编程目的
- 熟悉 MapReduce 计算引擎
- 初步学习 MapReduce 编程
MapReduce原理学习
位于MapReduce的生态架构的计算层 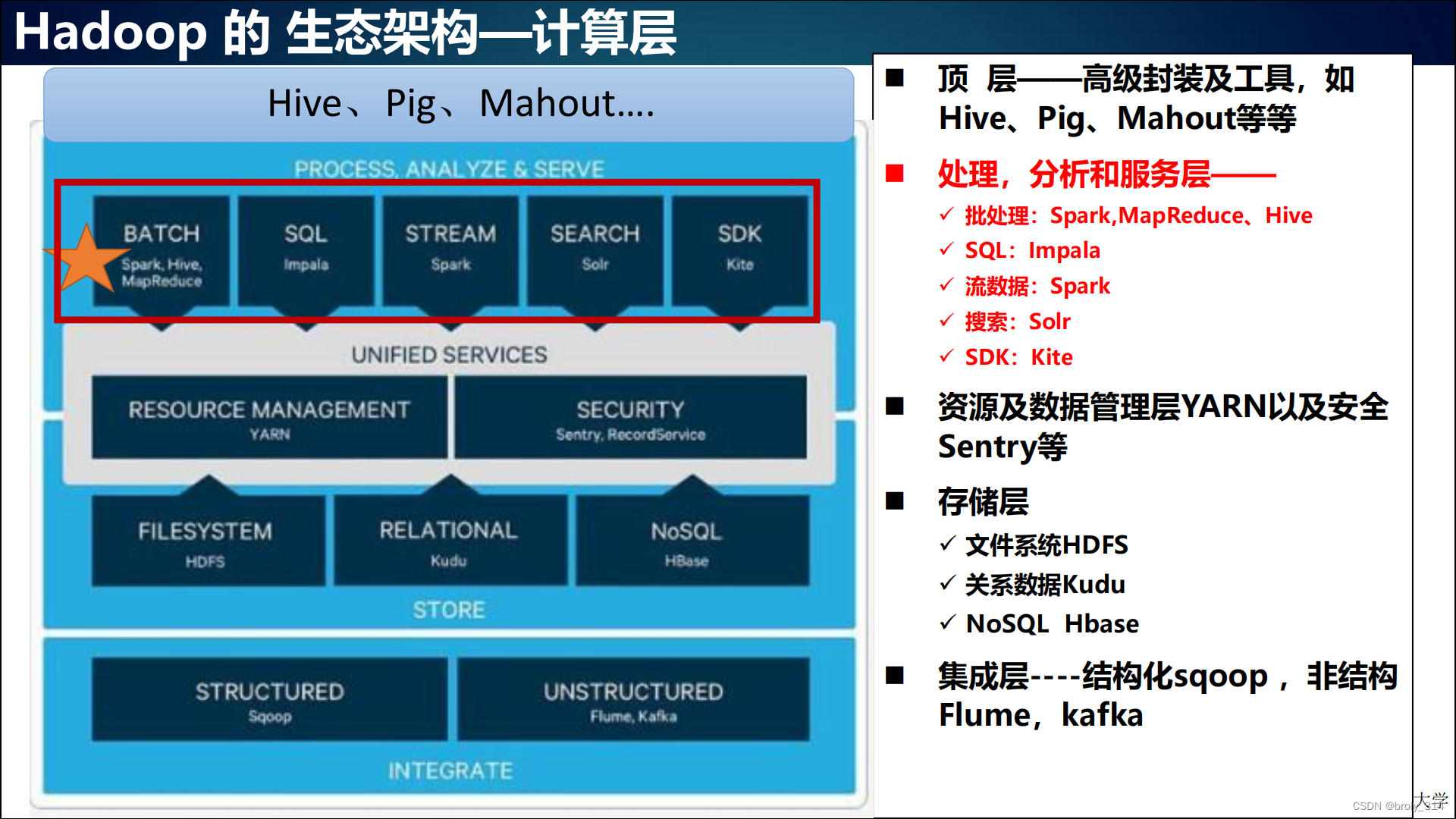 MapReduce是一种分布式计算模型,用以进行大数据量的计算。它屏蔽了分布式计算框架细节,将计算抽象成map和reduce两部分,其中Map对数据集上的独立元素进行指定的操作,生成键-值对形式中间结果。Reduce则对中间 结果相同“键”的所有“值”进行规约,以得到最终结果。MapReduce非常适合在大量计算机组成的分布式并行环境里进行数据处理。
关于MapReduce的了解到这里基本足够了,如想了解更多,这里提供学习链接: lMapReduce进阶学习
MapReduce词频统计编程
编程任务
题目描述: 统计文件集合中每个单词出现的次数。 例如: 文件集合包含两个文件 inputA.txt,inputB.txt。文件内容如下:
inputA.txt:
Hello world
This is WordCount
inputB.txt:
Hello guys
This is MapReduce
输出结果:
Hello 2
MapReduce 1
This 2
WordCount 1
is 2
guys 1
world 1
编程原理
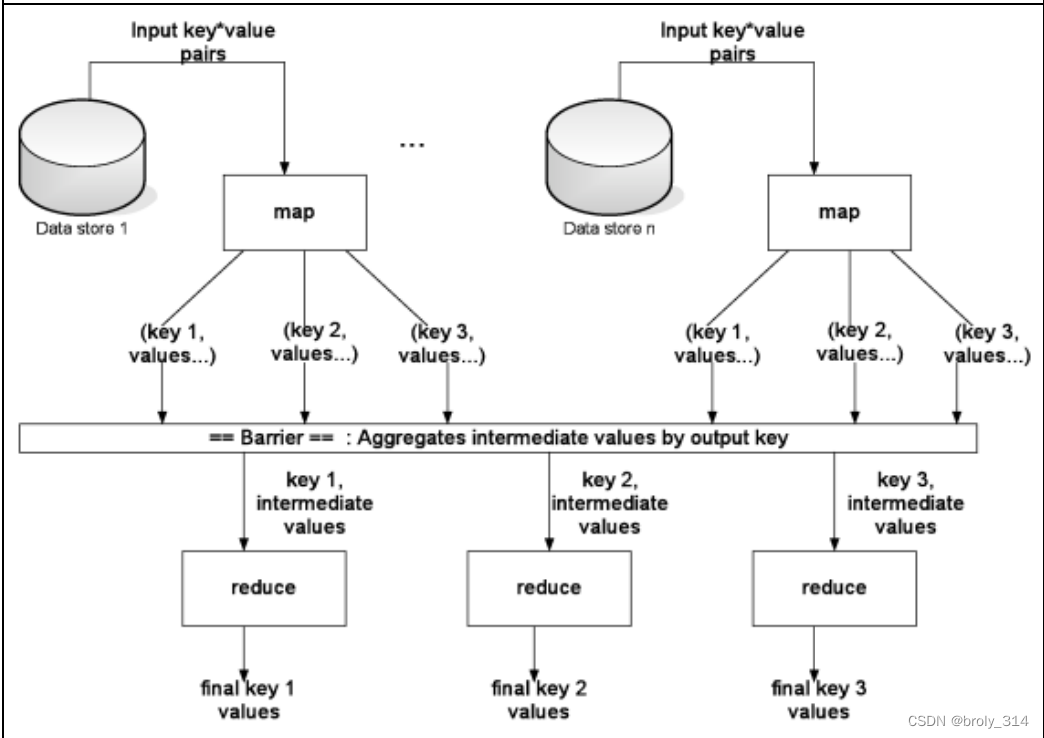 原理:利用一个输入 Key/Value pair 集合来产生一个输出的 Key/Value pair 集合 Map 函数:接受一个输入的 Key/Value pair 值,然后产生一个中间 Key/Value pair 值的集合。 Reduce 函数:接受一个中间 Key 值和相关的一个 Value 值的集合,合并这些 Value 值。
