在实现一个留存业务需求时,碰到了一个难题,我需要提供展示一个按照如下图格式的数据, 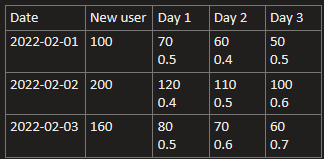 day 1 ~ day n的第一行是留存用户数量,第二行是一个由多个事件组合执行四则算术运算得到的复合数值,这里碰到的难点主要是第二行的计算,如果只想查看第二行的解决方法可以点击这里
由于数据传输速率受限,我不能使用先查询出所有数据然后在代码里处理数据的方法,因此我需要在sql查询中尽量完成所有聚合计算以减少查询返回的行数
留存模型采用的是经典模型(Classic retention)留存用户的数量都是在各天day n独立计算的
这里day 1~day n第一行计算新用户留存数量,第二行的小数计算留存的新用户中某个混合事件 的表现,混合事件 可以是由某一事件计算得到的值或者由多个事件进行四则运算得到的组合事件的值,第二行的值计算是这篇文章要讲的重点
例如求某个活动事件有两个入口entrance_a 和entrance_b ,结束通关标识事件为event_over
假设事件名称event_name 为"activety_1" ,用户表为t_user ,事件表为t_event t_user 表的数据是这样的 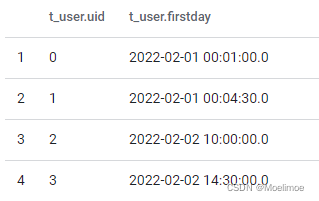 t_event 表的数据是这样的 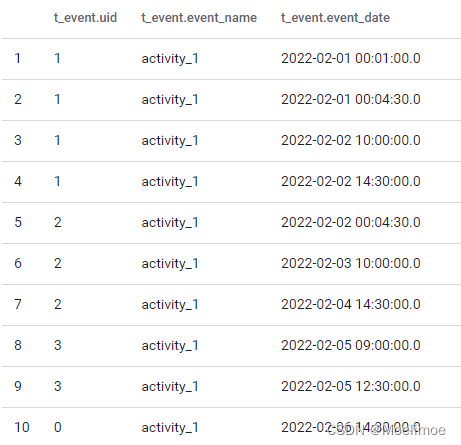
1
计算第一行, 也就是计算经典留存模型day 1~day n的留存用户量,可以用t_user和t_event的join和case when语句实现:
with temp_user as (
select distinct
`uid`,
to_date(`firstday`) as `firstday`
from `t_user`
where firstday >= "2022-02-01" and firstday < "2022-02-04"
),
temp_event as (
select distinct
`uid`,
to_date(`event_date`) as `event_date`
from `t_event`
where event_name = "activity_1"
and event_date >= "2022-02-01"
and event_date < "2022-02-07"
)
select
`firstday`,
Count(distinct a.uid) as `new_user`,
Count(distinct case when event_date = date_add(firstday, 1) then a.uid end) as `day 1`,
Count(distinct case when event_date = date_add(firstday, 2) then a.uid end) as `day 2`,
Count(distinct case when event_date = date_add(firstday, 3) then a.uid end) as `day 3`
from temp_user a left join temp_event b on a.uid = b.uid
group by firstday
用以上sql语句查询得到的结果: 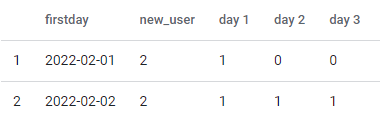 格式跟开头的图中的表格保持了一致,数值稍微验证一下可知没有问题,求第一行的留存用户数相对较简单
2
计算第二行的值,我想计算事件activity_1 在day 1 ~ day n的通关表现,具体来说就是要计算出day 1~day n各留存用户的(entrance_a + entrance_b)/event_over 值
