一、Flume背景
Hadoop业务的整体开发流程: 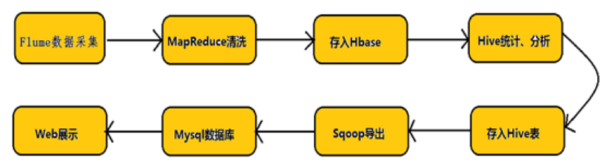 从Hadoop的业务开发流程图中可以看出,在大数据的业务处理过程中,对于数据的采集是十分重要的一步,也是不可避免的一步。 许多公司的平台每天会产生大量的日志(一般为流式数据,如,搜索引擎的pv,查询等),处理这些日志需要特定的日志系统,一般而言,这些系统需要具有以下特征: (1) 构建应用系统和分析系统的桥梁,并将它们之间的关联解耦; (2) 支持近实时的在线分析系统和类似于Hadoop之类的离线分析系统; (3) 具有高可扩展性。即:当数据量增加时,可以通过增加节点进行水平扩展。 flume.apache.org (flume官网)
二、Flume介绍
Flume是一个分布式、可靠、高可用的海量日志聚合系统,支持在系统中定制各类数据发送方,用于收集数据;同时,Flume提供对数据的简单处理,并写到各种数据接收方的能力。 Flume在0.9.x and 1.x之间有较大的架构调整,1.x版本之后的改称Flume NG(next generation),0.9.x的称为Flume OG(originalgeneration)。 对比OG版本, Flume NG (1.x.x)的主要变化如下: ① sources和sinks 使用channels进行链接 ② 两个主要channel。in-memorychannel 非持久性支持,速度快。JDBC-based channel 持久性支持,速度偏慢。 ③ 不再区分逻辑和物理node,所有物理节点统称为“agents”,每个agents 都能运行0个或多个sources 和sinks ④ 不再需要master节点和对zookeeper的依赖,配置文件简单化。 ⑤ 插件化,一部分面对用户,工具或系统开发人员。 ⑥ 使用Thrift、Avro Flume sources 可以从Flume0.9.4 发送 events 到Flume 1.x 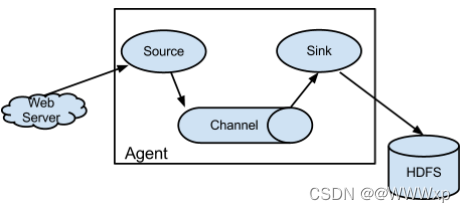
其中相关组件成员如下: 组件 功能 Agent 使用JVM运行Flume。每台机器运行一个Agent,但是可以在一个Agent中包含多个sources和sinks Client 生产数据,运行在一个独立的线程 Source 从Client收集数据,传递给Channel Sink 从Channel中接收数据,运行在一个独立的线程 Channel 连接Source与Sink,有点类似队列 Events 可以是日志记录、 avro 对象等。 Flume架构整体上看就是source-channel-sink的三层架构,类似于生产者和消费者的架构。它们通过channel传输,解耦。 Flume以Agent作为最小的独立运行单位。一个Agent就是一个JVM。单Agent由 Source、Sink和Channel三大构件组成。 事件是Flume的基本数据单位,它携带日志数据(字节数组形式)并且携带头信息,这些Event由Agent外的数据源组成。 当Source捕获事件后会进行特定的格式化,然后Source会把事件推入(单个或多个Channel中)。你可以把Channel看作为一个缓冲区,它将保存事件直到Sink处理完该事件。Sink负责持久化日志或将事件推向另一个Source。 Flume支持用户建立多级流,也就是说,多个Agent可以协同工作。
2.1 Flume Source介绍
Flume Source是数据的收集端,负责将数据捕获后进行特殊的格式化,将数据封装到事件(event)里,然后将事件推入到Channel中。Flume提供了各种source的实现,包括Avro Source、Exce Source、Spooling Directory Source、NetCat Source、Syslog Source、Syslog TCP Source、Syslog UDP Source、HTTP Source、HDFS Source,etc。如果内置的Source无法满足需要,Flume还支持自定义Source。
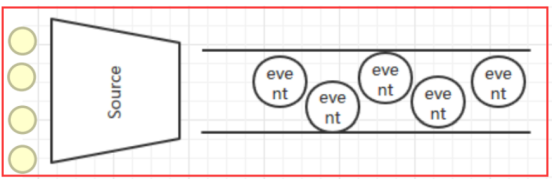
2.2 Flume Channel介绍
Channel是连接Source和Sink的组件,我们可以将它看做一个数据的缓冲区(数据队列),它可以将事件暂存到内存中也可以持久化到本地磁盘上, 直到Sink处理完该事件。 Flume对于Channel,则提供了Memory Channel、JDBC Chanel、File Channel,etc。 ① MemoryChannel可以实现高速的吞吐,但是无法保证数据的完整性。 ② MemoryRecoverChannel在官方文档的建议上已经建义使用FileChannel来替换。 ③ FileChannel保证数据的完整性与一致性。在具体配置不现的FileChannel时,建议FileChannel设置的目录和程序日志文件保存的目录设成不同的磁盘,以便提高效率。
