一、MapReduce
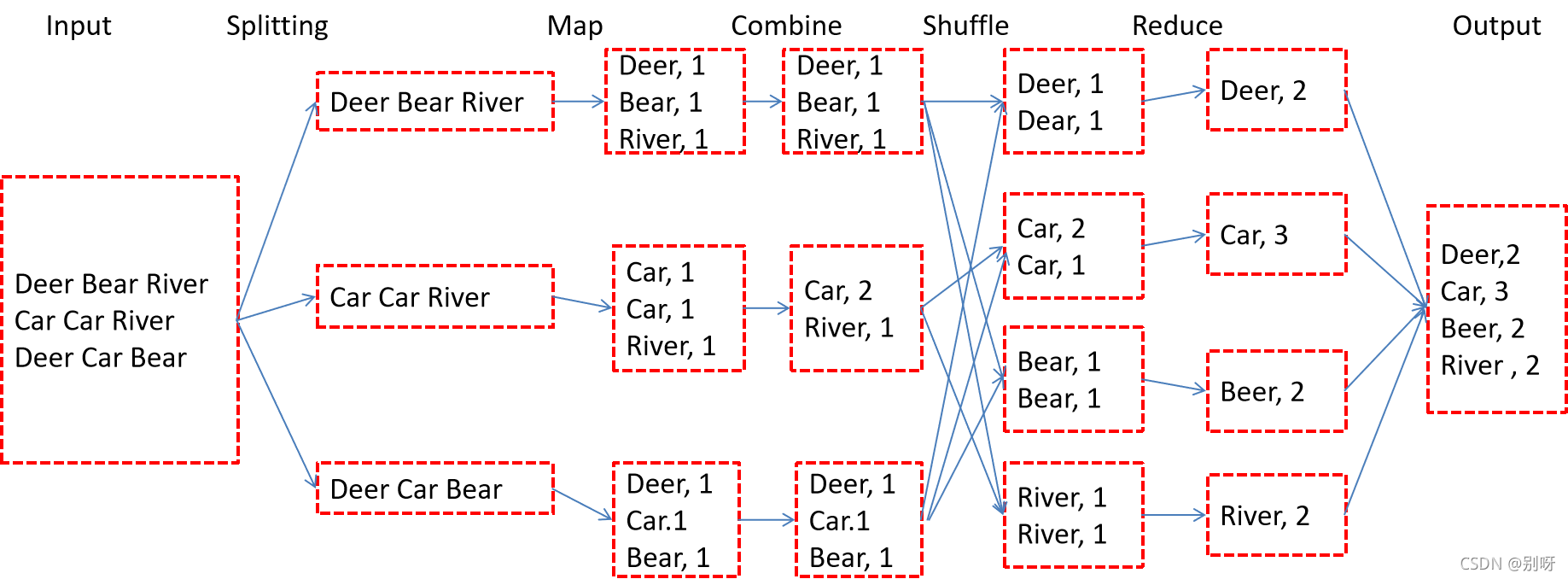 MapReduce是Google提出的一个软件架构,用于大规模数据集(大于1TB)的并行运算。概念“Map(映射)”和“Reduce(归纳)”,及他们的主要思想,都是从函数式编程语言借来的,还有从矢量编程语言借来的特性。
当前的软件实现是指定一个Map(映射)函数,用来把一组键值对映射成一组新的键值对,指定并发的Reduce(归纳)函数,用来保证所有映射的键值对中的每一个共享相同的键组。
二、MapReduce开发环境搭建
环境准备: Java, Intellij IDEA, Maven 开发环境搭建方式
java安装链接及步骤:https://www.cnblogs.com/de-ming/p/13909440.html
2.1、Maven环境
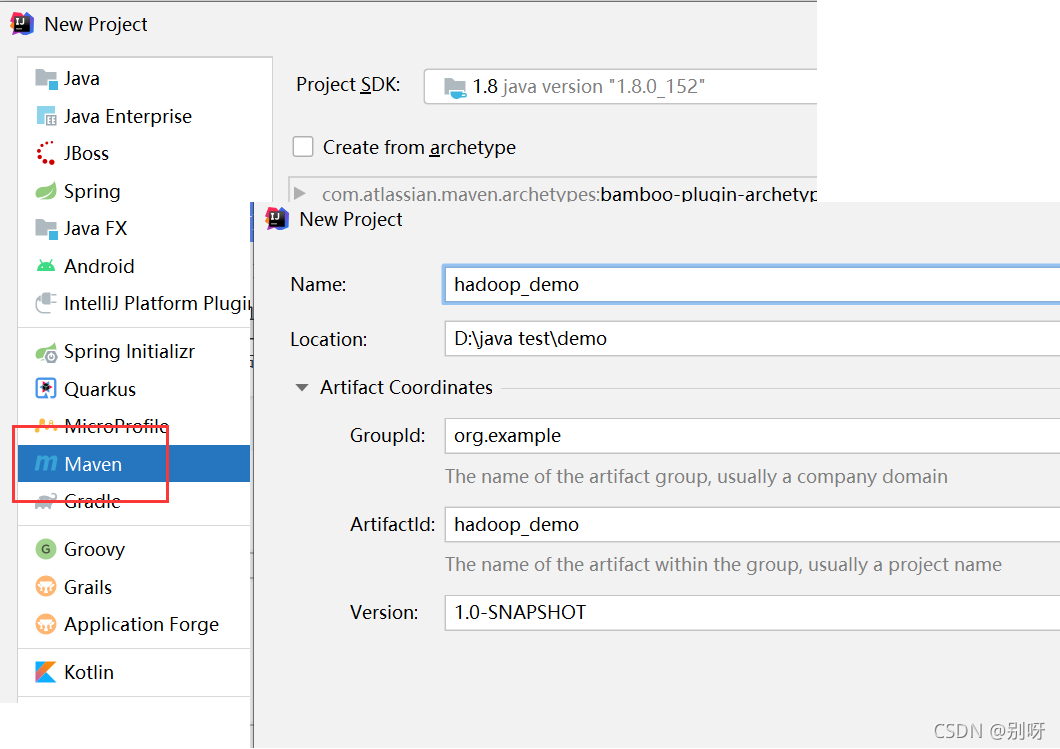 添加依赖
https://search.maven.org/artifact/org.apache.hadoop/hadoop-client/3.1.4/jar
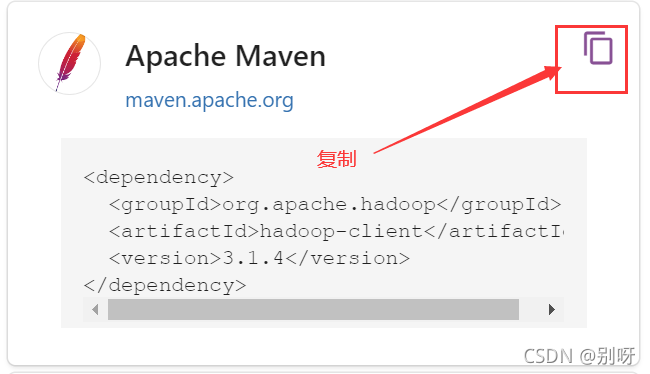
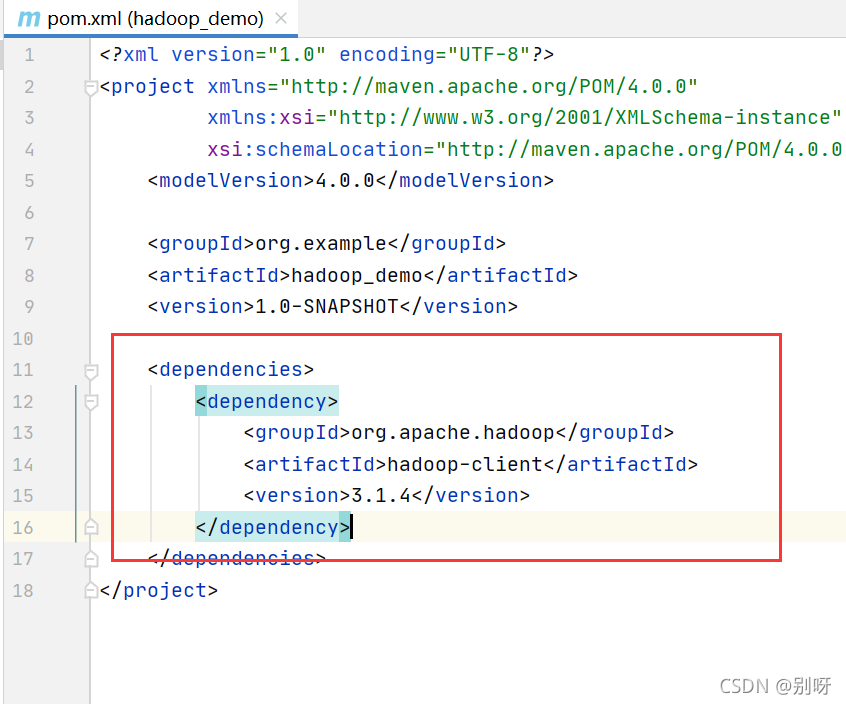
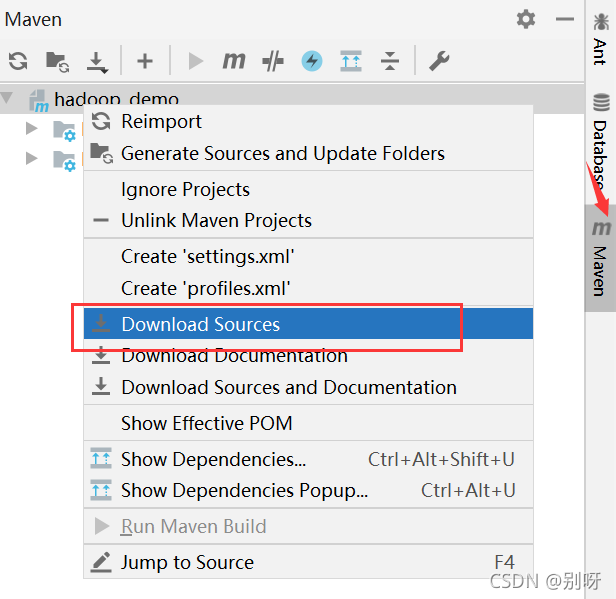 添加源码 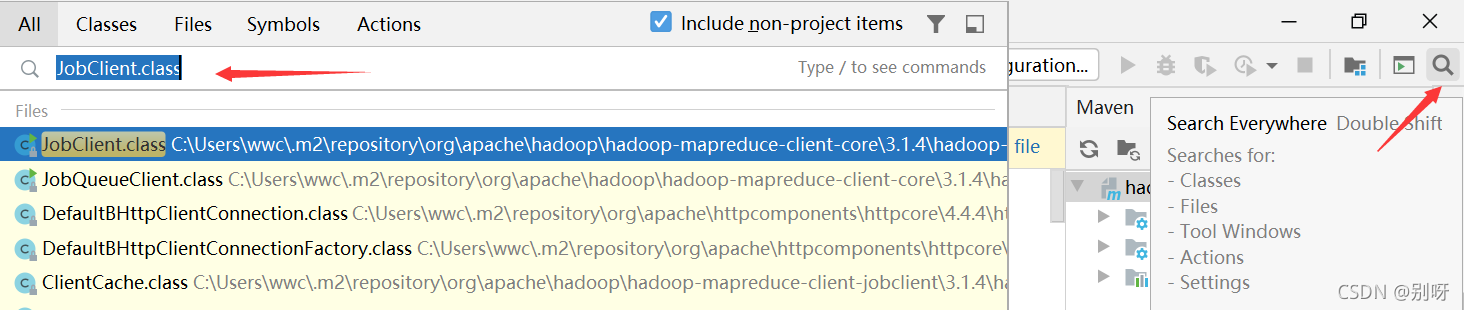 
2.2、手动导入Jar包
Hadoop安装包链接:https://pan.baidu.com/s/1teHwnBH2Qm6F7iWZ3q-hSQ 提取码:cgnb
新建一个java工程 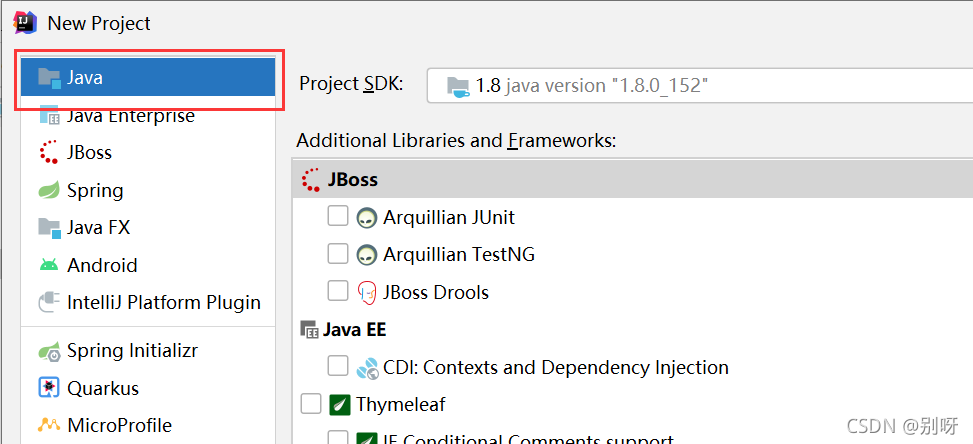 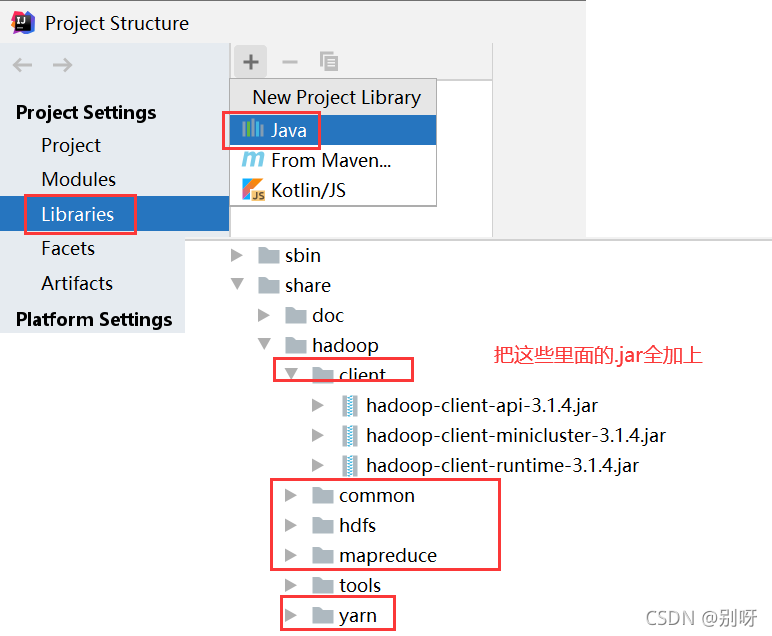 然后,搜JobClient.class,点击’Choose Sources’ 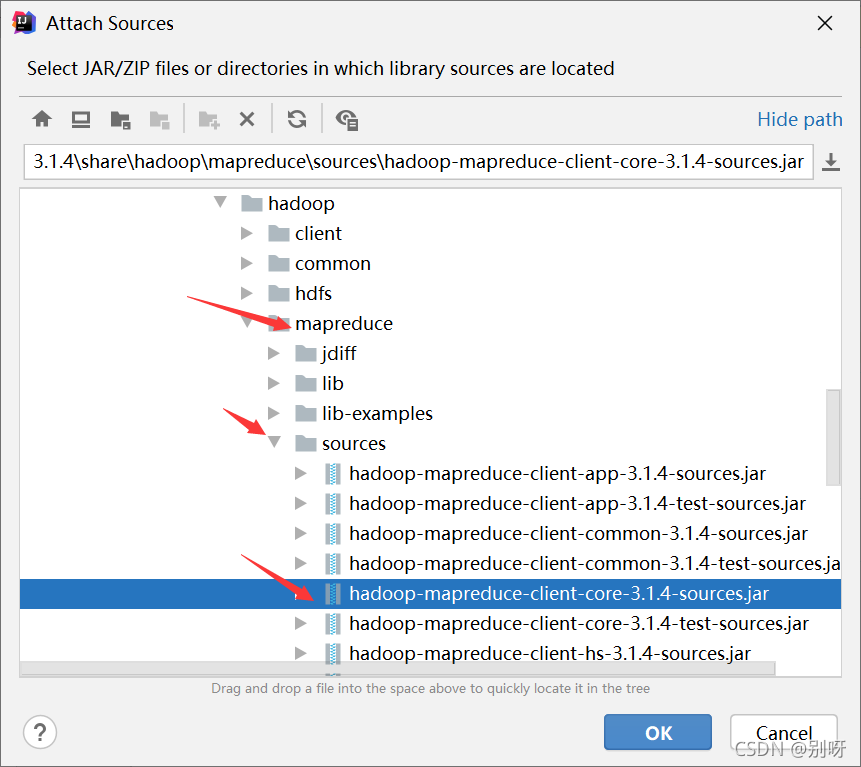
这样就OK了,可以看到JobClient.java
三、MapReduce单词计数源码分析
3.1、打开WordCount.java
