场景
有时候需要处理并存储大量流式数据,以Gps数据为例,面对一天千万级别的数据,时常要分库分表,数据偶尔会存在丢失的情况,很难做到既要保证大量数据处理的同时又要保证数据查询的实时性。这时借助云平台就是一个很好的做法。
数据流向
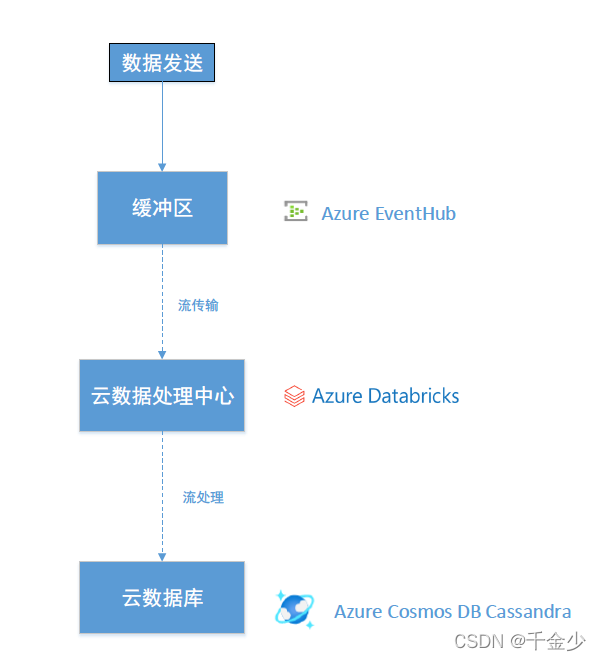
大致数据流向数据源->缓冲区->数据库处理中心->云数据库
所用Azure云平台介绍
1.Azure EventHub (云Kakfa) Azure 事件中心是大数据流式处理平台和事件引入服务。 它可以每秒接收和处理数百万个事件。 可以使用任何实时分析提供程序或批处理/存储适配器转换和存储发送到事件中心的数据。 2.Azure Databricks (云Spark) 是一个已针对 Microsoft Azure 云服务平台进行优化的数据分析平台。 Azure Databricks开发数据密集型应用程序的三个环境:Databricks SQL、Databricks Data Science Engineering 和 & Databricks 机器学习。Databricks SQL 为想要针对数据湖运行 SQL 查询、创建多种可视化类型以从不同角度探索查询结果,以及生成和共享仪表板的分析员提供了一个易于使用的平台。 3.Azure Cosmos DB Cassandra(云数据库) Azure Cosmos DB Cassandra API 可以充当为 Apache Cassandra 编写的应用的数据存储。 这意味着通过使用现有的符合 CQLv4 的 Apache 驱动程序,现有 Cassandra 应用程序现在可以与 Azure Cosmos DB Cassandra API 通信。 在许多情况下,只需更改连接字符串,就可以从使用 Apache Cassandra 切换为使用 Azure Cosmos DB Cassandra API。通过 Cassandra API 可以使用 Cassandra 查询语言 (CQL)、基于 Cassandra 的工具(如 cqlsh)和熟悉的 Cassandra 客户端驱动程序与 Azure Cosmos DB 中存储的数据进行交互。
技术方案优势
1.高效实时,高吞吐(理论上只要预算够无论多大的数据都能处理) 2.只要部署好,根本不用担心自己搭的服务器会爆炸 3.减少各种环境搭建过程,减少人力机器维护成本 4.后续查询扩展快速方便,可以根据业务需求做出各种定制
具体讲解
- 数据发送部分
数据发送部分主要将数据源发送至EventHub,那么这里以.net为例附上一部分发送代码,因为是在老项目上改的,用的是.net fk,所以没有太多的操作空间(不然不兼容),EventHub使用环境在.net fk4.6以上,大家记得更新框架,推荐使用.net core或者.net 5的BackGroundService。 大概说一下思路,一个并发队列,4线程发送,而且做了分区,可以根据自身语言环境或者需要更改,最好自己写
using System;
using System.Collections.Concurrent;
using System.Collections.Generic;
using System.Linq;
using System.Threading;
using System.Threading.Tasks;
using Azure.Messaging.EventHubs;
using Azure.Messaging.EventHubs.Producer;
using Microsoft.AppCenter.Crashes;
namespace EHiGPSSocket.FormsApp