2.3 Flume Sink介绍
Flume Sink取出Channel中的数据,进行相应的存储文件系统,数据库,或者提交到远程服务器。 Flume也提供了各种sink的实现,包括HDFS sink、Logger sink、Avro sink、File Roll sink、Null sink、HBase sink,etc。 Flume Sink在设置存储数据时,可以向文件系统中,数据库中,hadoop中存储数据,在日志数据较少时,可以将数据存储在文件系中,并且设定一定的时间间隔保存数据。在日志数据较多时,可以将相应的日志数据存储到Hadoop中,便于日后进行相应的数据分析。
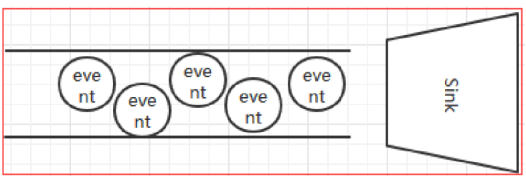
三、Flume的安装
安装规划:由slave1和slave2收集日志信息,传给master,再由master上传到hdfs上 #上传至slave1节点上并解压重命名 cd /usr/local tar -zxvf apache-flume-1.8.0-bin.tar.gz mv apache-flume-1.8.0-bin flume #配置flume-env.sh文件 cp flume-env.sh.template flume-env.sh vim flume-env.sh #将java的环境变量替换为自己的 export JAVA_HOME=/usr/local/jdk1.8.0_121 #配置flume的环境变量 vim /etc/profile source /etc/profile #查看flume的版本 flume-ng version 
四、Flume的简单案例
4.1编写配置文件
#此配置定义了一个名称为a1的agent 分别指定了sources sinks channels a1.sources = r1 a1.sinks = k1 a1.channels = c1
#描述/配置源 a1.sources.r1.type = netcat a1.sources.r1.bind = localhost a1.sources.r1.port = 44444
#描述了sink 日志收集 a1.sinks.k1.type = logger
#使用在内存中缓存事件的通道 a1.channels.c1.type = memory a1.channels.c1.capacity = 1000 a1.channels.c1.transactionCapacity = 100
#将channel绑定到source与sink上 a1.sources.r1.channels = c1 a1.sinks.k1.channel = c1
4.2启动Flume服务
Flume的服务需要使用flume-ng启动,同学们需要在命令行上指定agent名称,配置文件目录与配置文件 bin/flume-ng agent -c conf -f conf/flume-conf.properties -n a1 -Dflume.root.logger=INFO,console
4.3使用telnet发送测试数据
安装xinted服务:yum install xinetd 安装telnet服务端:yum install telnet-server 安装telnet客户端:yum install telnet.* 配置并启动Telnet,xinetd和telnet必须设置开机启动,否则无法启动Telnet服务: ① systemctl enable xinetd.service ② systemctl enable telnet.socket 接下来启动服务: ① systemctl start telnet.socket ② systemctl start xinetd 使用telnet测试: telnet localhost 44444 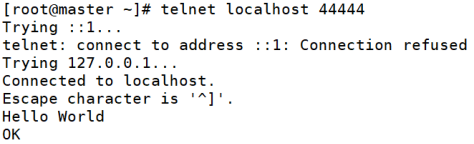 查看接收的消息: 
五、Flume与Hadoop的集成
5.1 拷贝配置文件
将Hadoop配置目录下的hdfs-site.xml、core-site.xml两个文件拷贝到flume的conf目录下: cp hdfs-site.xml core-site.xml /usr/local/flume/conf/
5.2 拷贝依赖文件
将以下的jar文件copy到flume的lib目录下:
六、Flume的实现案例
6.1 采集Hive的运行日志