打开:https://mvnrepository.com/artifact/org.apache.hadoop/hadoop-mapreduce-examples/3.1.4,复制Maven里面的内容 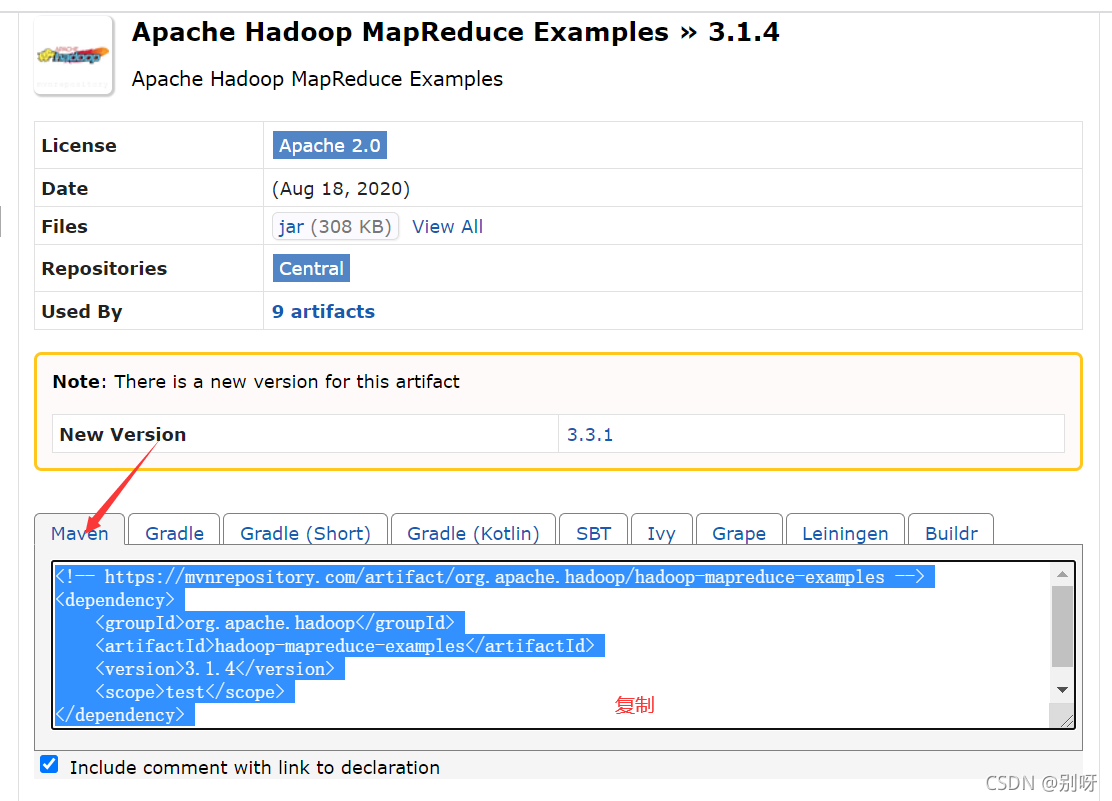 粘贴到源码 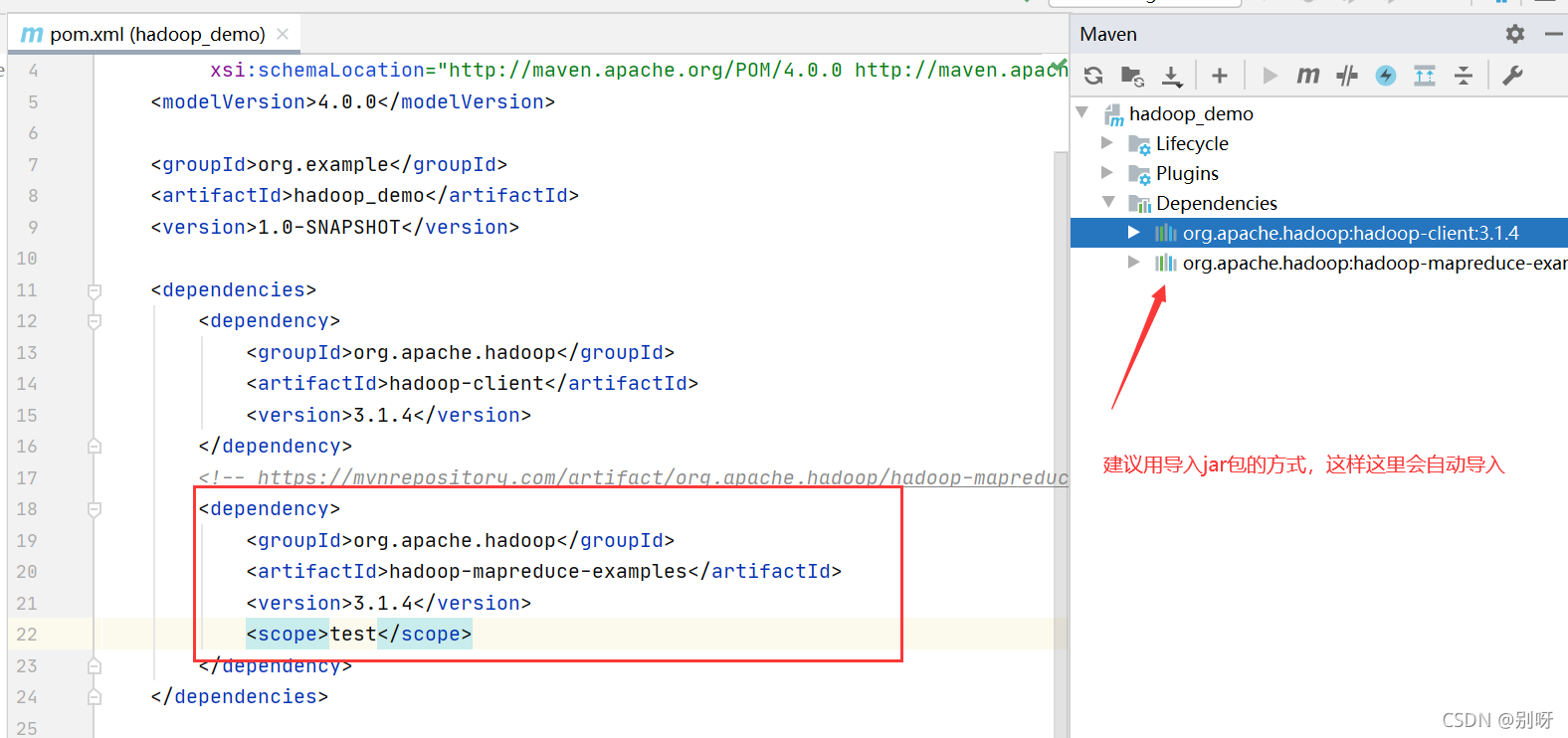 搜索WordCount 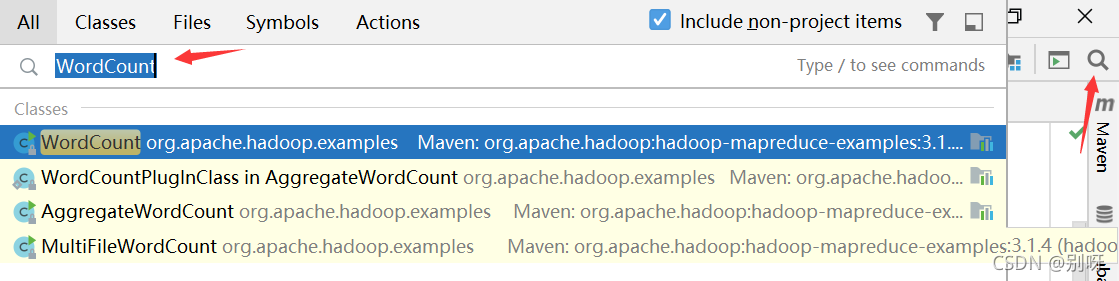
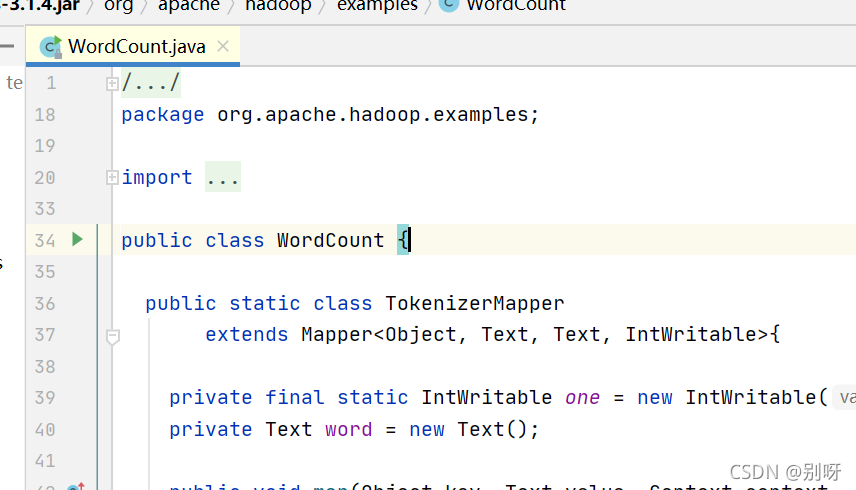
3.2、源码分析
3.2.1、MapReduce单词计数源码 : Map任务
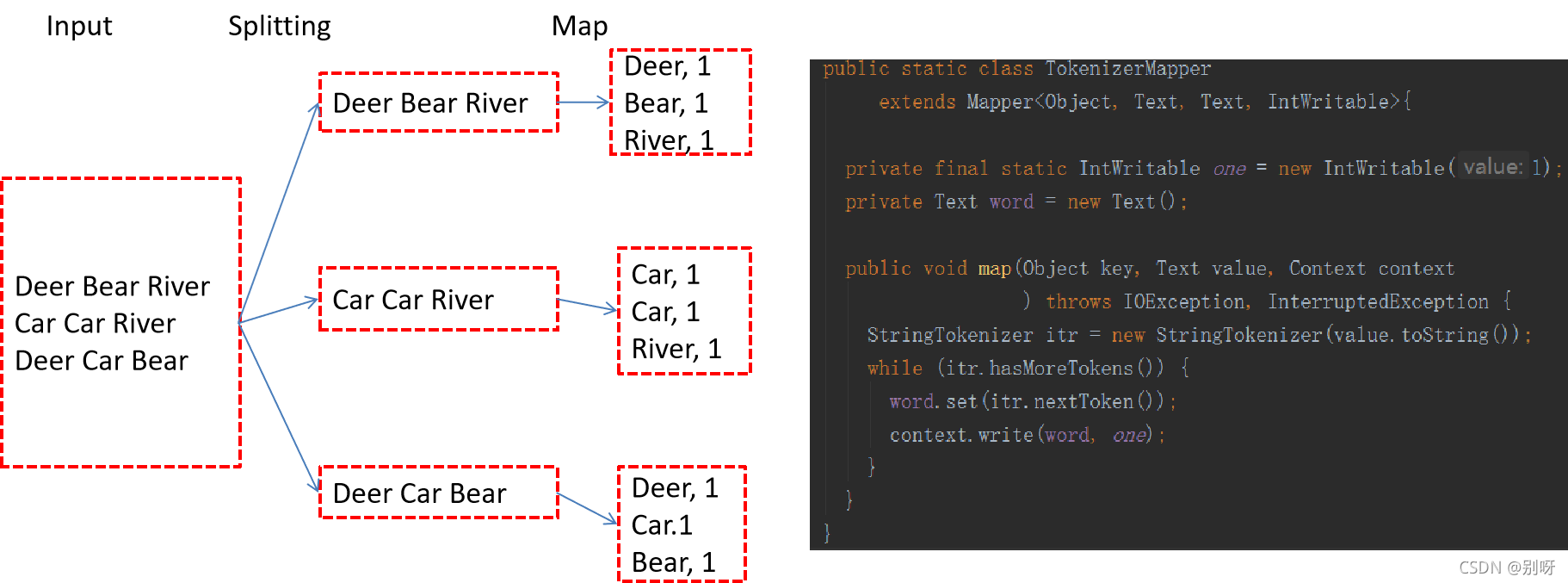
3.2.2、MapReduce单词计数源码 : Reduce任务
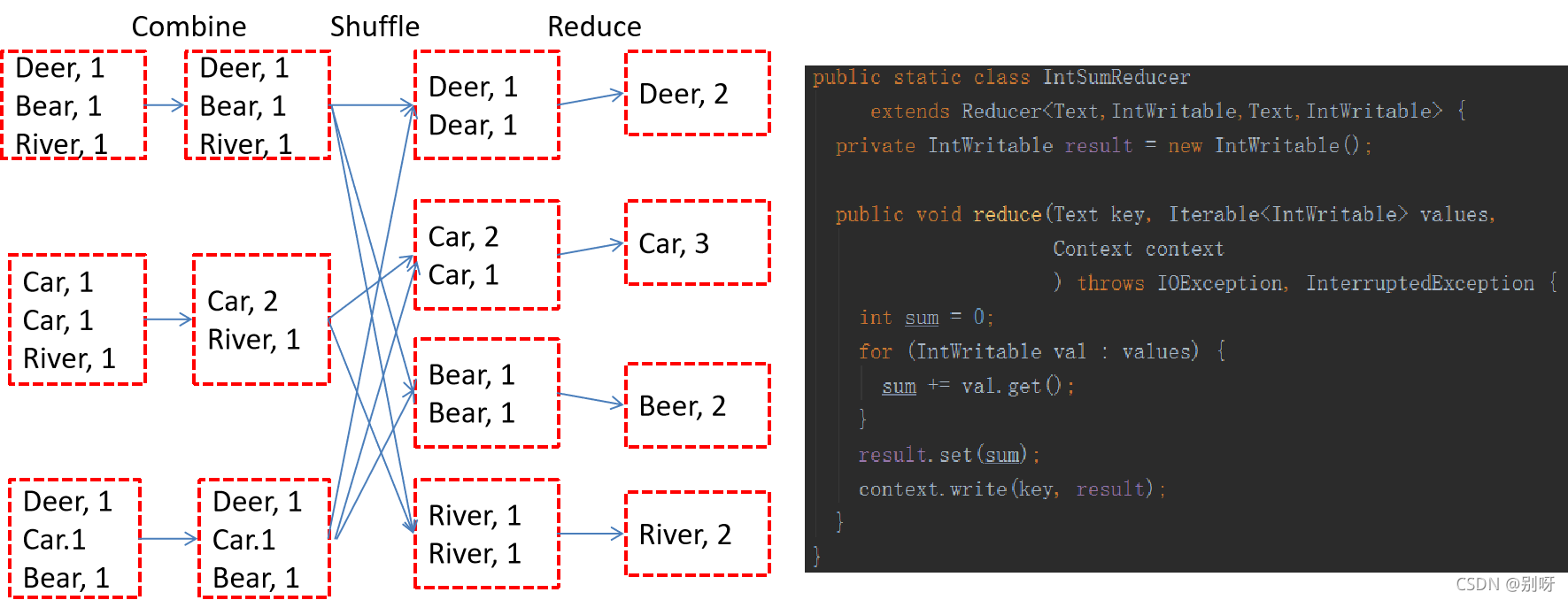
3.2.3、MapReduce单词计数源码 : main 函数
设置必要参数及组装MapReduce程序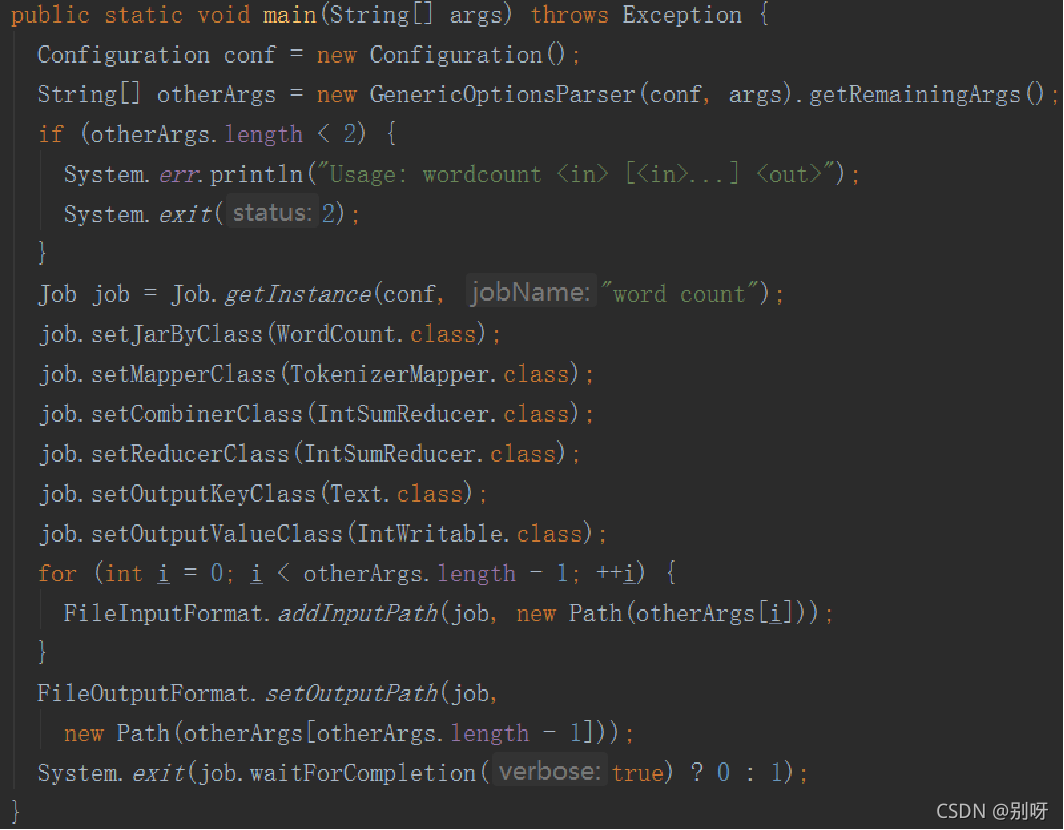
四、MapReduce API介绍
- 一般MapReduce都是由Mapper, Reducer 及main 函数组成。
- Mapper程序一般完成键值对映射操作;
- Reducer 程序一般完成键值对聚合操作;
- Main函数则负责组装Mapper,Reducer及必要的配置;
- 高阶编程还涉及到设置输入输出文件格式、设置Combiner、Partitioner优化程序等;
4.1、MapReduce程序模块 : Main 函数
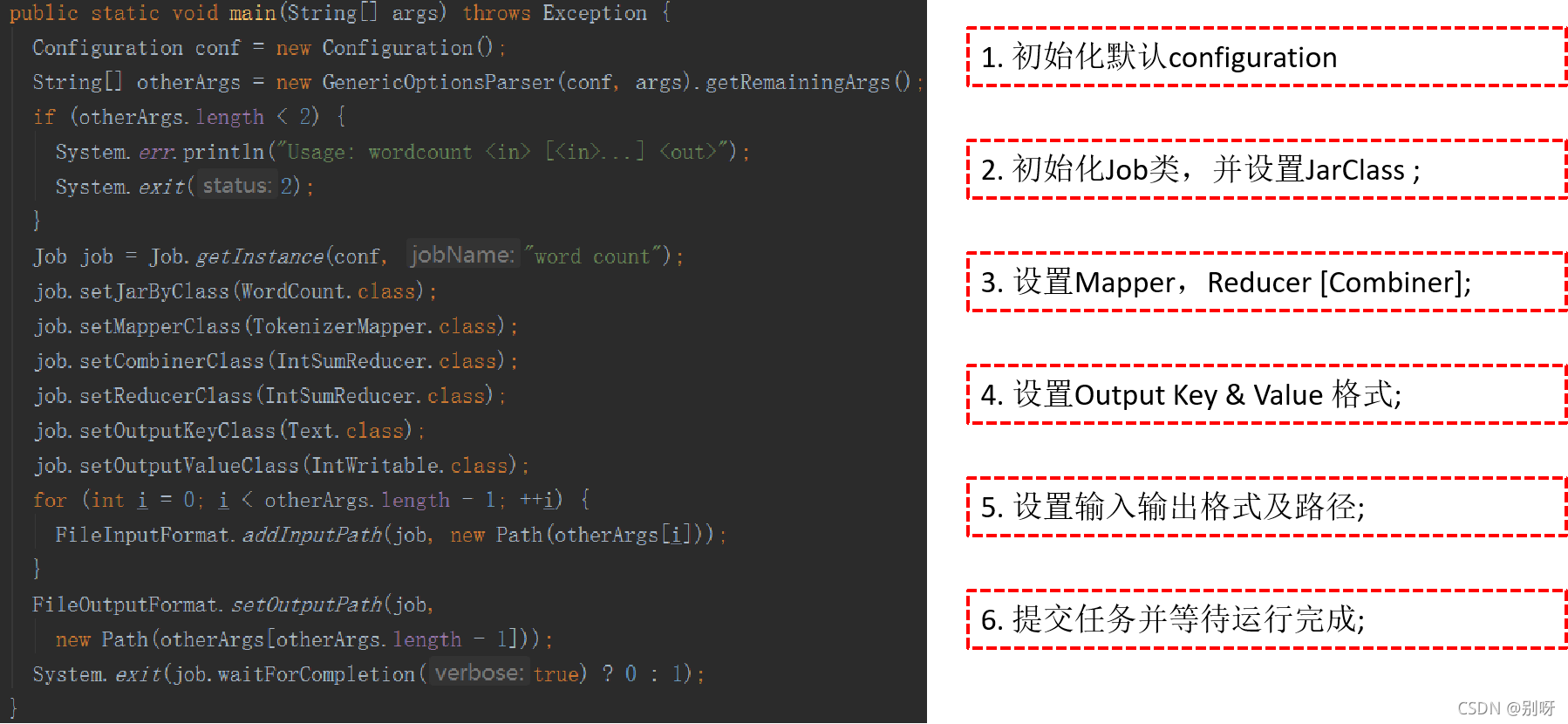
4.2、MapReduce程序模块: Mapper
- org.apache.hadoop.mapreduce.Mapper
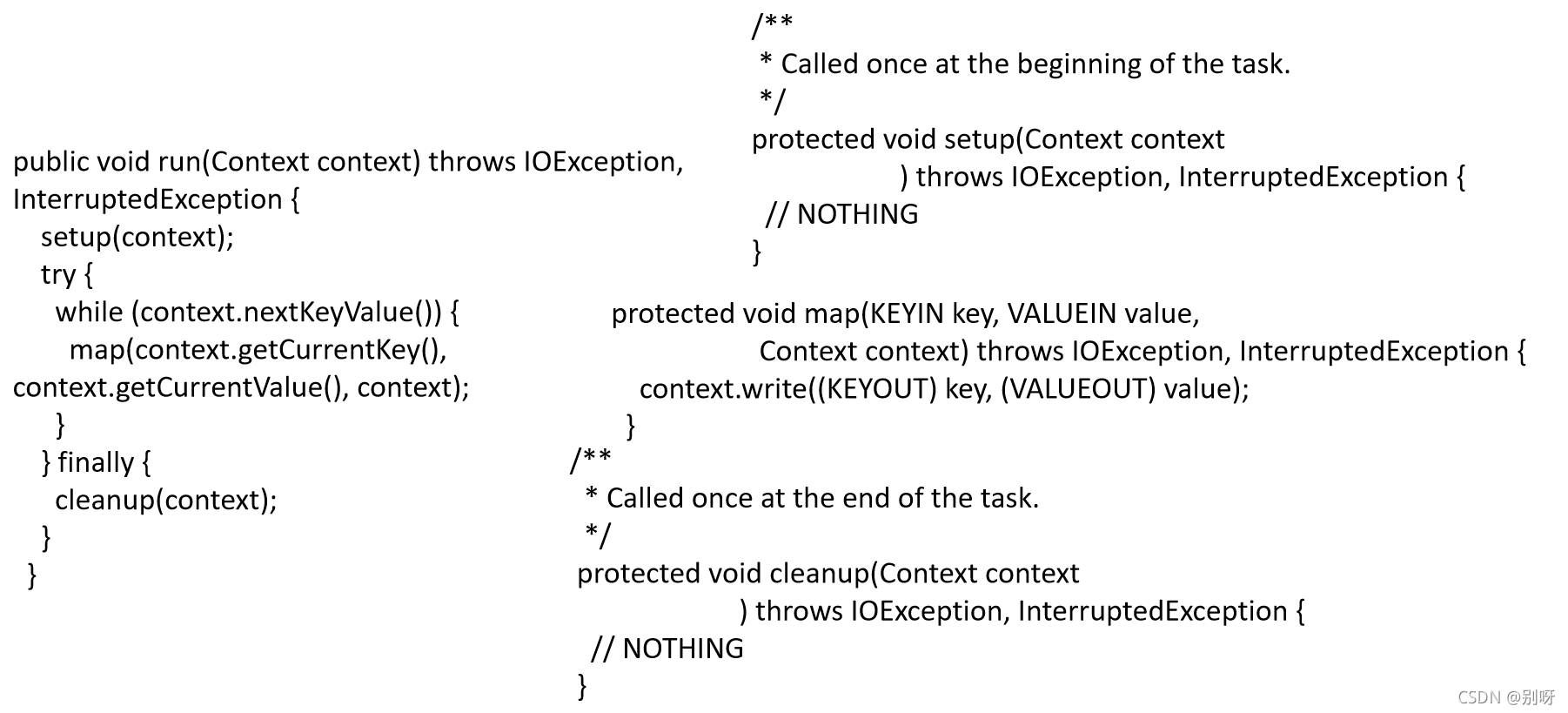
4.3、MapReduce程序模块: Reducer
- org.apache.hadoop.mapreduce.Reducer
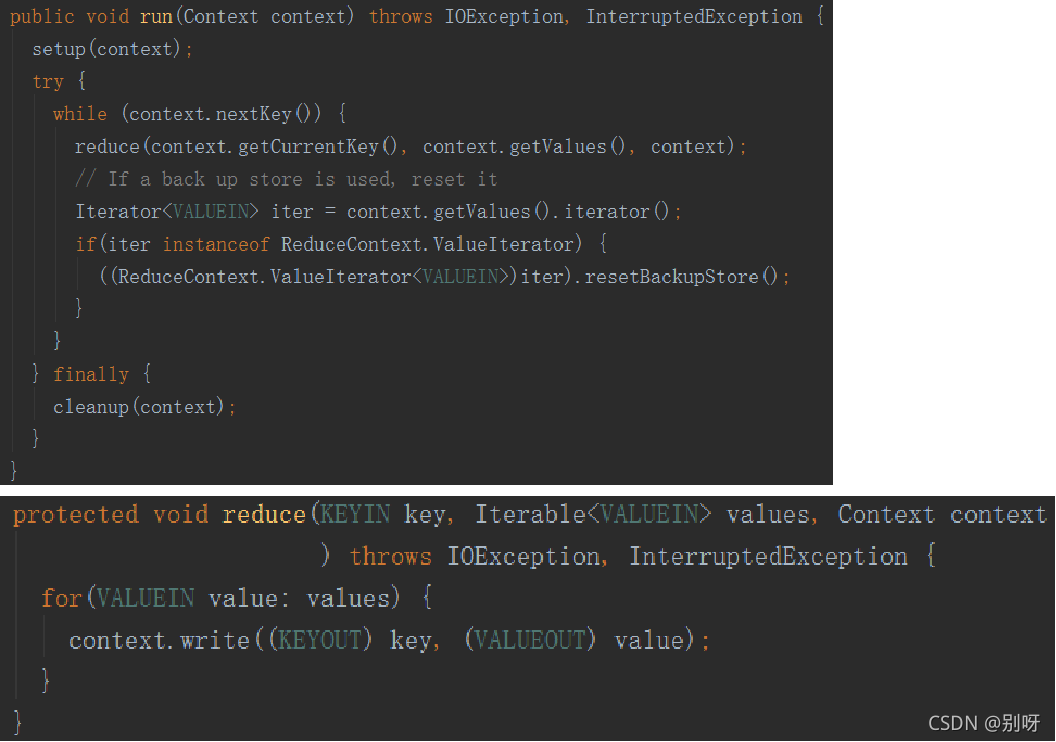
五、MapReduce实例
5.1、流程(Mapper、Reducer、Main、打包运行)
- 参考WordCount程序,修改Mapper;
- 直接复制 Reducer程序;
- 直接复制Main函数,并做相应修改;
- 编译打包 ;
- 上传Jar包;
- 上传数据;
- 运行程序;
- 查看运行结果;
5.2、实例1:按日期访问统计次数:
1、参考WordCount程序,修改Mapper; (这里新建一个java程序,然后把下面(1、2、3步代码)复制到类里)
