一、对执行语句进行调优
1.开启本地模式,对语句执行时间进行优化
set hive.exec.mode.local.auto=true;
2.测试fetch,对语句执行时间进行优化
①set hive.fetch.task.conversion=none;//都转为mr
②set hive.fetch.task.conversion=minimal;//直接获取数据,不 转为mr(但是filter会转化(where子句))
③set hive.fetch.task.conversion=more;//直接查,不走mr(是简单查询,没有函数,没有orderby)
3.执行计划
explain (extended|dependency|authorization) select * from hive_produce;
extended:显示抽象语法树,详细执行计划
dependency:要依赖的输入数据,分为两部分
authorization:这个语句用来表达当前用户对哪些inputs有读操作,对哪些outputs有写操作
4.设置map任务数量
任务数量主要决定因素:input文件总个数,input的文件大小,集群设置的文件块大小
//设置最大切面值为100个字节,观察map任务数
set mapreduce input.fileinputformat split maxsize=100;
5.设置reduce个数
①计算reduece个数 Ⅰ.每个Reduce处理的数据量默认是256MB hive.exec.reducers.bytes.per.reducer=256000000 Ⅱ.每个任务最大的reduce数,默认是1009 hive.exec.reducers.max=1009 Ⅲ.计算reducer数的公式 N=min(参数2,总输入数据量/参数1)
②.调整reduce个数 在Hadoop的mapred-default.xml文件中修改,设置每个job的Reduce数量 set mapreduce.job.reduces=15; ③reduce个数并不是越多越好
有几个reduce就会有几个输入文件,过多的启动和初始化reduce会消耗时间和资源
6.join原则
尽量采用小表join大表 多个表关联时,最好分拆成小段,避免大sql(无法控制中间Job) 大表Join大表的时,主要要考虑空值情况。
7.小文件合并:
默认值 : HiveInputFormat,没有合并,只能文本操作 set hive.input.format =org.apache.hadoop.hive.ql.io.CombineHiveInputFormat;
8. jvm重用:
jvm重用: set mapred.job.reuse.jvm.num.tasks=400;
9. 开启 mapjoin
set hive.auto.convert.join = true;
10.空值处理
- 过滤掉空值( 先判断空值的占比 )
- 生成随机数
二、对文件存储格式进行调优
1.hive文件存储格式
①.textfile
默认格式; 存储方式为行存储; 磁盘开销大 数据解析开销大; 但使用这种方式,hive不会对数据进行切分,从而无法对数据进行并行操作。
②.sequencefile
二进制文件,以<key,value>的形式序列化到文件中; 存储方式:行存储; 可分割 压缩; 一般选择block压缩; 优势是文件和Hadoop api中的mapfile是相互兼容的
③.refile
存储方式:数据按行分块 每块按照列存储; 压缩快 快速列存取; 读记录尽量涉及到的block最少; 读取需要的列只需要读取每个row group 的头部定义; 读取全量数据的操作 性能可能比sequencefile没有明显的优势,
④.orcfile
存储方式:数据按行分块 每块按照列存储; 压缩快 快速列存取; 效率比rcfile高,是rcfile的改良版本。
⑤.parquet
类似于orc,相对于orc文件格式,hadoop生态系统中大部分工程都支持parquet文件。
2.hive文件存储格式和压缩方式进行测试
测试一: 只测格式 文件大小
- textfile
create table if not exists sheet1_textfile( id int, cname string, age double, pname string, sex string, nationality string, workyear double, diploma string, zhiyezige string, position string, agerange string, type string, positiontype string, professional string )row format delimited fields terminated by “\t” stored as textfile;
load data local inpath ‘/tmp/Sheet1.txt’ into table sheet1_textfile;
select count(*) from sheet1_textfile;
hadoop dfs -du -h /user/hive/warehouse/sheet1_textfile;
–210.2k
- sequencefile
create table if not exists sheet1_sequencefile( id int, cname string, age double, pname string, sex string, nationality string, workyear double, diploma string, zhiyezige string, position string, agerange string, type string, positiontype string, professional string )row format delimited fields terminated by “\t” stored as sequencefile;
–load data local inpath ‘/tmp/Sheet1.txt’ into table sheet1_sequencefile; insert into sheet1_sequencefile select * from sheet1_textfile;
select count(*) from sheet1_sequencefile;
hadoop dfs -du -h /user/hive/warehouse/sheet1_sequencefile;
—232K
- rcfile
create table if not exists sheet1_rcfile( id int, cname string, age double, pname string, sex string, nationality string, workyear double, diploma string, zhiyezige string, position string, agerange string, type string, positiontype string, professional string )row format delimited fields terminated by “\t” stored as rcfile;
