准备工作
from pyspark import SparkContext, SparkConf
from pyspark.sql import SparkSession
spark = SparkSession \
.builder \
.appName("Python Spark IBM Cloud Example") \
.config("spark.some.config.option", "some-value") \
.getOrCreate()
创建RDD
nums = [i for i in range(10)]
rdd = sc.parallelize(nums)
print("Number of partitions: {}".format(rdd.getNumPartitions()))
print("Partitioner: {}".format(rdd.partitioner))
print("Partitions structure: {}".format(rdd.glom().collect()))
使用getNumPartitions() 查看分区数量,使用glom() 查看分区内容。
output:
 在函数parallelize()指定分区数量
- 分区为2
rdd = sc.parallelize(nums, 2)
print("Default parallelism: {}".format(sc.defaultParallelism))
print("Number of partitions: {}".format(rdd.getNumPartitions()))
print("Partitioner: {}".format(rdd.partitioner))
print("Partitions structure: {}".format(rdd.glom().collect()))
output: 
- 分区为15
rdd = sc.parallelize(nums, 15)
print("Number of partitions: {}".format(rdd.getNumPartitions()))
print("Partitioner: {}".format(rdd.partitioner))
print("Partitions structure: {}".format(rdd.glom().collect()))
output:

使用partitionBy()函数
其中,数据集需要是具有键/值对的元组,因为默认分区器使用键的哈希值将元素分配给分区。
rdd = sc.parallelize(nums) \
.map(lambda el: (el, el)) \
.partitionBy(2) \
.persist()
print("Number of partitions: {}".format(rdd.getNumPartitions()))
print("Partitioner: {}".format(rdd.partitioner))
print("Partitions structure: {}".format(rdd.glom().collect()))
j=0
for i in rdd.glom().collect():
j+=1
print("partition: " + str(j) + " "+ str(i))
output:

显式分区
准备数据:
transactions = [
{'name': 'Bob', 'amount': 100, 'country': 'United Kingdom'},
{'name': 'James', 'amount': 15, 'country': 'United Kingdom'},
{'name': 'Marek', 'amount': 51, 'country': 'Poland'},
{'name': 'Johannes', 'amount': 200, 'country': 'Germany'},
{'name': 'Thomas', 'amount': 30, 'country': 'Germany'},
{'name': 'Paul', 'amount': 75, 'country': 'Poland'},
{'name': 'Pierre', 'amount': 120, 'country': 'France'},
{'name': 'Frank', 'amount': 180, 'country': 'France'}
]
为了使得每一个国家在一个node中,我们使用自定义分区:
def country_partitioner(country):
return hash(country)% (10**7+1)
rdd = sc.parallelize(transactions) \
.map(lambda el: (el['country'], el)) \
.partitionBy(3, country_partitioner)
print("Number of partitions: {}".format(rdd.getNumPartitions()))
print("Partitioner: {}".format(rdd.partitioner))
print("Partitions structure: {}".format(rdd.glom().collect()))
print("\n--\n")
for i, j in enumerate(rdd.glom().collect()):
print("\npartition: " + str(i+1) + "\n"+ str(j))
output:
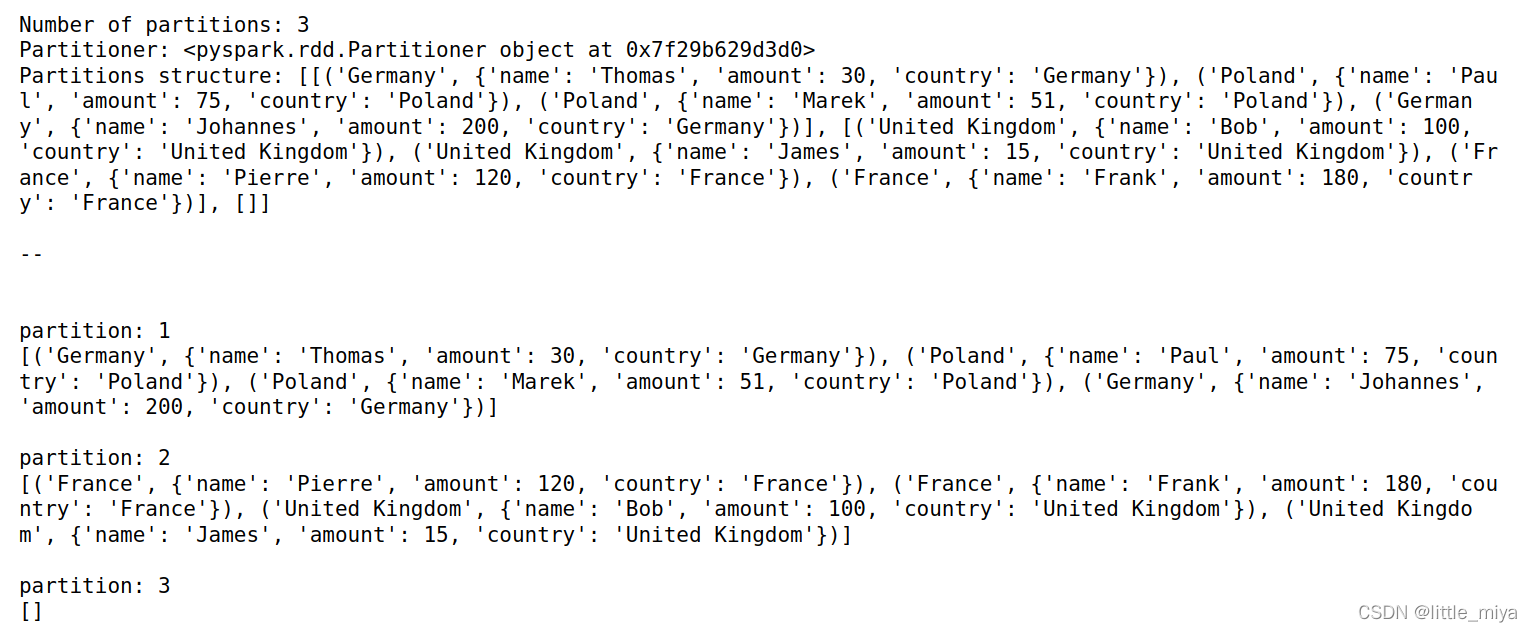 利用partition做计算
使用mapPartitions() 函数可以单独对每个partition进行map运算。
def sum_sales(iterator):
yield sum(transaction[1]['amount'] for transaction in iterator)
by_country = sc.parallelize(transactions) \
.map(lambda el: (el['country'], el)) \
.partitionBy(5, country_partitioner)
sum_amounts = by_country \
.mapPartitions(sum_sales) \
.collect()
print("Total sales for each partition: {}".format(sum_amounts))
output:
每个国家对应的amounts总数