public static class SpiltMapper
extends Mapper<Object, Text, Text, IntWritable> {
private final static IntWritable one = new IntWritable(1);
private Text word = new Text();
public void map(Object key, Text value, Context context
) throws IOException, InterruptedException {
String[] data = value.toString().split("\\|",-1);
word.set(data[1]);
context.write(word, one);
}
}
2、直接复制 Reducer程序;
public static class IntSumReducer
extends Reducer<Text,IntWritable,Text,IntWritable> {
private IntWritable result = new IntWritable();
public void reduce(Text key, Iterable<IntWritable> values,
Context context
) throws IOException, InterruptedException {
int sum = 0;
for (IntWritable val : values) {
sum += val.get();
}
result.set(sum);
context.write(key, result);
}
}
3、直接复制Main函数,并做相应修改;
public static void main(String[] args) throws Exception {
Configuration conf = new Configuration();
String[] otherArgs = new GenericOptionsParser(conf, args).getRemainingArgs();
if (otherArgs.length < 2) {
System.err.println("Usage: wordcount <in> [<in>...] <out>");
System.exit(2);
}
Job job = Job.getInstance(conf, "word count");
job.setJarByClass(CountByDate.class);
job.setMapperClass(SpiltMapper.class);
job.setCombinerClass(IntSumReducer.class);
job.setReducerClass(IntSumReducer.class);
job.setOutputKeyClass(Text.class);
job.setOutputValueClass(IntWritable.class);
for (int i = 0; i < otherArgs.length - 1; ++i) {
FileInputFormat.addInputPath(job, new Path(otherArgs[i]));
}
FileOutputFormat.setOutputPath(job,
new Path(otherArgs[otherArgs.length - 1]));
System.exit(job.waitForCompletion(true) ? 0 : 1);
}
4、编译打包 (jar打包)
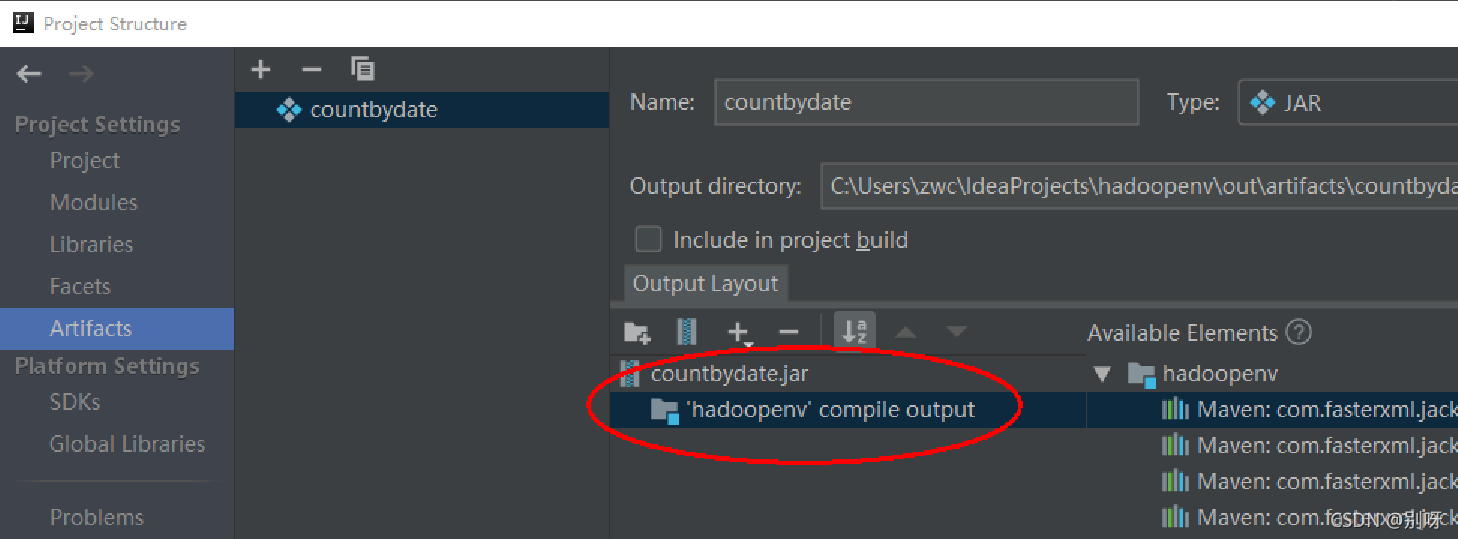 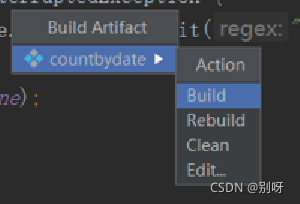
build出现错误及解决办法: 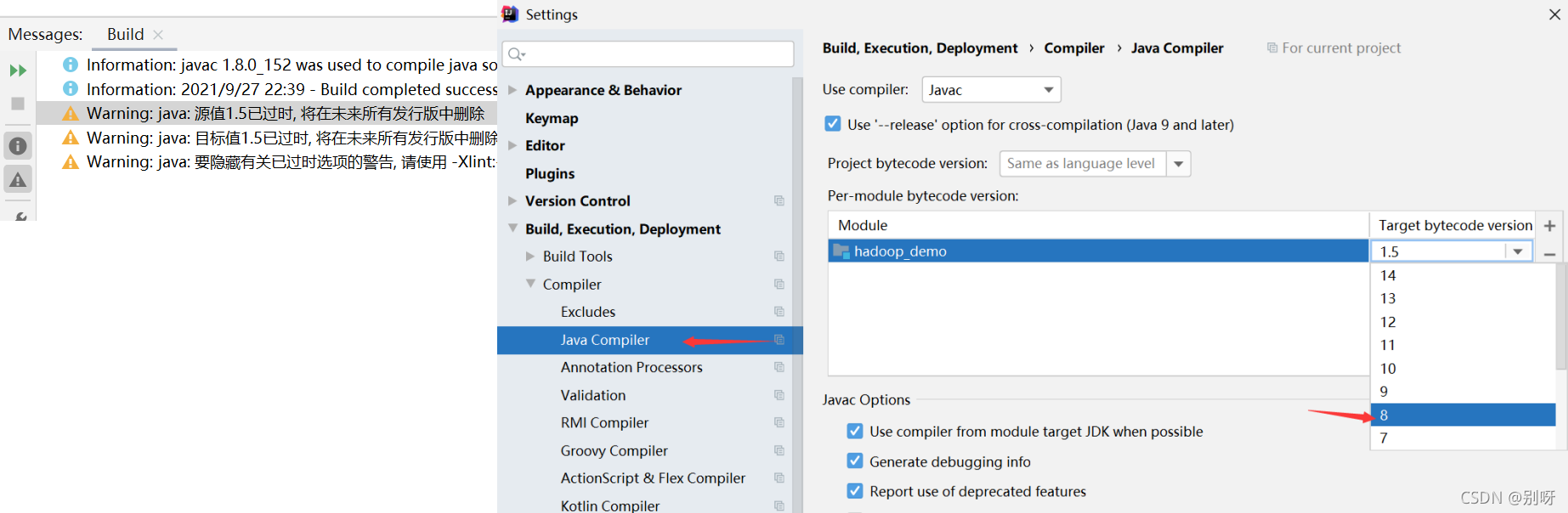 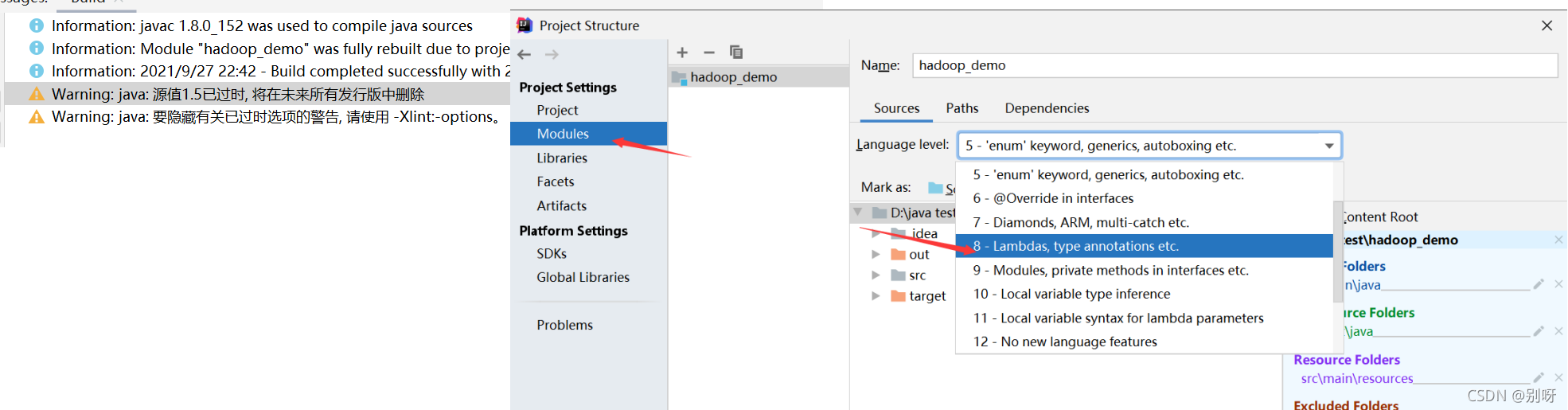
完成 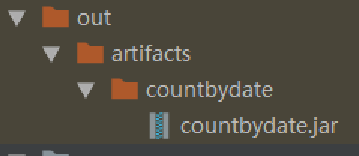
5/6、上传jar包&数据 email_log_with_date.txt数据包链接:https://pan.baidu.com/s/1HfwHCfmvVdQpuL-MPtpAng 提取码:cgnb 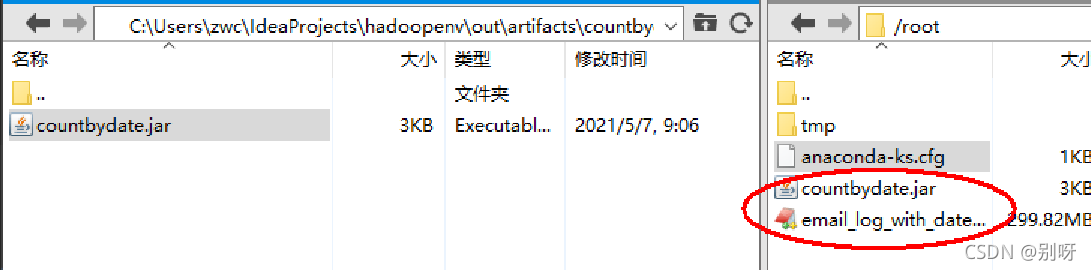 上传数据包(注意开启hdfs):  上传OK(浏览器:master:50070 查看) 
7、运行程序 (注意开启yarn)  上传完成后:
(master:8088 )
 8、查看结果 (master:50070 ) 
5.3、实例2:按用户访问次数排序
Mapper、Reducer、Main程序 SortByCountFirst.Mapper
package demo;
import org.apache.hadoop.conf.Configuration;