编程步骤
Java分词jar编写
准备工作
使用IEDA创建名字为WordCount的项目文件 ↓ 在WordCount 的 src中创建名为com.sugon.mapred.example ↓ 在包中分别编写三个class文件,名字依次为:TokenizerMapper,IntSumReducer,WordCount
完成后目录文件示例: 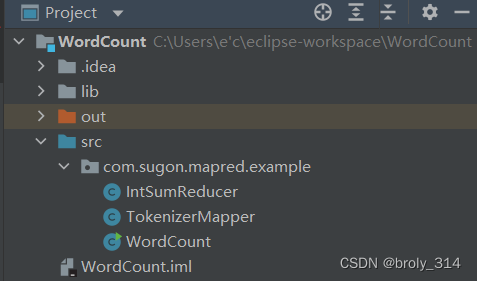 然后导包,因为后续编程需要一些外部包,所以先导入,eclipse 版本的在实验文件里有,这里提供IEDA版本的链接: IDEA导包及打包教程
class文件编写
注:不建议直接复制哈!!自己照着写一写熟悉熟悉内部构造!!!!! TokenizerMapper.java
package com.sugon.mapred.example;
import java.io.IOException;
import java.util.StringTokenizer;
import org.apache.hadoop.io.IntWritable;
import org.apache.hadoop.io.Text;
import org.apache.hadoop.mapreduce.Mapper;
public class TokenizerMapper extends Mapper<Object, Text, Text,IntWritable>{
IntWritable one = new IntWritable(1);
Text word = new Text();
