目录
概述
本地模式
新建一个maven项目
新建三个包
写mapper组件
Reducer组件
Driver组件
运行结果
?编辑
集群模式
maven一劳永逸
?修改pom文件
添加mapper、reducer和driver三个组件
准备用于单词计数的文件夹和文件
?将文件放到dfs?编辑
项目本地进行调试
?项目打jar包
把jar包上传到centos下
复制主类运行
?查看运行过程和结果
三个教材上的案例
?编辑
dedup 去重
Driver
mapper
reducer
成功运行
?invertedIndex倒序索引
?Combiner
Driver
reducer
?运行成功
?topN
?Driver
mapper
reducer
?运行成功
概述
MapReduce是Hadoop系统核心组件之一,它是一种可用于大数据并行处理的计算模型、框架和平台,主要解决海量数据的计算,是目前分布式计算模型中应用较为广泛的一种。
MapReduce核心思想
MapReduce的核心思想是“分而治之”。所谓“分而治之”就是把一个复杂的问题,按照一定的“分解”方法分为等价的规模较小的若干部分,然后逐个解决,分别找出各部分的结果,把各部分的结果组成整个问题的结果,这种思想来源于日常生活与工作时的经验,同样也完全适合技术领域。
本地模式
新建一个maven项目
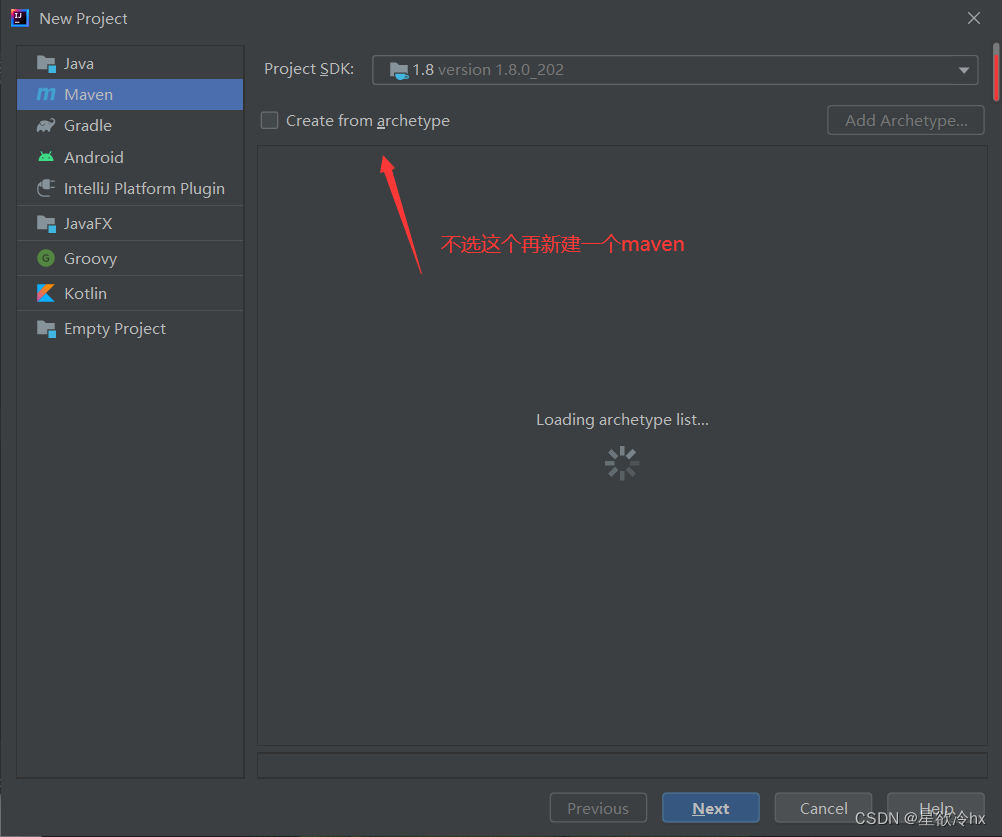