案例一:将hive的日志文件中的数据采集到flume的日志中,并展示在前端 source: exce(exec source在启动时运行给定的unix命令,适用于每天的日志都来 源与一个文件) channel: memory(事件存储在具有可配置最大大小的内存队列中) sink: logger(以INFO为级别记录事件,通常用于测试和调试目的) 在flume的conf目录下新建文件如下: #新建hive-memory-log.properties文件 #指定source/channel/sink a1.sources = s1 a1.channels = c1 a1.sinks = k1
define the source
a1.sources.s1.type = exec a1.sources.s1.command = tail -f /usr/mydir/software/hive-2.3.3/logs/hive.log a1.sources.s1.shell = /bin/sh -c
define the channel
a1.channels.c1.type = memory #channel的总容量 a1.channels.c1.capacity = 100
define the sink
a1.sinks.k1.type = logger
bind
a1.sources.s1.channels = c1 a1.sinks.k1.channel = c1
启动flume服务,观察接收情况: bin/flume-ng agent -c conf -f conf/hive-memory-log.properties -n a1 -Dflume.root.logger=INFO,console
6.2 更换为File Channel
#复制上述案例的hive-memory-log.properties文件 cp hive-memory-log.properties hive-file-log.properties
File Channel特点:能够将source传递过来的数据进行临时文件存储 将hive-file-log.properties文件中的Channel对应的内容进行替换
define the channel
a1.channels.c1.type = file
存储检查点文件的目录(必须手动创建)
a1.channels.c1.checkpointDir = /root/flume/check
以逗号分隔的用于存储日志文件的目录列表(必须手动创建)
a1.channels.c1.dataDirs = /root/flume/data 再次运行flume服务与hive服务: bin/flume-ng agent -c conf -f conf/hive-file-log.properties -n a1 -Dflume.root.logger=INFO,console 查看/root/flume/data下的文件:
.lock文件为操作系统的锁文件,代表此data目录不允许其他进程访问 log-1文件为source发送的数据
6.3 将Sink更改为HDFS Sink
#复制上述案例的hive-file-log.properties文件 cp hive-mem-log.properties hive-mem-hdfs.properties
HDFS Sink:此接收器将事件写入hadoop分布式文件系统(hdfs)。它目前支持创建文本和序列文件。它支持两种文件类型的压缩。可以根据经过的时间、数据大小或事件数定期滚动文件(关闭当前文件并创建新文件)。它还通过时间戳或事件发生的机器等属性存储/分区数据。
#设定为hdfs的sink类型 a1.sinks.k1.type = hdfs #指向hdfs的指向位置 a1.sinks.k1.hdfs.path = /flume/hdfs #设置hdfs上文件的前缀 a1.sinks.k1.hdfs.filePrefix = hive-log #设置文件格式 DataStream不能被压缩 a1.sinks.k1.hdfs.hdfs.fileType = DataStream #设置写格式 a1.sinks.k1.hdfs.hdfs.writeFormat=Text 再次运行flume服务与hive服务: bin/flume-ng agent -c conf -f conf/hive-mem-hdfs.properties -n a1 -Dflume.root.logger=INFO,console 查看hdfs上对应/flume/hdfs下生成的文件:filePrefix.timestamp
查看结束:思考对应问题: 1: 每个上传的文件都很小,如何解决文件大小的问题? 2: 如何能够让文件实现按天存储?