现在假设事件表t_event_1 的内容是这样的 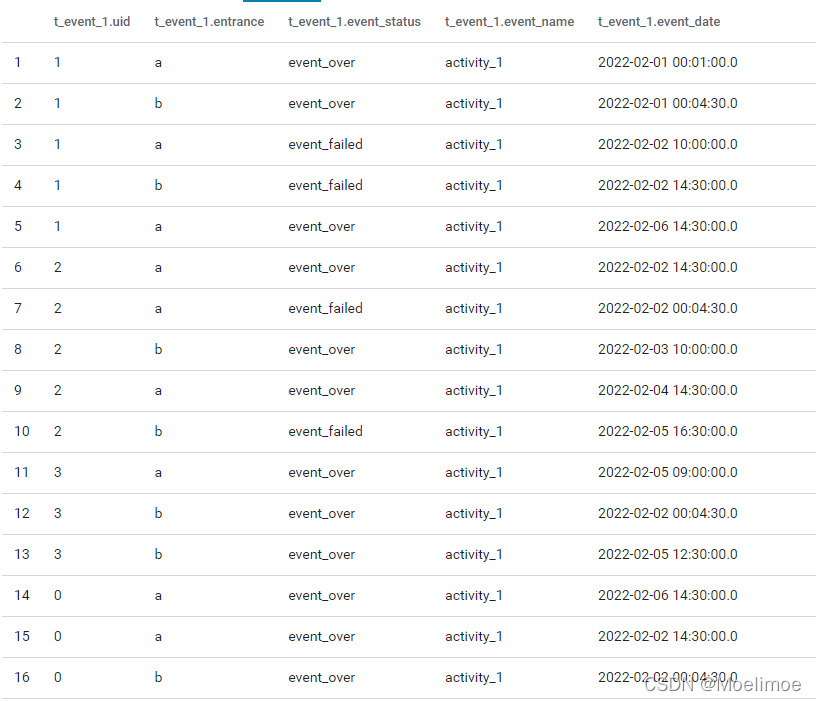 用户表t_user 同之前的不变 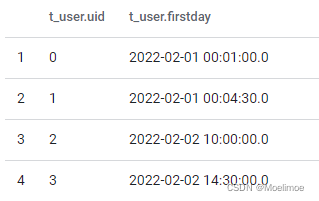 要计算出day 1~day n各留存用户的(entrance_a + entrance_b)/event_over 的表现,结果展示格式类似下面这张图 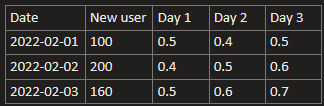 除了要按day 1 ~ day n展示数据外还涉及到属性entrance_a , entrance_b 等的聚合计算,使用分析函数(Analytics Function)并不能降低得到的行数,这里我采用了先把要统计的数据(entrance_a, entrance_b, event_over )先分别计算出来按firstday 和event_date 分组成行,然后再利用case when和求和语句把算出来的结果聚合到对应的day n,写出来的sql如下
with temp_user as (
select distinct
`uid`,
to_date(`firstday`) as `firstday`
from `t_user`
where firstday >= "2022-02-01" and firstday < "2022-02-04"
),
temp_event_1 as (
select
a.uid,
to_date(a.firstday) `firstday`,
to_date(b.event_date) `event_date`,
count(case when b.entrance = "a" then 1 end) as entrance_a,
count(case when b.entrance = "b" then 1 end) as entrance_b,
count(case when b.event_status = "event_over" then 1 end) as event_over
from t_user a left join t_event_1 b on a.uid = b.uid
and to_date(b.event_date) between date_add(a.firstday, 1) and date_add(a.firstday, 3)
and (b.entrance in ("a", "b") OR b.event_status = "event_over")
and b.event_date >= "2022-02-01"
and b.event_date < "2022-02-07"
group by firstday, event_date, a.uid
)
select
evt.firstday,
count(distinct evt.uid) `new user`,
(sum(case when date_add(evt.firstday, 1) = evt.event_date then evt.entrance_a else 0 end ) +
sum(case when date_add(evt.firstday, 1) = evt.event_date then evt.entrance_b else 0 end )) /
sum(case when date_add(evt.firstday, 1) = evt.event_date then evt.event_over else 0 end ) `day 1`,
(sum(case when date_add(evt.firstday, 2) = evt.event_date then evt.entrance_a else 0 end ) +
sum(case when date_add(evt.firstday, 2) = evt.event_date then evt.entrance_b else 0 end )) /
sum(case when date_add(evt.firstday, 2) = evt.event_date then evt.event_over else 0 end ) `day 2`,
(sum(case when date_add(evt.firstday, 3) = evt.event_date then evt.entrance_a else 0 end ) +
sum(case when date_add(evt.firstday, 3) = evt.event_date then evt.entrance_b else 0 end )) /
