基于windows已配置号hadoop客户端,本次使用的事hadoop3.1.3版本
数据:
As soon as an abnormally elevated IOP was encountered, patient was excluded from the study
and the prior regimen was reestablished. RESULTS This study included 53 eyes of 53
patients with open angle glaucoma. Twenty-seven patients suffered from primary open
angle glaucoma and 26 patients had pseudoexfoliative glaucoma. After beginning the
second phase of the study, a mild trend of increasing IOP was recordable. A corresponding
trend was even detected in female and male patients separately. The P values at week
In the first 2 weeks after initiation of the 2nd phase, 66% of cases have no change
in IOPs, but thereafter, 69.8%, experienced increasing IOPs.The present
study shows the superiority of the conventional dosage of Latanoprost 0.005% in
comparison with once every other day dose but at least in first few weeks, the
IOPs are reasonably close to each other. Further studies with higher number of
cases would widen the present findings.
#maper代码
package com.pw.study.wordcount;
import org.apache.hadoop.io.IntWritable;
import org.apache.hadoop.io.LongWritable;
import org.apache.hadoop.io.Text;
import org.apache.hadoop.mapreduce.Mapper;
import java.io.IOException;
public class WCMapper extends Mapper<LongWritable, Text, Text, IntWritable> {
private final Text outKey = new Text();
private final IntWritable outValue = new IntWritable();
@Override
protected void map(LongWritable key, Text value, Context context) throws IOException, InterruptedException {
//1.读取一行
String line = value.toString();
//2.切割
String[] values = line.split(" ");
for (String item : values) {
//每一个单词记录一下
outKey.set(item);
outValue.set(1);
context.write(outKey, outValue);
}
}
}
#reducer代码
package com.pw.study.wordcount;
import org.apache.hadoop.io.IntWritable;
import org.apache.hadoop.io.Text;
import org.apache.hadoop.mapreduce.Reducer;
import java.io.IOException;
public class WCReducer extends Reducer<Text, IntWritable, Text, IntWritable> {
//输出内容
private final IntWritable outValue = new IntWritable();
@Override
protected void reduce(Text key, Iterable<IntWritable> values, Context context) throws IOException, InterruptedException {
//累计求和
int sum = 0;
for (IntWritable value : values) {
sum += value.get();
}
outValue.set(sum);
context.write(key, outValue);
}
}
# wcDriver 主类,本地直接启动(本地运行)得到结果
import java.io.IOException;
public class WCDriver {
public static void main(String[] args) throws IOException, ClassNotFoundException, InterruptedException {
// 启动mrJob
Job job = Job.getInstance(new Configuration());
//设置主启动类
/*设置map实现类*/
job.setMapperClass(WCMapper.class);
/*设置reduce实现类*/
job.setReducerClass(WCReducer.class);
//设置map输出key类型
job.setMapOutputKeyClass(Text.class);
//设置map输出value类型
job.setMapOutputValueClass(IntWritable.class);
//设置最终输出key
job.setOutputKeyClass(Text.class);
//设置最终输出value
job.setOutputValueClass(IntWritable.class);
//文件输入
FileInputFormat.setInputPaths(job, new Path("E:\\work\\note\\iodata\\input"));
//最终结果输出
FileOutputFormat.setOutputPath(job, new Path("E:\\work\\note\\iodata\\out3"));
//执行job
job.waitForCompletion(true);
}
}
#hdfs运行,在本地将Wordcount项目打包,将jar上传到hdfs的节点上,并将输入文件上传到/input中。然后运行如下代码,由于我打包时没有你配置主类所以包名一起输入,其他的和官网的一致,
hadoop jar./MR_WC.jar com.pw.study.wordcount.WCDriverForHDFSAndSingle /input /output
package com.pw.study.wordcount;