6.4 设置文件大小与时间分区
#复制上述案例的hive-memory-log.properties文件 cp hive-mem-hdfs.properties hive-mem-hdfs-size.properties 设置Sink操作: #设定为hdfs的sink类型 a1.sinks.k1.type = hdfs #指向hdfs的指向位置 a1.sinks.k1.hdfs.path = /flume/size #设置hdfs上文件的前缀 a1.sinks.k1.hdfs.filePrefix = hive-log #设置回滚的size 单位为字节 相当于10kb a1.sinks.k1.hdfs.hdfs.rollSize = 10240 #相隔多少个event向hdfs中写入一个文件 0代表不设置 a1.sinks.k1.hdfs.rollCount = 0 #相隔多少秒向hdfs中写入一个时间 0代表不设置 a1.sinks.k1.hdfs.rollInterval = 0 启动Flume服务: bin/flume-ng agent -c conf -f conf/hive-mem-hdfs-size.properties -n a1 -Dflume.root.logger=INFO,console 查看位于/flume/size下的文件:
发现文件的容量在10kb左右,大于等于10kb。原因是要等待event中的内容写入完成,如果想要设置为hdfs的blockSize(128MB),建议设置为123MB,提供大于5MB的误差值。 如果想要安装时间去分配目录的话,那么需要配置hdfs.path参数,根据官网上的案例,重新添加上述的sink参数如下:
#指向hdfs的指向位置 基于日期格式按照每天一个目录 a1.sinks.k1.hdfs.path = /flume/date/%Y-%m-%d/ #设置系统的当前时间作为参照如不设置 将会出现异常 a1.sinks.k1.hdfs.useLocalTimeStamp = true 再次启动: bin/flume-ng agent -c conf -f conf/hive-mem-hdfs-size.properties -n a1 -Dflume.root.logger=INFO,console 查看位于/flume/date下的目录名称即可!
6.5 Spooling Dir Source的替换
在上述案例中,我们讲述了使用exec source完成的对应Flume的案例,那么exec source使用通过command命令进行对单个文件每天所产生的日志收集,那么如何能够动态的监控一个文件目录,将其之下的所有log文件进行采集的呢?那我们就选用Spoorling Directory Source! 复制上述案例文件: cp hive-mem-hdfs.properties dir-mem-hdfs.properties 配置如下: a1.sources = s1 a1.channels = c1 a1.sinks = k1
define the source
a1.sources.s1.type = spooldir
从中读取文件的目录 实现创建
a1.sources.s1.spoolDir=/root/flume/spooling/
define the channel
a1.channels.c1.type = memory a1.channels.c1.capacity = 100 #设定为hdfs的sink类型 a1.sinks.k1.type = hdfs #指向hdfs的指向位置 a1.sinks.k1.hdfs.path = /flume/dir #设置hdfs上文件的前缀 a1.sinks.k1.hdfs.filePrefix = hive-log #设置文件格式 DataStream不能被压缩 a1.sinks.k1.hdfs.hdfs.fileType = DataStream #设置写格式 a1.sinks.k1.hdfs.hdfs.writeFormat=Text
bind
a1.sources.s1.channels = c1 a1.sinks.k1.channel = c1 接下来,准备好一个我们简单的文本文件,随机写入些内容:
启动服务: bin/flume-ng agent -c conf -f conf/dir-mem-hdfs.properties -n a1 -Dflume.root.logger=INFO,console 接下来将我们刚刚创建的文件复制到spooling这个目录下: #文件复制操作 cp a.txt spooling/ #查看spooling目录下的文件 ll spooling/
我们发现此时a.txt文件已经重命名成.COMPLETED这样的文件了,这是在我们的Spooling Dir目录下设置已被采集的对应文件标识,标识着该文件已经被传输完成了。 查看HDFS:
6.6 Spooling Dir实现过滤操作