protected void map(Object key, Text value, Mapper<Object, Text, Text, IntWritable>.Context context) throws IOException, InterruptedException {
StringTokenizer itr = new StringTokenizer(value.toString());
while (itr.hasMoreTokens()){
word.set(itr.nextToken());
context.write(word, one);
}
}
}
IntSumReducer.java
package com.sugon.mapred.example;
import java.io.IOException;
import org.apache.hadoop.io.IntWritable;
import org.apache.hadoop.io.Text;
import org.apache.hadoop.mapreduce.Reducer;
public class IntSumReducer extends Reducer<Text, IntWritable, Text, IntWritable>{
IntWritable result = new IntWritable();
protected void reduce(Text key, Iterable<IntWritable> values, Reducer<Text, IntWritable, Text, IntWritable>.Context context) throws IOException, InterruptedException {
int sum = 0;
for (IntWritable val: values){
sum += val.get();
}
result.set(sum);
context.write(key, result);
}
}
WordCount.java
package com.sugon.mapred.example;
import org.apache.hadoop.conf.Configuration;
import org.apache.hadoop.fs.Path;
import org.apache.hadoop.io.IntWritable;
import org.apache.hadoop.io.Text;
import org.apache.hadoop.mapreduce.Job;
import org.apache.hadoop.mapreduce.lib.input.FileInputFormat;
import org.apache.hadoop.mapreduce.lib.output.FileOutputFormat;
import org.apache.hadoop.util.GenericOptionsParser;
public class WordCount {
public static void main(String[] args) throws Exception{
Configuration conf = new Configuration();
String[] otherArgs = new GenericOptionsParser(conf, args).getRemainingArgs();
