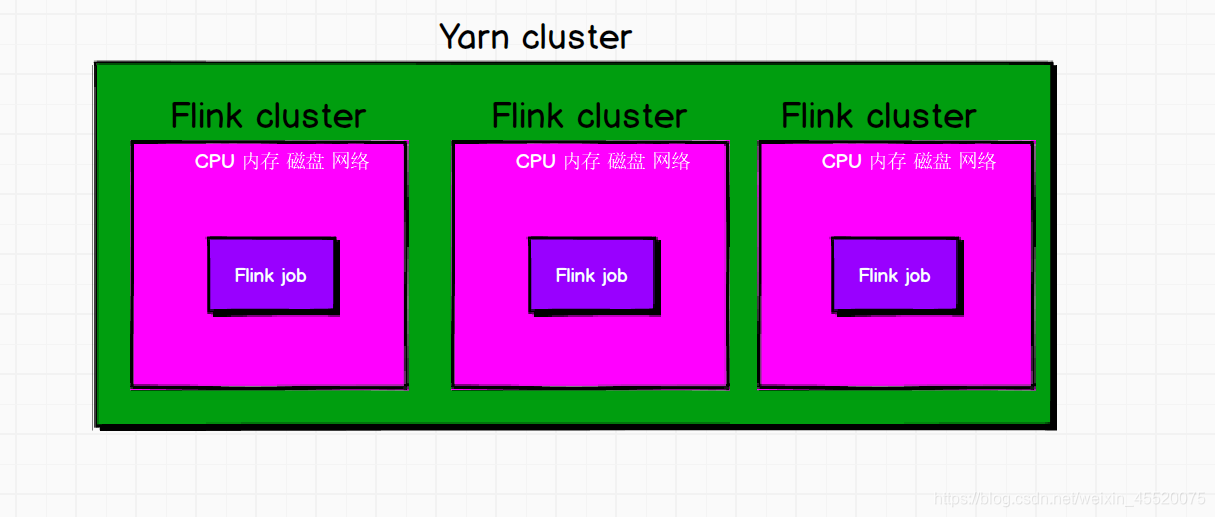
在Yarn集群中启动一个Flink集群
我们可以进入到Flink的bin目录下找到与之相关联的启动脚本 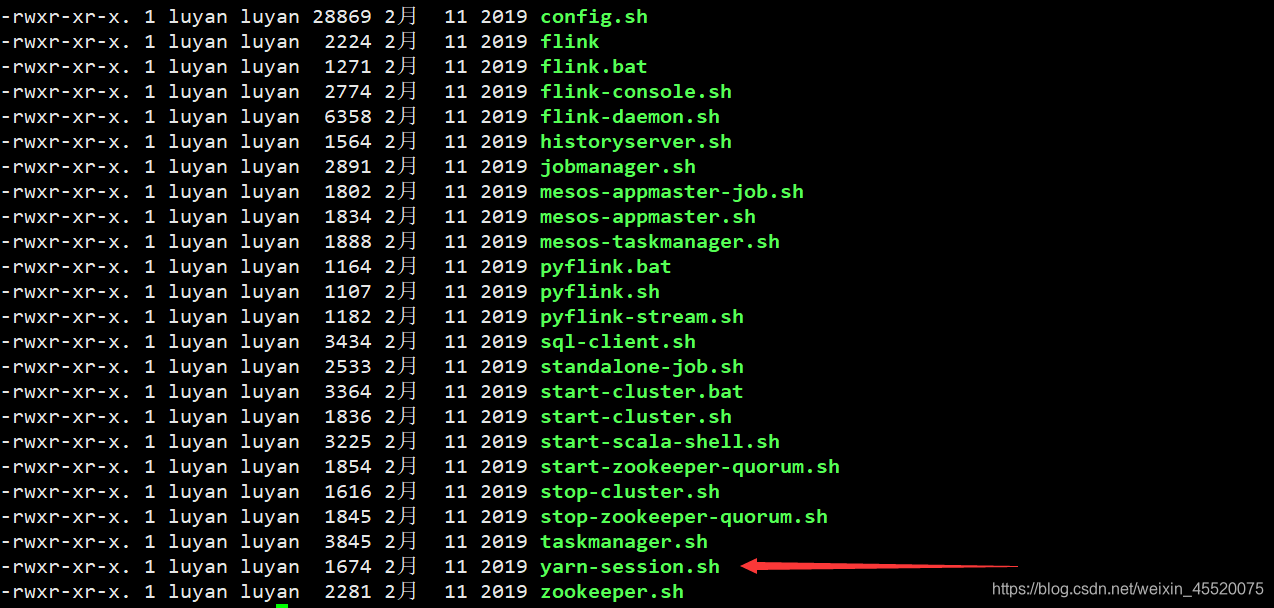
[luyan@hadoop114 bin]$ ./yarn-session.sh
执行这个脚本之后,控制台上就会打印Flink集群的各种信息。最终要的一点我们会看到如下信息: JobManager Web Interface: http://hadoop114:51775,这个表示在Yarn集群中初始化一个Flink集群的Web UI地址,如下图: 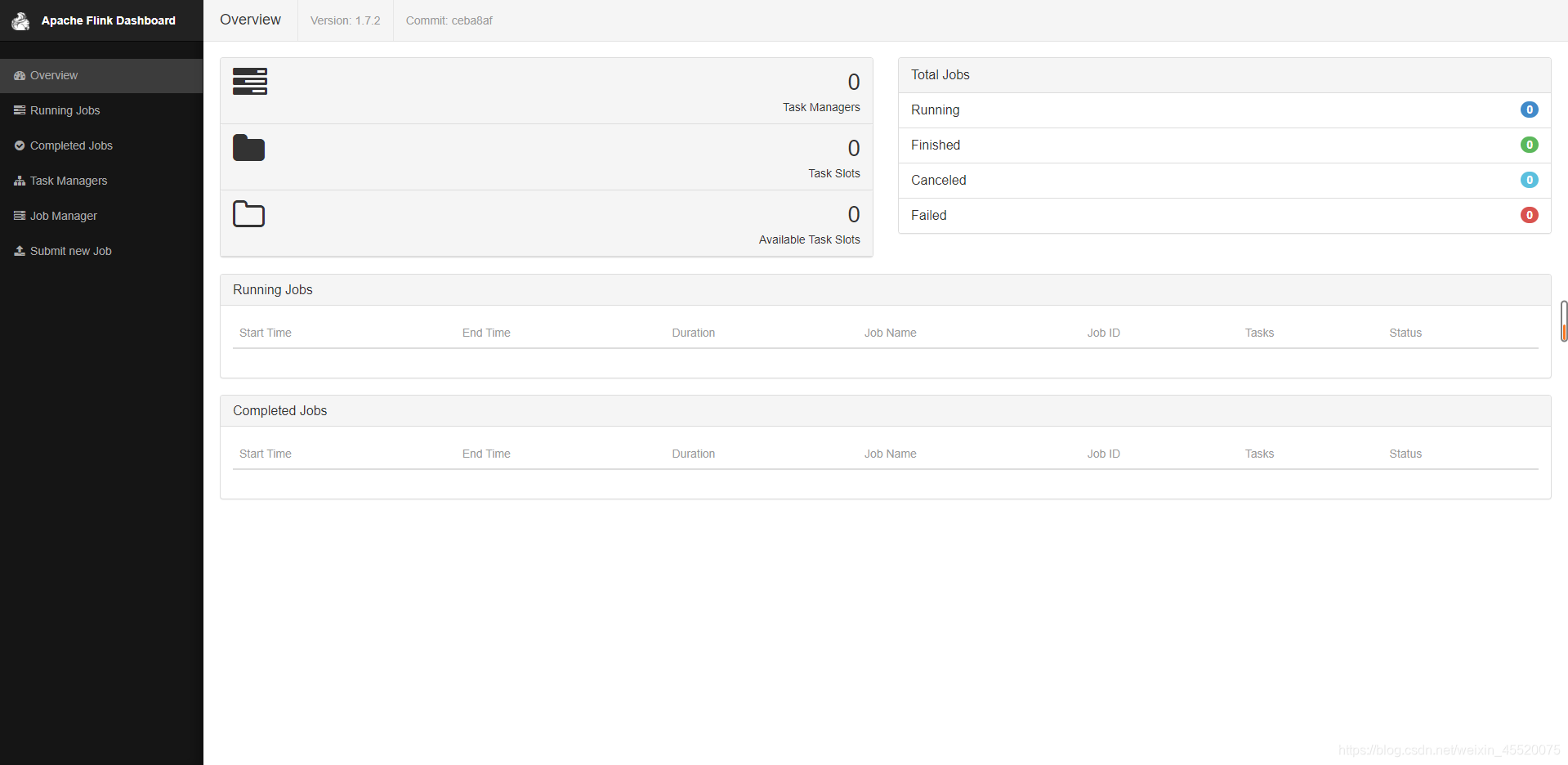 下面我们来介绍关于yarn-session的一些常用的命令 在调用yarn-session.sh脚本的时候,指定-h参数可以查看该脚本提供的可选参数:
[luyan@hadoop114 bin]$ ./yarn-session.sh -h
Usage:
Required
-n,--container <arg> Number of YARN container to allocate (=Number of Task Managers)
Optional
-D <property=value> use value for given property
-d,--detached If present, runs the job in detached mode
-h,--help Help for the Yarn session CLI.