使用dataframe查看partition效果
df = spark.createDataFrame(transactions)
for i, j in enumerate(df.rdd.glom().collect()):
print("partition: " + str(i+1) + "\n"+ str(j))
output: 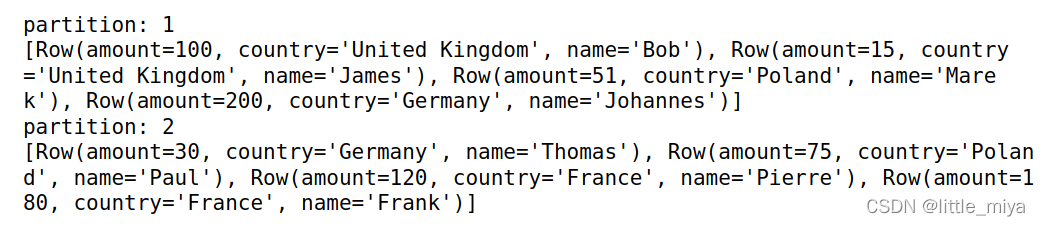 可知数据分布在两个partition。
使用repartition函数
可以直接指定列名:
df2 = df.repartition(10,"country")
print("\nAfter 'repartition()'")
print("Number of partitions: {}".format(df2.rdd.getNumPartitions()))
print("Partitioner: {}".format(df2.rdd.partitioner))
print("Partitions structure: {}".format(df2.rdd.glom().collect()))
outoput:
 查看每个partition中的数据:
for i, j in enumerate(df2.rdd.glom().collect()):
print("partition: " + str(i+1) + "\n"+ str(j))
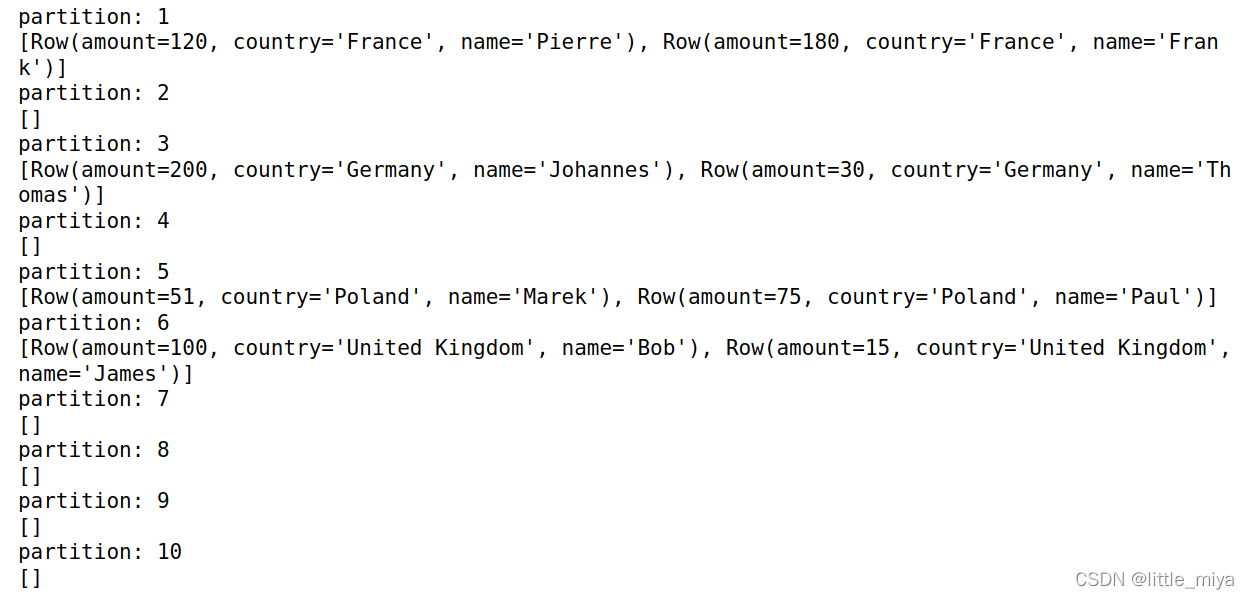
|