–load data local inpath ‘/tmp/Sheet1.txt’ into table sheet1_rcfile; --不能load,因为原文件是 /tmp/Sheet1.txt是文本文件,不是 rcfile,所以不能直接load. insert into sheet1_rcfile select * from sheet1_textfile;
select count(*) from sheet1_rcfile;
hadoop dfs -du -h /user/hive/warehouse/sheet1_rcfile; –199.2k 4. parquet create table if not exists sheet1_parquet( id int, cname string, age double, pname string, sex string, nationality string, workyear double, diploma string, zhiyezige string, position string, agerange string, type string, positiontype string, professional string )row format delimited fields terminated by “\t” stored as parquet;
insert into sheet1_parquet select * from sheet1_textfile;
select count(*) from sheet1_parquet;
hadoop dfs -du -h /user/hive/warehouse/sheet1_parquet; –39k
-
orcfile create table if not exists sheet1_orc( id int, cname string, age double, pname string, sex string, nationality string, workyear double, diploma string, zhiyezige string, position string, agerange string, type string, positiontype string, professional string )row format delimited fields terminated by “\t” stored as orc
insert into sheet1_orc select * from sheet1_textfile;
select count(*) from sheet1_orc;
hadoop dfs -du -h /user/hive/warehouse/sheet1_orc;
测试二: 结合压缩 文件大小.
- ZLIB压缩的ORC存储方式
create table if not exists sheet1_orc_zlib( id int, cname string, age double, pname string, sex string, nationality string, workyear double, diploma string, zhiyezige string, position string, agerange string, type string, positiontype string, professional string )row format delimited fields terminated by “\t” stored as orc tblproperties(“orc.compress”=“ZLIB”);
insert into sheet1_orc_zlib select * from sheet1_textfile;
select count(*) from sheet1_orc_zlib;
hadoop dfs -du -h /user/hive/warehouse/sheet1_orc_zlib;
–21.2k
- snappy 压缩的ORC存储方式
create table if not exists sheet1_orc_snappy( id int, cname string, age double, pname string, sex string, nationality string, workyear double, diploma string, zhiyezige string, position string, agerange string, type string, positiontype string, professional string )row format delimited fields terminated by “\t” stored as orc tblproperties(“orc.compress”=“snappy”);
insert into sheet1_orc_snappy select * from sheet1_textfile;
select count(*) from sheet1_orc_snappy;
hadoop dfs -du -h /user/hive/warehouse/sheet1_orc_snappy;
–30.8k
- SNAPPY压缩的parquet存储方式
create table if not exists sheet1_parquet_snappy( id int, cname string, age double, pname string, sex string, nationality string, workyear double, diploma string, zhiyezige string, position string, agerange string, type string, positiontype string, professional string )row format delimited fields terminated by “\t” stored as parquet tblproperties(“orc.compress”=“snappy”);
insert into sheet1_parquet_snappy select * from sheet1_textfile;
select count(*) from sheet1_parquet_snappy;
hadoop dfs -du -h /user/hive/warehouse/sheet1_parquet_snappy;
–39k
3.总结
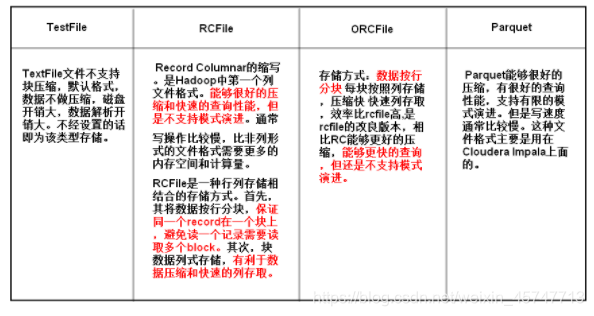 
|