{
public static class EventHub
{
private const string ConnectionString =
"Your ConnectionString ";
private const string EventHubName = "EventHubName";
private const int Capacity = 500;
private const int MacCapacity = 200000;
private static EventHubProducerClient _producerClient =
new EventHubProducerClient(ConnectionString, EventHubName);
private static ConcurrentDictionary<string, ConcurrentQueue<string>> DicQueues = new ConcurrentDictionary<string, ConcurrentQueue<string>>();
static EventHub()
{
for (int i = 0; i < 4; i++)
{
Task.Factory.StartNew(SendAsync, CancellationToken.None, TaskCreationOptions.LongRunning);
}
}
public static void Enqueue(string message, string topic)
{
if (!DicQueues.Keys.Contains(topic))
{
var queue = new ConcurrentQueue<string>();
queue.Enqueue(message);
DicQueues.TryAdd(topic, queue);
}
else
{
DicQueues.TryGetValue(topic, out var queue);
if (queue?.Count < MacCapacity)
{
queue?.Enqueue(message);
}
}
}
static async Task SendAsync(object state)
{
while (true)
{
try
{
foreach (var dicQueue in DicQueues)
{
var queue = dicQueue.Value;
if (queue.Count > Capacity)
{
var count = 0;
var list = new List<EventData>();
while (count < Capacity && count < dicQueue.Value.Count)
{
queue.TryDequeue(out var data);
if (!string.IsNullOrEmpty(data))
{
list.Add(new EventData(data));
count++;
}
}
if (_producerClient.IsClosed)
{
_producerClient = new EventHubProducerClient(ConnectionString, EventHubName);
}
await _producerClient.SendAsync(list, new SendEventOptions() { PartitionKey = dicQueue.Key }).ConfigureAwait(false);
}
}
}
catch (Exception e)