学习了上述的6.5案例,我们实现了能够实现动态的采集某个目录下的所有日志文件。 但这还不够,我们去考虑下日志输出的方式: 每天0点以后输出.tmp格式的文件,等到第二天的0点过后将文件转换为.log logs/20181001.log.tmp —> 20181001.log logs/20181002.log.tmp —> 20181002.log 我们可以设置过滤属性选择只加载.log格式的文件! 复制上述案例的配置文件: cp dir-mem-hdfs.properties filter-mem-hdfs.properties 完成sink的配置:
从中读取文件的目录
a1.sources.s1.spoolDir=/root/flume/spooling/
指定要忽略的文件的正则表达式 如以.tmp结尾的
a1.sources.s1.ignorePattern=([^ ]*.tmp) ignorePattern与includePattern的官网配置如下:
大意是: includePattern:指定要包含哪些文件的正则表达式。 ignorePattern:指定要跳过(忽略)哪些文件的正则表达式。 如果两者同时匹配的情况下,则忽略改文件 紧接着启动flume服务: bin/flume-ng agent -c conf -f conf/filter-mem-hdfs.properties -n a1 -Dflume.root.logger=INFO,console 接下来可以通过向spooling目录下放置.tmp文件与.log文件对比文件是否重命名。
七、Flume的扇入和扇出
扇入:将多个flume agent采集到的数据,全部传递给某一个flume collect, 然collect再将数据传递给HDFS。 优点:避免HDFS的磁盘负载过高,影响读写操作 使用的组件: ① Flume Agent(slave1,slave2): Source:exec Channel:mem Sink:avro ② Flume Collect(master): Source:avro Channel:mem Sink:hdfs Slave节点的Flume配置如下: #define a agent a1.sources = s1 a1.channels = c1 a1.sinks = k1
define source
a1.sources.s1.type = exec a1.sources.s1.command = tail -F /root/flume/avro-agent.txt a1.sources.s1.shell = /bin/sh -c
define channel
a1.channels.c1.type = memory a1.channels.c1.capacity = 1000 a1.channels.c1.transactionCapacity = 100
define sink
a1.sinks.k1.type = avro #指定sink的hostname地址 a1.sinks.k1.hostname = master #指定sink地址接收的port端口号 a1.sinks.k1.port = 50505 #zuhe source sink channel a1.sources.s1.channels = c1 a1.sinks.k1.channel = c1
Master节点的Flume配置如下: #define a agent a1.sources = s1 a1.channels = c1 a1.sinks = k1
define source
a1.sources.s1.type = avro #绑定自身 代表由slave1,slave2采集的数据由master接收 a1.sources.s1.bind = master #设置端口 a1.sources.s1.port = 50505
define channel
a1.channels.c1.type = memory a1.channels.c1.capacity = 100 a1.channels.c1.transactionCapacity = 100
define sink
a1.sinks.k1.type = hdfs a1.sinks.k1.hdfs.path = /flume/avro a1.sinks.k1.hdfs.fileType = DataStream #zuhe source sink channel a1.sources.s1.channels = c1 a1.sinks.k1.channel = c1 接下来分别启动三台节点上的Flume服务: #master节点启动的服务 bin/flume-ng agent -c conf -f conf/avro-collect.properties -n a1 -Dflume.root.logger=INFO,console #slave节点启动的服务 bin/flume-ng agent -c conf -f conf/avro-agent.properties -n a1 -Dflume.root.logger=INFO,console 观察日志情况!