sum(case when date_add(evt.firstday, 3) = evt.event_date then evt.event_over else 0 end ) `day 3`
from temp_event_1 evt
group by firstday
查询的结果: 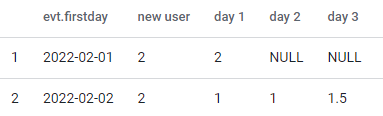 这里出现的null如果你想在sql中把它们变成0也可以使用zeroifnull() 函数将每一列计算结果包住
验证正确性 根据用户表t_user 和事件表t_event_1 对比查询结果的正确性: 用户表 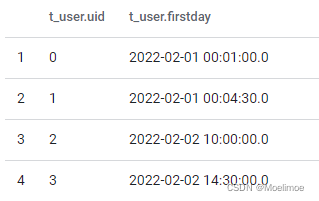 先看firstday在02-01的用户,只有0和1两个,对应的day 1就是他们在02-02的(entrance_a+entrance_b)/event_over 表现值: 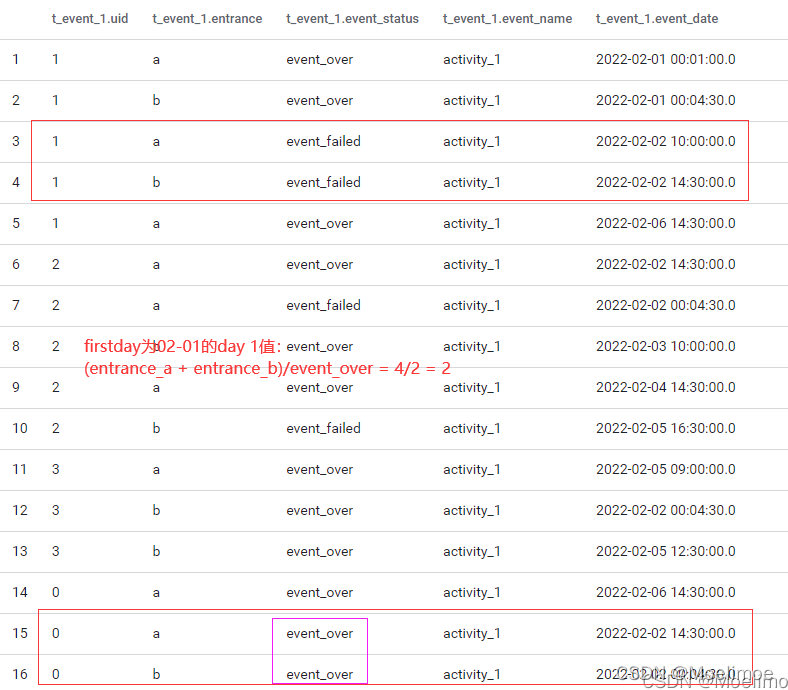 再检查02-02的用户2和3在day 1, day 2, day3的表现数值,也就是他们分别再02-03, 02-04和02-05的表现数值: 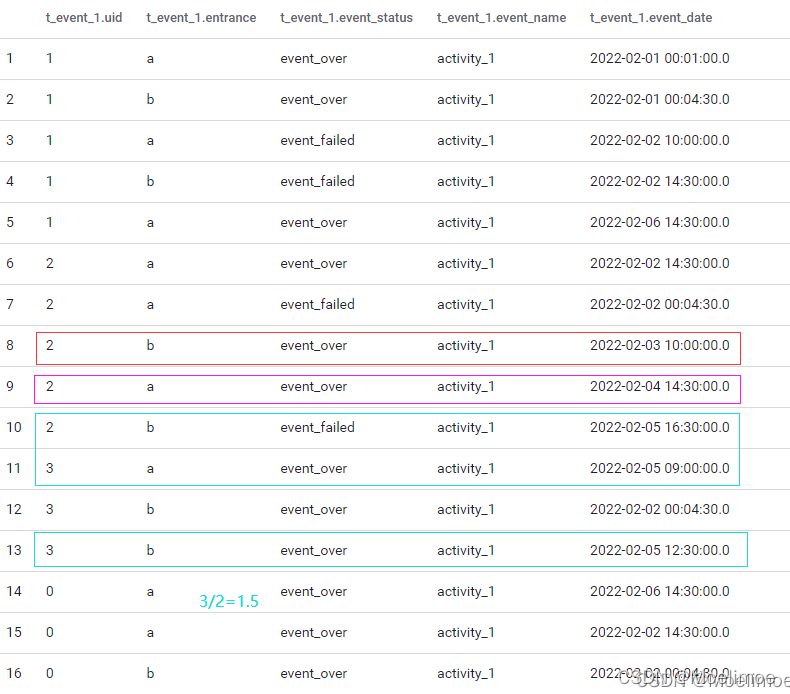 根据验证结果查询是没有问题的
day 1 ~ day n第二行的解决sql相对来说比较复杂,但是我目前没有想到更好的sql,如果有更好的方法查出结果,欢迎评论告诉我,感谢~
以上的模拟数据,都可以在这个链接(https://demo.gethue.com/hue/home)找到,账号密码登录的时候都是demo,找到Hive下的数据库选择default子库 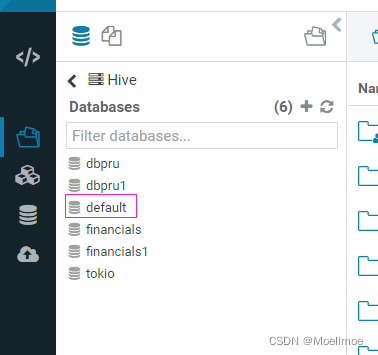 Editor选择Hive就可以查询了 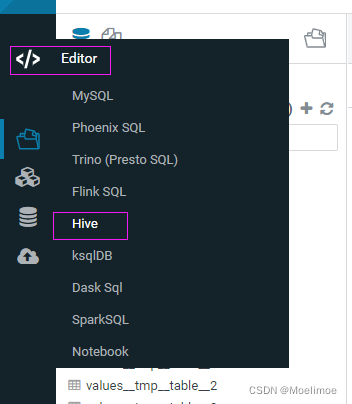 你也可以选择自己创建新表和插入数据模拟,以下是我的建表和插入数据的sql:
CREATE TABLE `t_user`(
`uid` int,
`firstday` timestamp)
ROW FORMAT SERDE
'org.apache.hadoop.hive.serde2.lazy.LazySimpleSerDe'
STORED AS INPUTFORMAT
'org.apache.hadoop.mapred.TextInputFormat'
OUTPUTFORMAT
'org.apache.hadoop.hive.ql.io.HiveIgnoreKeyTextOutputFormat'
LOCATION
'hdfs://namenode:8020/user/hive/warehouse/t_user'
;
INSERT INTO t_user (uid, firstday)
VALUES
(0, '2022-02-01 00:01:00'),
(1, '2022-02-01 00:04:30'),
(2, '2022-02-02 10:00:00'),
(3, '2022-02-02 14:30:00')
;
CREATE TABLE `t_event`(
`uid` int,
`event_name` STRING,
`event_date` TIMESTAMP
)
ROW FORMAT SERDE
'org.apache.hadoop.hive.serde2.lazy.LazySimpleSerDe'
STORED AS INPUTFORMAT
'org.apache.hadoop.mapred.TextInputFormat'
OUTPUTFORMAT
'org.apache.hadoop.hive.ql.io.HiveIgnoreKeyTextOutputFormat'
LOCATION
'hdfs://namenode:8020/user/hive/warehouse/t_event'
;
INSERT INTO t_event (uid, event_name, event_date)
VALUES
(1, "activity_1", '2022-02-01 00:01:00'),
(1, "activity_1", '2022-02-01 00:04:30'),
(1, "activity_1", '2022-02-02 10:00:00'),