{
Crashes.TrackError(e);
}
}
}
}
}
2.EventHub 数据发送至EventHub后,可以看到大致的数据量 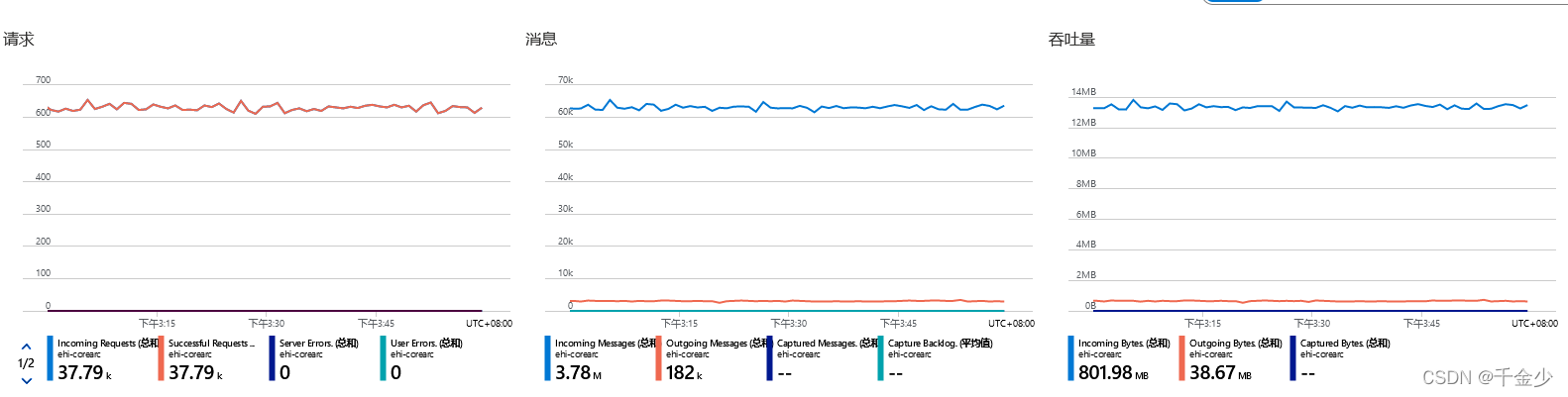
3.Azure Databricks Azure Databricks从EventHub读取数据 大致的读取代码如下Scala
def EventHandle(): Unit = {
val namespaceName = "namespaceName "
val eventHubName = "eventHubName "
val sasKeyName = "sasKeyName "
val sasKey = "sasKey "
val domainName="domainName.chinacloudapi.cn"
val connStr = new com.microsoft.azure.eventhubs.ConnectionStringBuilder()
.setEndpoint(namespaceName,domainName)
.setEventHubName(eventHubName)
.setSasKeyName(sasKeyName)
.setSasKey(sasKey)
val customEventhubParameters =EventHubsConf(connStr.toString()).setMaxEventsPerTrigger(100)
customEventhubParameters.setConsumerGroup("$Default")
println(customEventhubParameters.consumerGroup)
println(customEventhubParameters.connectionString)
val carDF = spark.sql("select * from xxxx")
val incomingStream = spark.readStream.format("eventhubs").options(customEventhubParameters.toMap).load()
val messages =incomingStream.withColumn("Body", $"body".cast(StringType)).withColumn("PartitionKey", $"PartitionKey".cast(StringType)).select("Body","PartitionKey")
val query= messages.writeStream.foreachBatch{(batchDF: DataFrame, batchId: Long)=>
batchDF.filter("PartitionKey='DBQ'")
.write.cassandraFormat("gpsdata", "gpsprofile").mode("append").save()
}
query.start().awaitTermination()
}
4.Azure Cosmos DB Cassandra 最后丢入Azure Cosmos DB Cassandra 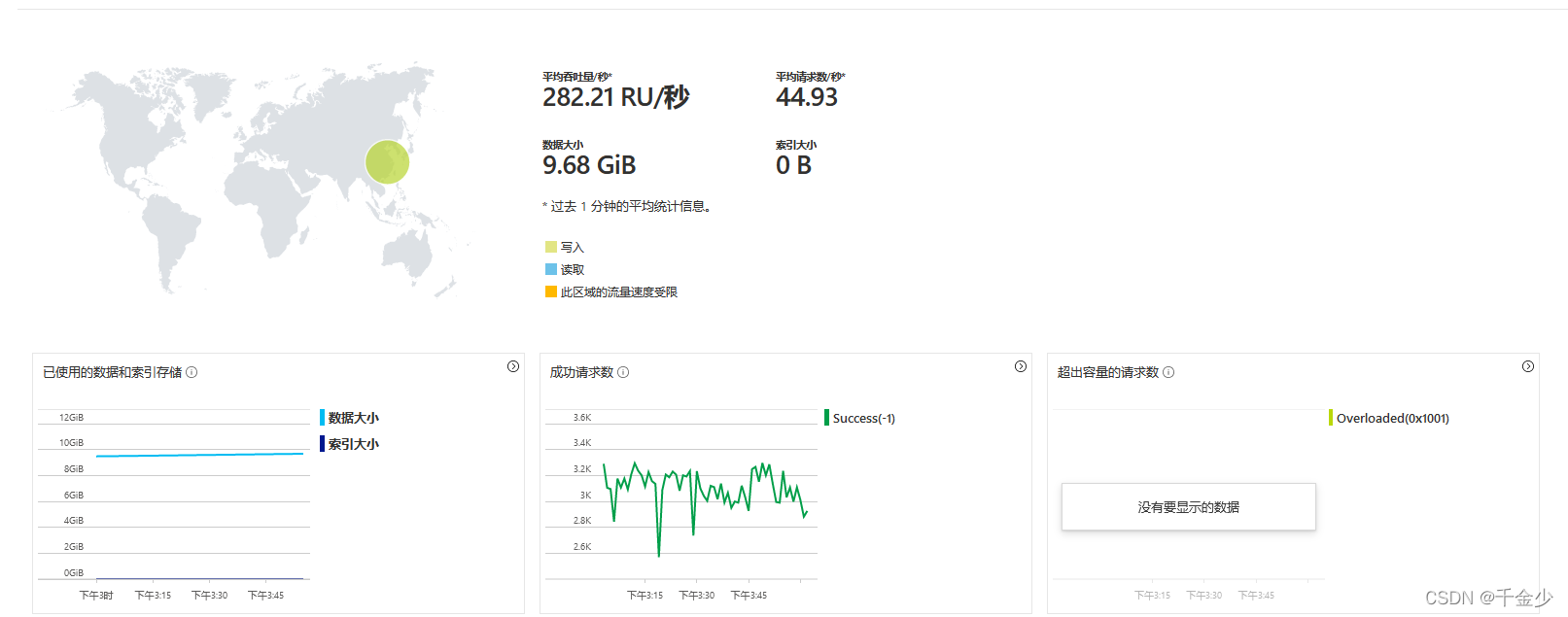
总结
EventHub Azure Databricks Azure Cosmos DB Cassandra 这三个云平台中需要有很多可以配置的地方,大家参阅官方文档应该都可以解决,这里只 、提供一些思路和解决方案,具体操作与选型还是要看个人业务需求与技术选型
|