import org.apache.hadoop.fs.Path;
import org.apache.hadoop.io.IntWritable;
import org.apache.hadoop.io.Text;
import org.apache.hadoop.mapreduce.Job;
import org.apache.hadoop.mapreduce.Mapper;
import org.apache.hadoop.mapreduce.Reducer;
import org.apache.hadoop.mapreduce.lib.input.FileInputFormat;
import org.apache.hadoop.mapreduce.lib.output.FileOutputFormat;
import org.apache.hadoop.util.GenericOptionsParser;
import java.io.IOException;
public class SortByCountFirst {
public static class SpiltMapper
extends Mapper<Object, Text, Text, IntWritable> {
private final static IntWritable one = new IntWritable(1);
private Text word = new Text();
public void map(Object key, Text value, Context context
) throws IOException, InterruptedException {
String[] data = value.toString().split("\\|",-1);
word.set(data[0]);
context.write(word, one);
}
}
public static class IntSumReducer
extends Reducer<Text,IntWritable,Text,IntWritable> {
private IntWritable result = new IntWritable();
public void reduce(Text key, Iterable<IntWritable> values,
Context context
) throws IOException, InterruptedException {
int sum = 0;
for (IntWritable val : values) {
sum += val.get();
}
result.set(sum);
context.write(key, result);
}
}
public static void main(String[] args) throws Exception {
Configuration conf = new Configuration();
String[] otherArgs = new GenericOptionsParser(conf, args).getRemainingArgs();
if (otherArgs.length < 2) {
System.err.println("Usage: demo.SortByCountFirst <in> [<in>...] <out>");
System.exit(2);
}
Job job = Job.getInstance(conf, "sort by count first ");
job.setJarByClass(SortByCountFirst.class);
job.setMapperClass(SpiltMapper.class);
job.setCombinerClass(IntSumReducer.class);
job.setReducerClass(IntSumReducer.class);
job.setOutputKeyClass(Text.class);
job.setOutputValueClass(IntWritable.class);
for (int i = 0; i < otherArgs.length - 1; ++i) {
FileInputFormat.addInputPath(job, new Path(otherArgs[i]));
}
FileOutputFormat.setOutputPath(job,
new Path(otherArgs[otherArgs.length - 1]));
System.exit(job.waitForCompletion(true) ? 0 : 1);
}
}
SortByCountSecond.Mapper
package demo;
import org.apache.hadoop.conf.Configuration;
import org.apache.hadoop.fs.Path;
import org.apache.hadoop.io.IntWritable;