if (otherArgs.length != 2){
System.err.println("usage: wordcount <in> <out>");
System.exit(2);
}
Job job = Job.getInstance(conf, "wordcount");
job.setJarByClass(WordCount.class);
job.setMapperClass(TokenizerMapper.class);
job.setCombinerClass(IntSumReducer.class);
job.setReducerClass(IntSumReducer.class);
job.setOutputKeyClass(Text.class);
job.setOutputValueClass(IntWritable.class);
FileInputFormat.addInputPath(job, new Path(otherArgs[0]));
FileOutputFormat.setOutputPath(job, new Path(otherArgs[1]));
System.exit(job.waitForCompletion(true)?0:1);
}
}
写完后打包,打包方法:IDEA导包及打包教程
打包后的样子: 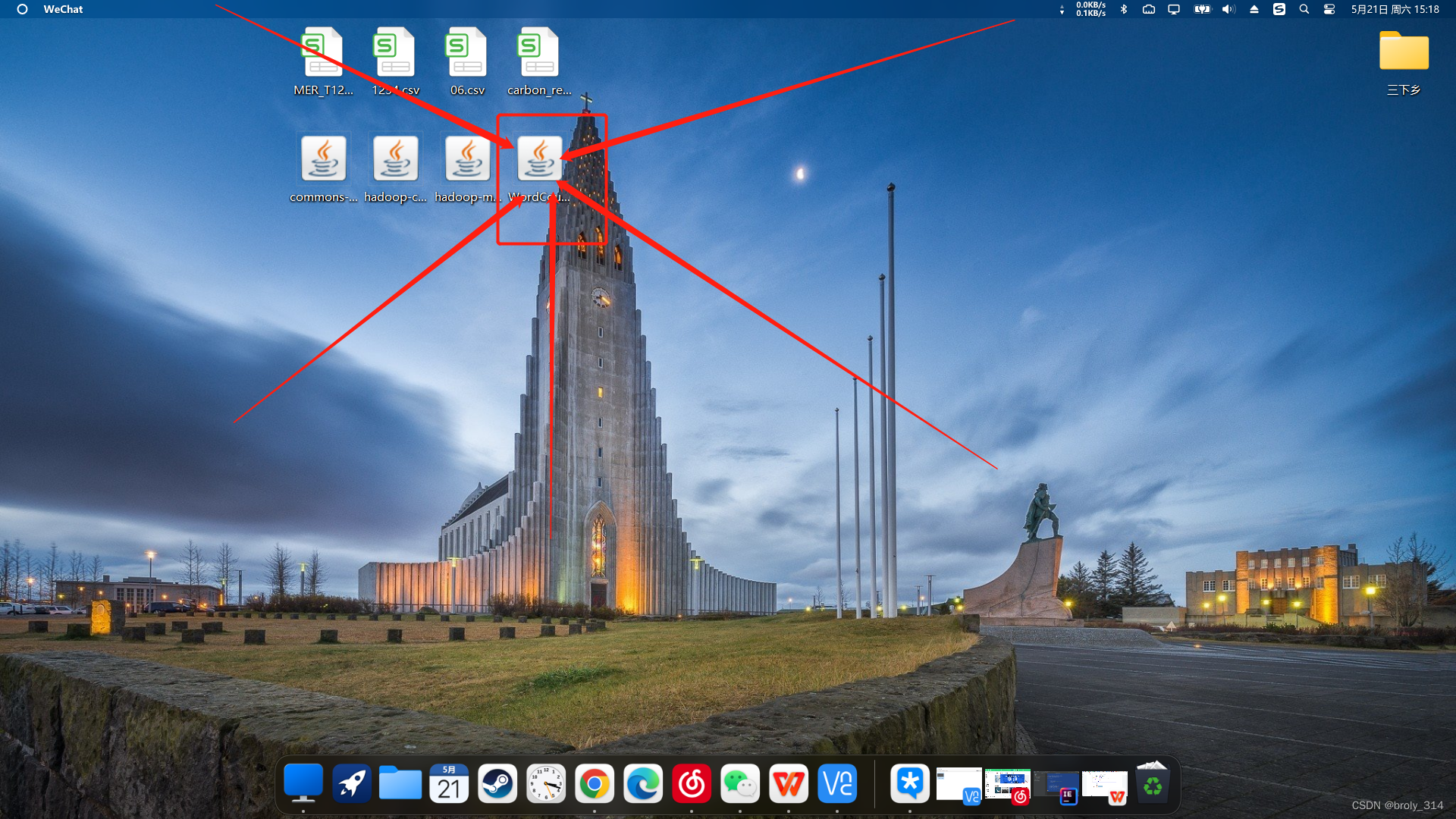
MapReduce编程文件准备
进入第二部分,这里的主要任务就是把刚才的包上传到曙光大数据平台中,以及准备需要统计词频的txt。
jar包上传