-id,--applicationId <arg> Attach to running YARN session
-j,--jar <arg> Path to Flink jar file
-jm,--jobManagerMemory <arg> Memory for JobManager Container with optional unit (default: MB)
-m,--jobmanager <arg> Address of the JobManager (master) to which to connect. Use this flag to connect to a different JobManager than the one specified in the configuration.
-n,--container <arg> Number of YARN container to allocate (=Number of Task Managers)
-nl,--nodeLabel <arg> Specify YARN node label for the YARN application
-nm,--name <arg> Set a custom name for the application on YARN
-q,--query Display available YARN resources (memory, cores)
-qu,--queue <arg> Specify YARN queue.
-s,--slots <arg> Number of slots per TaskManager
-sae,--shutdownOnAttachedExit If the job is submitted in attached mode, perform a best-effort cluster shutdown when the CLI is terminated abruptly, e.g., in response to a user interrupt, such
as typing Ctrl + C.
-st,--streaming Start Flink in streaming mode
-t,--ship <arg> Ship files in the specified directory (t for transfer)
-tm,--taskManagerMemory <arg> Memory per TaskManager Container with optional unit (default: MB)
-yd,--yarndetached If present, runs the job in detached mode (deprecated; use non-YARN specific option instead)
-z,--zookeeperNamespace <arg> Namespace to create the Zookeeper sub-paths for high availability mode
- -q,–query:该参数用于显示Yarn集群中可用的资源,
并不会开启一个Yarn会话
[luyan@hadoop114 bin]$ ./yarn-session.sh -q
NodeManagers in the ClusterClient 1|Property |Value
+---------------------------------------+
|NodeID |hadoop114:43463
|Memory |8192 MB
|vCores |8
|HealthReport |
|Containers |0
+---------------------------------------+
Summary: totalMemory 8192 totalCores 8
Queue: default, Current Capacity: 0.0 Max Capacity: 1.0 Applications: 0
- 以指定的参数来启动一个Flink集群