import org.apache.hadoop.io.Text;
import org.apache.hadoop.mapreduce.Job;
import org.apache.hadoop.mapreduce.Mapper;
import org.apache.hadoop.mapreduce.Reducer;
import org.apache.hadoop.mapreduce.lib.input.FileInputFormat;
import org.apache.hadoop.mapreduce.lib.output.FileOutputFormat;
import org.apache.hadoop.util.GenericOptionsParser;
import java.io.IOException;
public class SortByCountSecond {
public static class SpiltMapper
extends Mapper<Object, Text, IntWritable, Text> {
private IntWritable count = new IntWritable(1);
private Text word = new Text();
public void map(Object key, Text value, Context context
) throws IOException, InterruptedException {
String[] data = value.toString().split("\t",-1);
word.set(data[0]);
count.set(Integer.parseInt(data[1]));
context.write(count,word);
}
}
public static class ReverseReducer
extends Reducer<IntWritable,Text,Text,IntWritable> {
public void reduce(IntWritable key, Iterable<Text> values,
Context context
) throws IOException, InterruptedException {
for (Text val : values) {
context.write(val,key);
}
}
}
public static void main(String[] args) throws Exception {
Configuration conf = new Configuration();
String[] otherArgs = new GenericOptionsParser(conf, args).getRemainingArgs();
if (otherArgs.length < 2) {
System.err.println("Usage: demo.SortByCountFirst <in> [<in>...] <out>");
System.exit(2);
}
Job job = Job.getInstance(conf, "sort by count first ");
job.setJarByClass(SortByCountSecond.class);
job.setMapperClass(SpiltMapper.class);
job.setReducerClass(ReverseReducer.class);
job.setMapOutputKeyClass(IntWritable.class);
job.setMapOutputValueClass(Text.class);
job.setOutputKeyClass(Text.class);
job.setOutputValueClass(IntWritable.class);
for (int i = 0; i < otherArgs.length - 1; ++i) {
FileInputFormat.addInputPath(job, new Path(otherArgs[i]));
}
FileOutputFormat.setOutputPath(job,
new Path(otherArgs[otherArgs.length - 1]));
System.exit(job.waitForCompletion(true) ? 0 : 1);
}
}
然后打包上传
yarn jar sortbycount.jar demo.SortByCountSecond -Dmapreduce.job.queuename=prod email_log_with_date.txt sortbycountfirst_output00
yarn jar sortbycount.jar demo.SortByCountSecond -Dmapreduce.job.queuename=prod email_log_with_date.txt sortbycountfirst_output00 sortbycountsecond_output00
|