扇出:将一份数据源采集到多个不同的目标中 需求:将对应的日志文件采集到两个hdfs的目录上去 注意:一个sink必须对应一个channel Flume的配置如下: a1.sources = s1 a1.channels = c1 c2 a1.sinks = k1 k2
define the source
a1.sources.s1.type = exec a1.sources.s1.command = tail -f /root/flume/a.txt a1.sources.s1.shell = /bin/sh -c
定义第一个channel
a1.channels.c1.type = memory a1.channels.c1.capacity = 1000 a1.channels.c1.transactionCapacity = 100
定义第二个channel
a1.channels.c2.type = memory a1.channels.c2.capacity = 1000 a1.channels.c2.transactionCapacity = 100
定义第一个sink
a1.sinks.k1.type = hdfs a1.sinks.k1.hdfs.path = /flume/hdfs1 a1.sinks.k1.hdfs.filePrefix = hive-log a1.sinks.k1.hdfs.writeFormat = Text a1.sinks.k1.hdfs.fileType = DataStream
定义第二个sink
a1.sinks.k2.type = hdfs a1.sinks.k2.hdfs.path = /flume/hdfs2 a1.sinks.k2.hdfs.filePrefix = hive-log a1.sinks.k2.hdfs.writeFormat = Text a1.sinks.k2.hdfs.fileType = DataStream
bind 绑定环节
a1.sources.s1.channels = c1 c2 a1.sinks.k1.channel = c1 a1.sinks.k2.channel = c2 启动服务: bin/flume-ng agent -c conf -f conf/sinks.properties -n a1 -Dflume.root.logger=INFO,console 查看hdfs上/flume下的对应目录,发现同时出现了hdfs1与hdfs2目录,且两个目录下的文件内容完全一致。扇出结束
八、Taildir Source的使用
Flume中有三种可监控文件或目录的source、分别是Exec Source、Spooling Directory Source和Taildir Source。 Taildir Source是1.7版本的新特性,综合了Spooling Directory Source和Exec Source的优点。 ① Exec:可通过tail -f命令去tail住一个文件,然后实时同步日志到sink ② Spool Dir:可监听一个目录,同步目录中的新文件到sink,被同步完的文件可被立即删除或被打上标记。适合用于同步新文件,但不适合对实时追加日志的文件进行监听并同步。 ③ Tail Dir:可实时监控一批文件,并记录每个文件最新消费位置,agent进程重启后不会有重复消费的问题。 配置如下: #agent_name a1.sources=r1 a1.sinks=k1 a1.channels=c1
source类型
a1.sources.r1.type = TAILDIR
元数据位置
a1.sources.r1.positionFile =/root/flume/bd/taildir/taildir_position_new.json
监控的目录组 可定义多个
a1.sources.r1.filegroups = f1 a1.sources.r1.filegroups.f1=/root/flume/taildir/*.log a1.sources.r1.fileHeader = true #sink的配置 a1.sinks.k1.type = hdfs #hdfs上的文件按照/年月日/时分进行分区 a1.sinks.k1.hdfs.path = /flume/%Y%m%d/%H%M #使用本地时间 a1.sinks.k1.hdfs.useLocalTimeStamp = true #文件前缀 a1.sinks.k1.hdfs.filePrefix = bd #文件后缀 a1.sinks.k1.hdfs.fileSuffix = .log #当达到多少时,临时文件滚动成目标文件 单位字节 128MB a1.sinks.k1.hdfs.rollSize =134217728 #当event的数量达到多少时,滚动为目标文件 a1.sinks.k1.hdfs.rollCount = 0 #hdfs sink间隔多久滚动为目标文件 a1.sinks.k1.hdfs.rollInterval = 60 #基于时间的四舍五入 a1.sinks.k1.hdfs.round = true #四舍五入的值为5 a1.sinks.k1.hdfs.roundValue = 5 #时间单位 分钟 a1.sinks.k1.hdfs.roundUnit = minute #最小副本数量 a1.sinks.k1.hdfs.minBlockReplicas = 1 a1.sinks.k1.hdfs.writeFormat = Text a1.sinks.k1.hdfs.fileType=DataStream #channel的配置 a1.channels.c1.type = memory a1.channels.c1.capacity = 1000 a1.channels.c1.transactionCapacity = 100 #用channel链接source和sink a1.sources.r1.channels = c1 a1.sinks.k1.channel =c1 接下来创建a.log文件,复制到刚刚创建好的taildir目录下。 使用echo命令动态向位于taildir目录下的a.log文件中插入日志。
|