默认情况下启动Flink集群将使用flink-conf.yaml文件中的配置 ,我们使用 -jm 参数给Flink集群的作业管理器分配1024MB的内存空间,-tm 参数给任务管理器分配1024MB的内存空间,-s 指定每个任务管理器的任务槽数,可以看到启动的时候打印的日志就会显示当前的配置参数的情况
[luyan@hadoop114 bin]$ ./yarn-session.sh -jm 1024m -tm 1024m -s 1 -nm luyanFlink
2021-07-23 06:44:45,070 INFO org.apache.flink.configuration.GlobalConfiguration - Loading configuration property: jobmanager.rpc.address, localhost
2021-07-23 06:44:45,072 INFO org.apache.flink.configuration.GlobalConfiguration - Loading configuration property: jobmanager.rpc.port, 6123
2021-07-23 06:44:45,072 INFO org.apache.flink.configuration.GlobalConfiguration - Loading configuration property: jobmanager.heap.size, 1024m
2021-07-23 06:44:45,072 INFO org.apache.flink.configuration.GlobalConfiguration - Loading configuration property: taskmanager.heap.size, 1024m
2021-07-23 06:44:45,072 INFO org.apache.flink.configuration.GlobalConfiguration - Loading configuration property: taskmanager.numberOfTaskSlots, 1