import org.apache.hadoop.conf.Configuration;
import org.apache.hadoop.fs.Path;
import org.apache.hadoop.io.IntWritable;
import org.apache.hadoop.io.Text;
import org.apache.hadoop.mapreduce.Job;
import org.apache.hadoop.mapreduce.lib.input.FileInputFormat;
import org.apache.hadoop.mapreduce.lib.output.FileOutputFormat;
import java.io.IOException;
/**
* hdfs 单机运行
* 设置主启类
* 2.将文件路劲输入修改为入参
*/
public class WCDriverForHDFSAndSingle {
public static void main(String[] args) throws IOException, ClassNotFoundException, InterruptedException {
// 启动mrJob
Job job = Job.getInstance(new Configuration());
//设置主启动类
job.setJarByClass(WCDriverForHDFSAndSingle.class);
/*设置map实现类*/
job.setMapperClass(WCMapper.class);
/*设置reduce实现类*/
job.setReducerClass(WCReducer.class);
//设置map输出key类型
job.setMapOutputKeyClass(Text.class);
//设置map输出value类型
job.setMapOutputValueClass(IntWritable.class);
//设置最终输出key
job.setOutputKeyClass(Text.class);
//设置最终输出value
job.setOutputValueClass(IntWritable.class);
//文件输入
FileInputFormat.setInputPaths(job, new Path(args[0]));
//最终结果输出
FileOutputFormat.setOutputPath(job, new Path(args[1]));
//执行job
job.waitForCompletion(true);
}
}
#本地连接hdfs集群,配置参数然后运行,设置环境变量
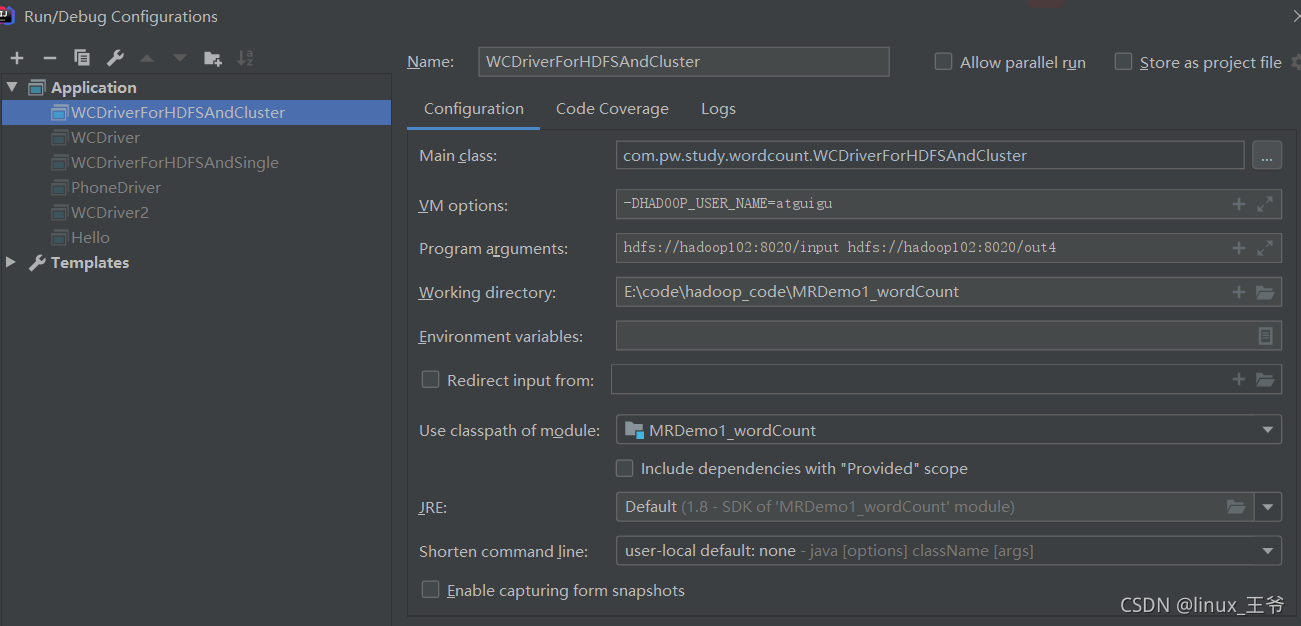
?注意的地方是5.6步先打包(打包是还没有设置jar的代码)
package com.pw.study.wordcount;
import org.apache.hadoop.conf.Configuration;
import org.apache.hadoop.fs.Path;
import org.apache.hadoop.io.IntWritable;
import org.apache.hadoop.io.Text;
import org.apache.hadoop.mapreduce.Job;
import org.apache.hadoop.mapreduce.lib.input.FileInputFormat;
import org.apache.hadoop.mapreduce.lib.output.FileOutputFormat;
import java.io.IOException;
/**
* hdfs 集群启动
* 1.配置参数
* //设置在集群运行的相关参数-设置HDFS,NAMENODE的地址
* configuration.set("fs.defaultFS", "hdfs://hadoop102:8020");
* //指定MR运行在Yarn上
* configuration.set("mapreduce.framework.name","yarn");
* //指定MR可以在远程集群运行
* configuration.set(
* "mapreduce.app-submission.cross-platform","true");
* //指定yarn resourcemanager的位置
* configuration.set("yarn.resourcemanager.hostname",
* "hadoop103")
* 2.设置主启类
* 3.将文件路劲输入修改为入参
* 4.配置环境信息(就是在IDE的运行参数配置)
* 5.打包完成后注释主启动类
* 6.设置jar包绝对路径
* */
public class WCDriverForHDFSAndCluster {
public static void main(String[] args) throws IOException, ClassNotFoundException, InterruptedException {
Configuration conf = new Configuration();
//设置在集群运行的相关参数-设置HDFS,NAMENODE的地址
conf.set("fs.defaultFS", "hdfs://hadoop102:8020");
//指定MR运行在Yarn上
conf.set("mapreduce.framework.name", "yarn");
//指定MR可以在远程集群运行
conf.set("mapreduce.app-submission.cross-platform", "true");
//指定yarn resourcemanager的位置
conf.set("yarn.resourcemanager.hostname", "hadoop103");
// 启动mrJob
Job job = Job.getInstance(conf);
//设置主启动类
// job.setJarByClass(WCDriverForHDFSAndCluster.class);
//设置jar的绝对路径并注释掉主启动类
job.setJar("E:\\code\\hadoop_code\\MRDemo1_wordCount\\target\\MRDemo1_wordCount-1.0-SNAPSHOT.jar");
/*设置map实现类*/
job.setMapperClass(WCMapper.class);
/*设置reduce实现类*/
job.setReducerClass(WCReducer.class);
//设置map输出key类型
job.setMapOutputKeyClass(Text.class);
//设置map输出value类型
job.setMapOutputValueClass(IntWritable.class);
//设置最终输出key
job.setOutputKeyClass(Text.class);
//设置最终输出value
job.setOutputValueClass(IntWritable.class);
//文件输入
FileInputFormat.setInputPaths(job, new Path(args[0]));
//最终结果输出
FileOutputFormat.setOutputPath(job, new Path(args[1]));
//执行job
job.waitForCompletion(true);
}
}
|