进入VNC,点击屏幕上方正中间,选取File transfer 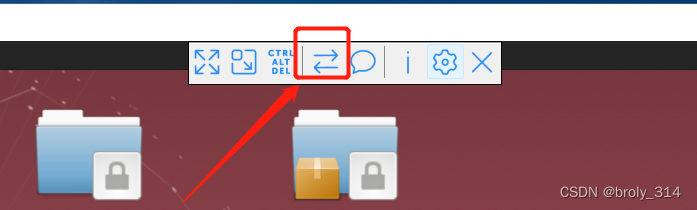 send files 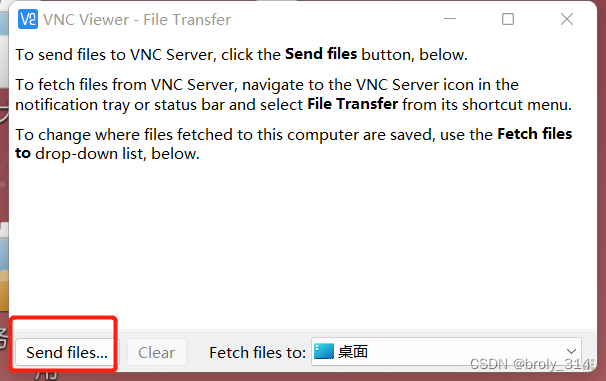 找到打包好的jar的路径 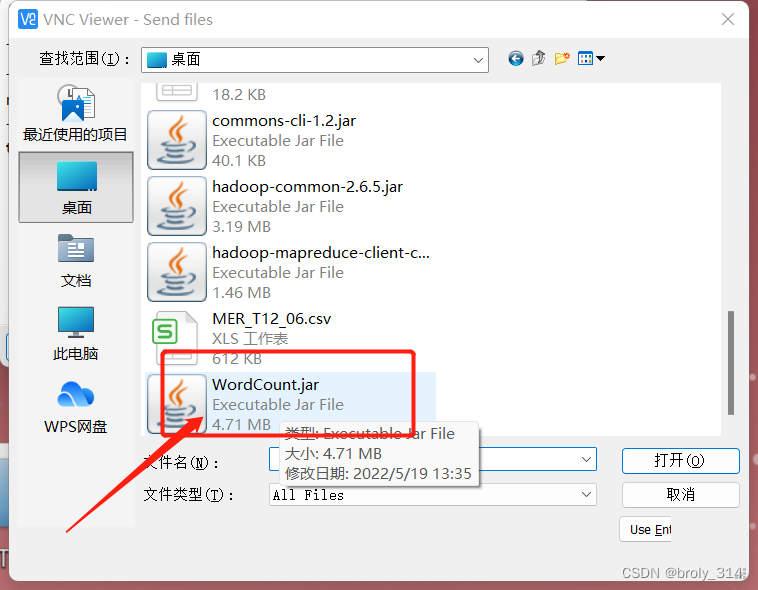
词频统计文本
创建data文件夹,并在其中创建inputA.txt, inputB.txt文本文件 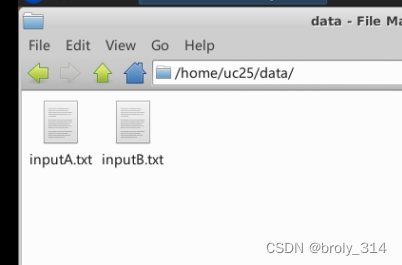 分别写入(可自定义): 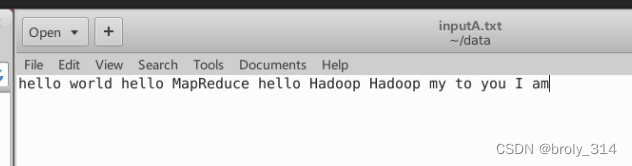 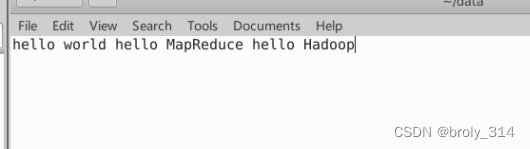
MapReduce词频统计
上传文件到hdfs中
创建一个 ./data.input hdfs文件夹,用以存放数据 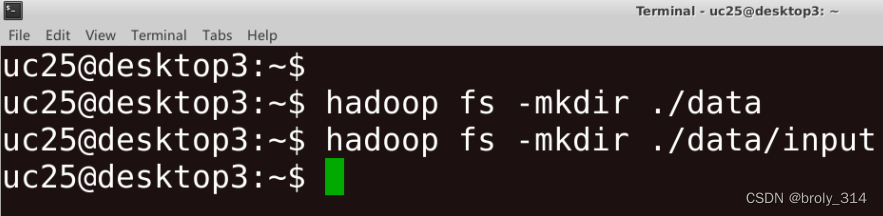 将之前data文件中的txt文件上传到hdfs文件中:  创建./wordcount hdfs文件夹,并上传自己的jar包到其中:  开始使用MapReduce 词频统计  查看分词结果: 其中的output_zhc便是我们输出的结果  打印一下: 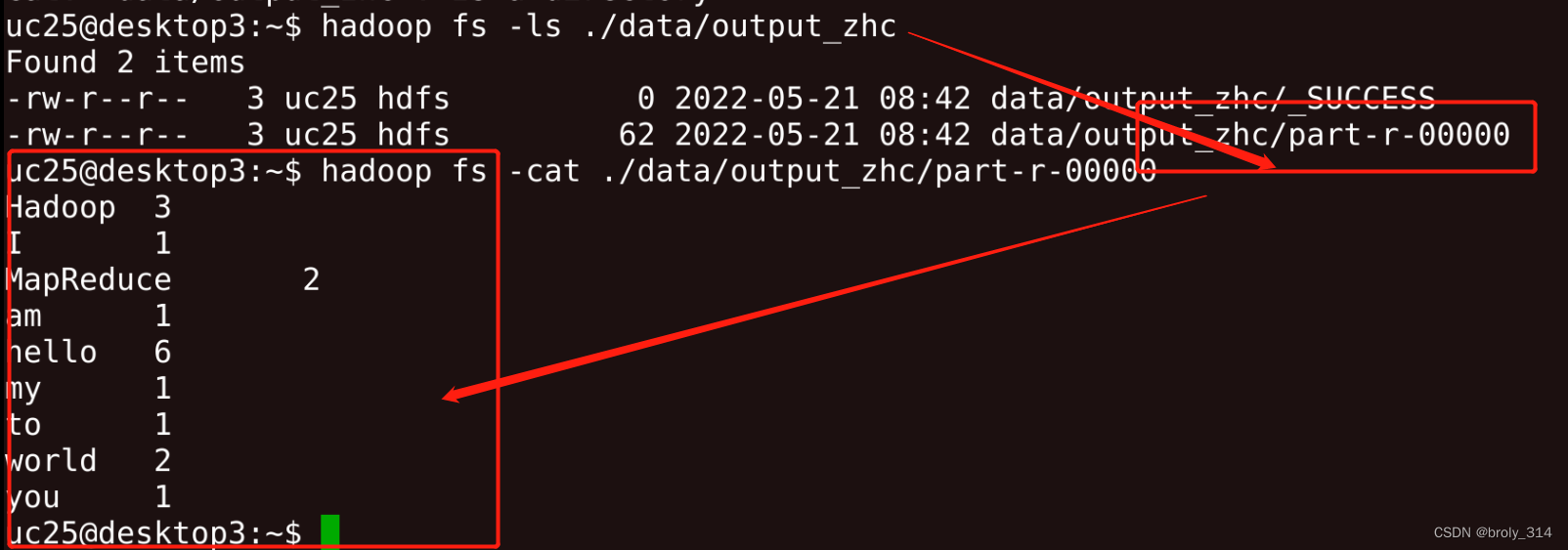 ok,词频统计基本编程结束学习差不多结束了。
总结
收获
了解了MapReduce的原理,知道它在大量数据下的优点,并掌握了MApReduce的一些基本使用方法以及Hadoop的一些操作原理及指令。更深一步的认知了大数据这门学科。
遇到的困难
自己写的java代码跑不出来,实验都是用的老师给的jar包,在java编程能力上还有待提高!
|