2021-07-23 06:44:45,072 INFO org.apache.flink.configuration.GlobalConfiguration - Loading configuration property: parallelism.default, 1
2021-07-23 06:44:45,073 INFO org.apache.flink.configuration.GlobalConfiguration - Loading configuration property: rest.port, 8081
[luyan@hadoop114 bin]$ ./yarn-session.sh -d
JobManager Web Interface: http://hadoop114:56083
2021-07-23 07:00:38,211 INFO org.apache.flink.yarn.cli.FlinkYarnSessionCli - The Flink YARN client has been started in detached mode. In order to stop Flink on YARN, use the following command or a YARN web interface to stop it:
yarn application -kill application_1626992692657_0004
以上截取了部分的重要信息,这个时候就无法通过停止Linux进程或者在客户端输入stop来停止会话,需要获取该Yarn会话的ID,使用Yarn命令进行关闭
yarn application -kill application_1626992692657_0004 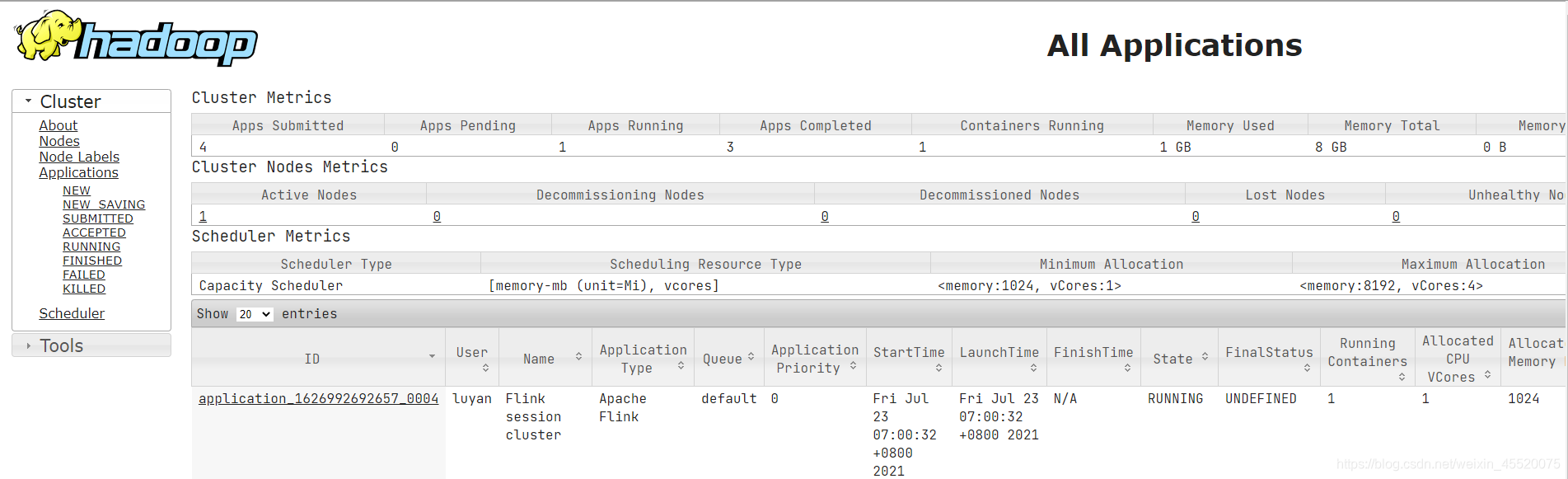
提交作业到Filnk集群中
在Yarn集群中初始化一个Flink集群之后,我们就可以提交Flink作业至上面运行,如下命令:
[luyan@hadoop114 flink-1.7.2]$ bin/flink run ./examples/batch/WordCount.jar