(1, "activity_1", '2022-02-02 14:30:00'),
(2, "activity_1", '2022-02-02 00:04:30'),
(2, "activity_1", '2022-02-03 10:00:00'),
(2, "activity_1", '2022-02-04 14:30:00'),
(3, "activity_1", '2022-02-05 09:00:00'),
(3, "activity_1", '2022-02-05 12:30:00'),
(0, "activity_1", '2022-02-06 14:30:00')
;
CREATE TABLE `t_event_1`(
`uid` int,
`entrance` string,
`event_status` STRING,
`event_name` STRING,
`event_date` TIMESTAMP
)
ROW FORMAT SERDE
'org.apache.hadoop.hive.serde2.lazy.LazySimpleSerDe'
STORED AS INPUTFORMAT
'org.apache.hadoop.mapred.TextInputFormat'
OUTPUTFORMAT
'org.apache.hadoop.hive.ql.io.HiveIgnoreKeyTextOutputFormat'
LOCATION
'hdfs://namenode:8020/user/hive/warehouse/t_event_1'
;
INSERT INTO `t_event_1` (uid, entrance, event_status, event_name, event_date)
VALUES
(1, "a", "event_over", "activity_1", '2022-02-01 00:01:00'),
(1, "b", "event_over", "activity_1", '2022-02-01 00:04:30'),
(1, "a", "event_failed", "activity_1", '2022-02-02 10:00:00'),
(1, "b", "event_failed", "activity_1", '2022-02-02 14:30:00'),
(1, "a", "event_over", "activity_1", '2022-02-06 14:30:00'),
(2, "a", "event_over", "activity_1", '2022-02-02 14:30:00'),
(2, "a", "event_failed", "activity_1", '2022-02-02 00:04:30'),
(2, "b", "event_over", "activity_1", '2022-02-03 10:00:00'),
(2, "a", "event_over", "activity_1", '2022-02-04 14:30:00'),
(2, "b", "event_failed", "activity_1", '2022-02-05 16:30:00'),
(3, "a", "event_over", "activity_1", '2022-02-05 09:00:00'),
(3, "b", "event_over", "activity_1", '2022-02-02 00:04:30'),
(3, "b", "event_over", "activity_1", '2022-02-05 12:30:00'),
(0, "a", "event_over", "activity_1", '2022-02-06 14:30:00'),
(0, "a", "event_over", "activity_1", '2022-02-02 14:30:00'),
(0, "b", "event_over", "activity_1", '2022-02-02 00:04:30')
;
注意你登录的session只会保持一段时间,大概十几分钟或更多?,一般你可以通过re-create session来重新打开并继续执行sql查询,如果re-create没有效果就只能重新登录了 
|