此时Flink集群的Web管控台的资源使用情况如下: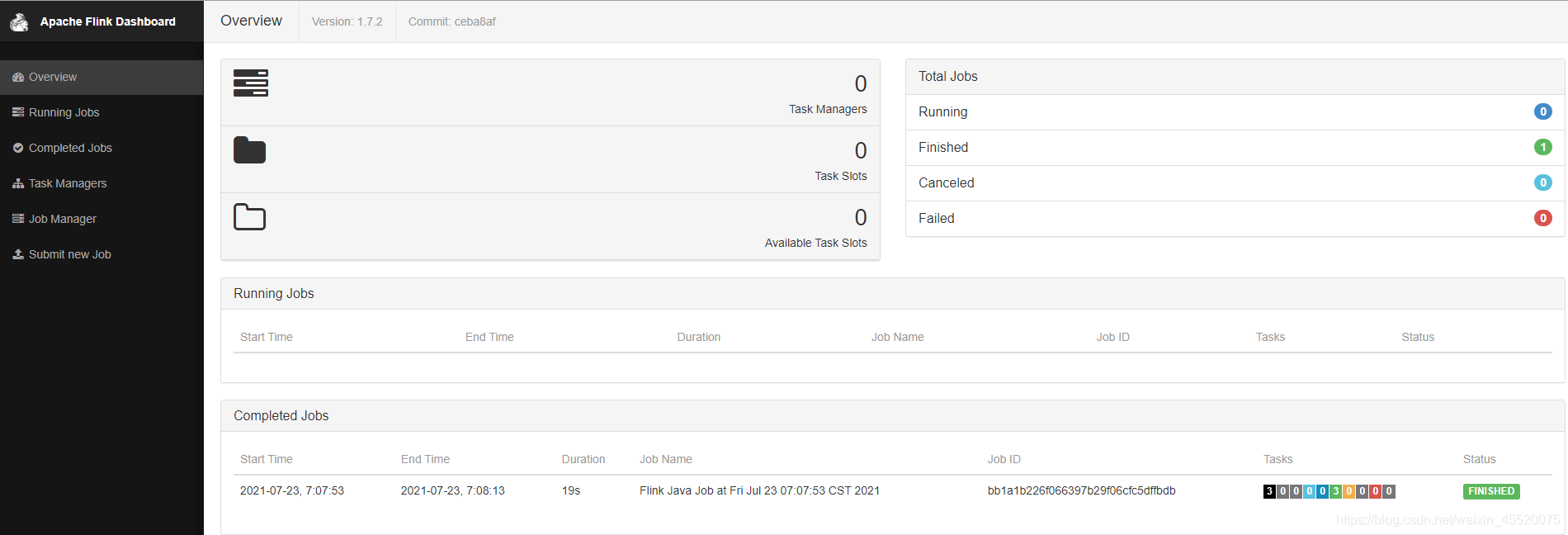
在Yarn集群中运行独立的Flink作业
此模式一个Flink作业会被开辟一个单独的Flink集群,我们可以直接通过bin/flink这个脚本启动,只需要加 -m yarn-cluster即可,Flink作业运行完之后,创建的Flink集群也会随之消失。
启动之后进入YARN的管控台可以看到 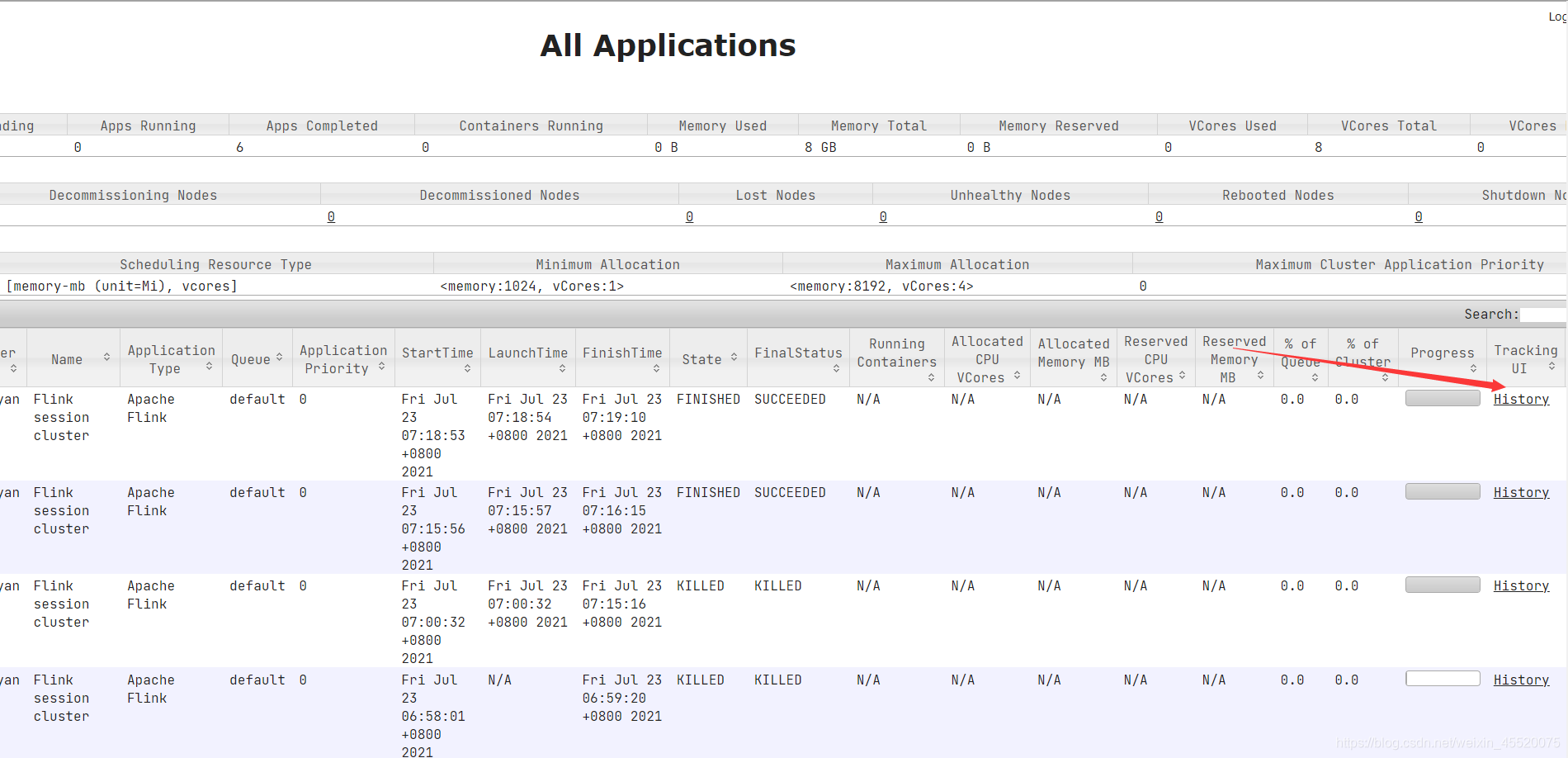 点击对应的会话的Tracking UI可进入当前运行的Flink集群的管控台页面,如下: 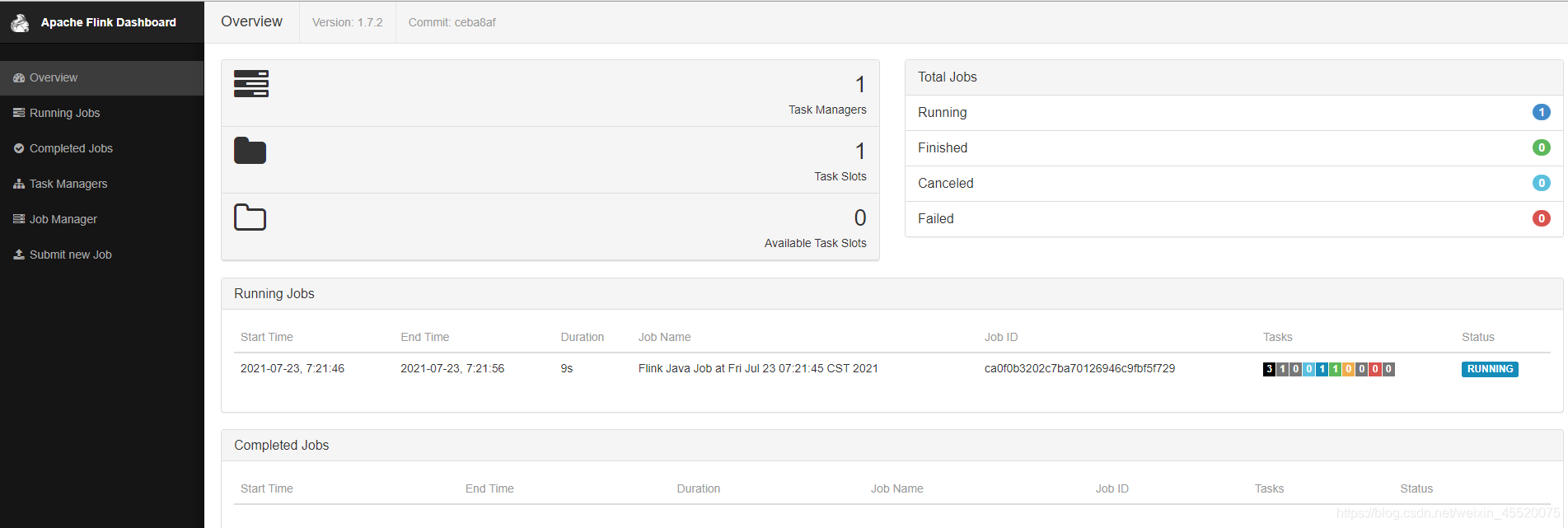
Flink和Yarn的交互
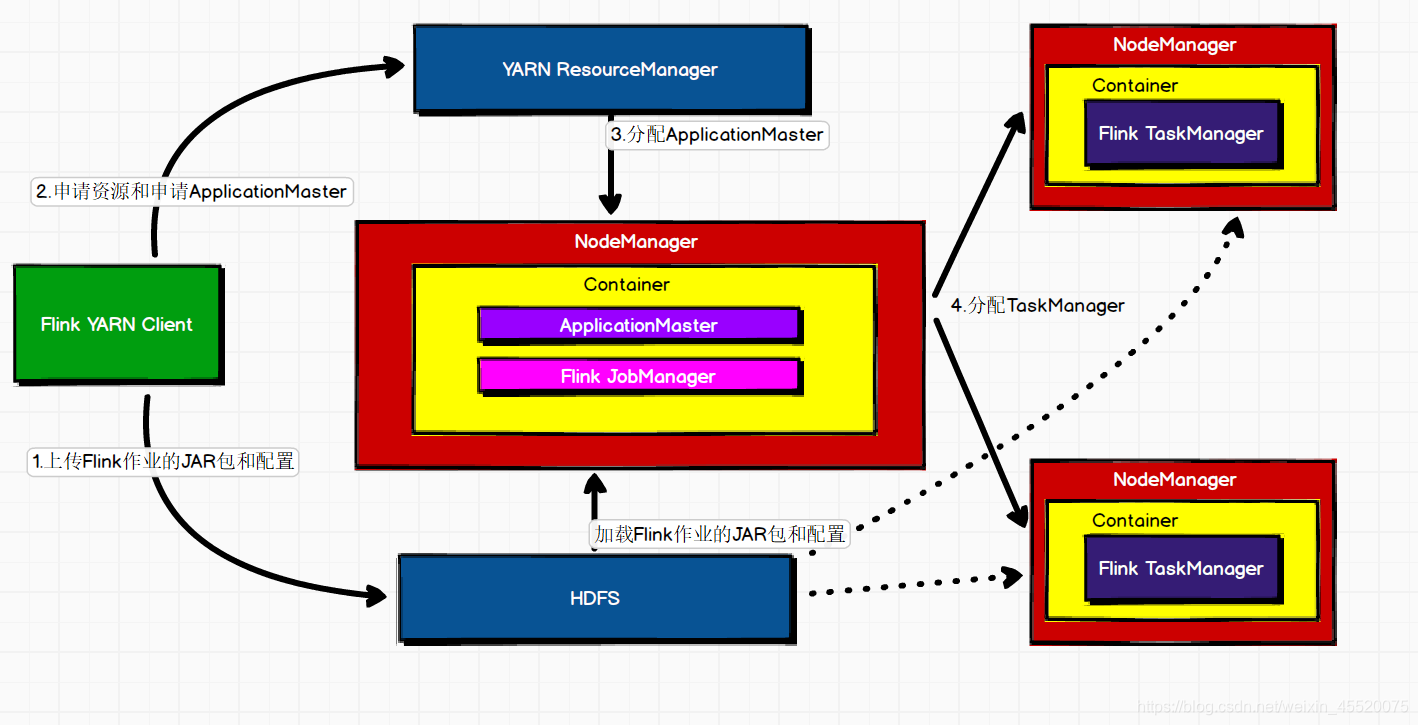
1. 在启动一个新的Flink Yarn会话时,Flink Yarn客户端先检查所请求的资源是否满足可用,可用之后将Flink作业的JAR包和配置上传到HDFS 2. Flink Yarn客户端请求Yarn的ResourceManager去分配容器和通知对应的NodeManager启动ApplicationMaster 3. ApplicationMaster启动后将从HDFS上加载Flink作业的JAR包和配置以启动JobManager,JobManager启动之后ApplicationMaster向ResourceManager申请容器资源以启动TaskManager,ResourceManager分配容器资源以后,由ApplicationMaster通知容器资源所在的NodeManager去启动TaskManager。随后NodeManager将从HDFS上加载Flink作业的JAR包和配置并启动TaskManager,之后TaskManager向JobManager发送心跳,并等待JobManager分配任务。
大家如果喜欢就点赞支持一下,谢谢大家的阅读!
|