?修改maven仓库为本地镜像
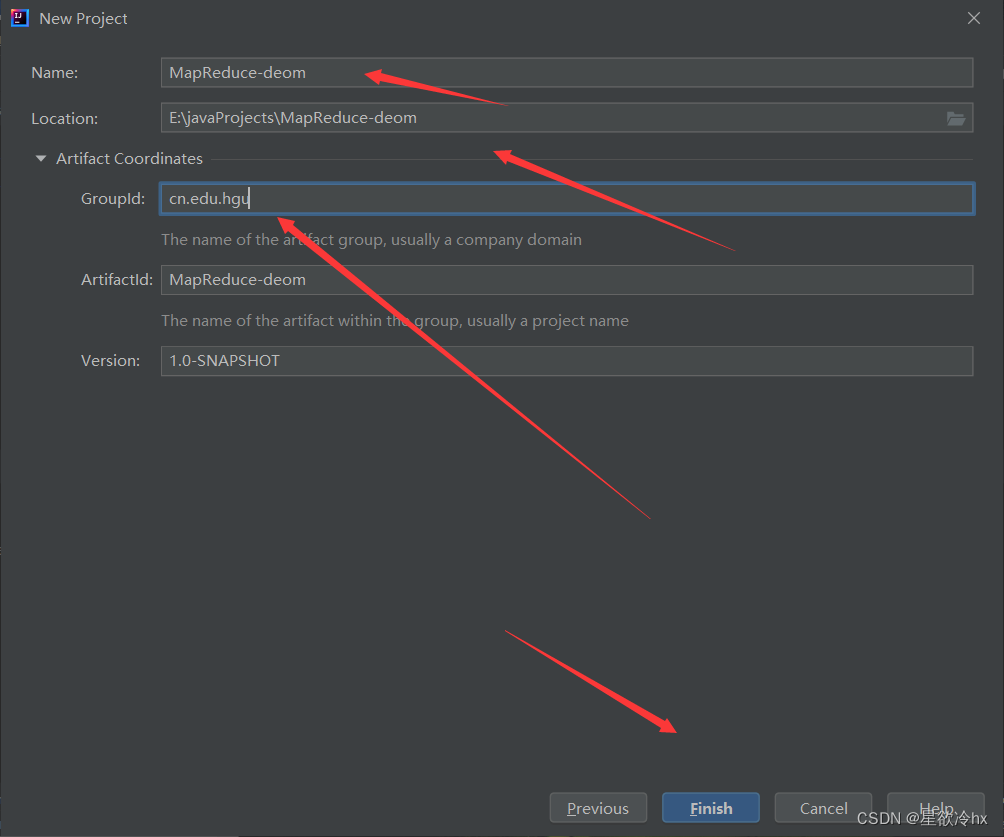
?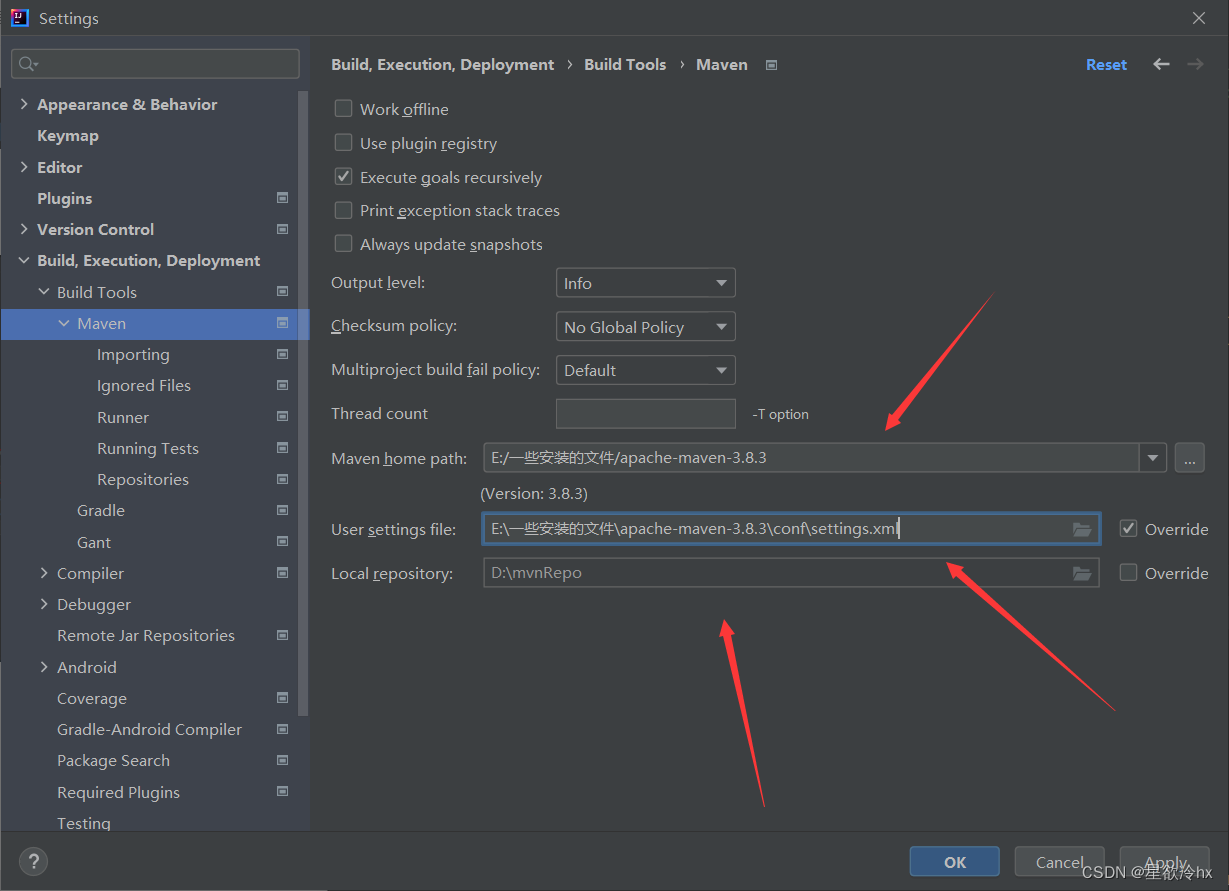
?添加maven依赖
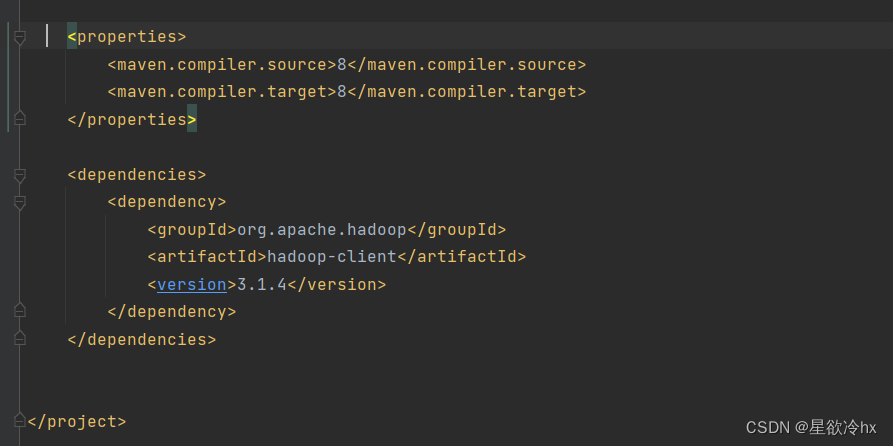
?复制源码
<dependencies>
<dependency>
<groupId>org.apache.hadoop</groupId>
<artifactId>hadoop-client</artifactId>
<version>3.1.4</version>
</dependency>
</dependencies>
新建三个包
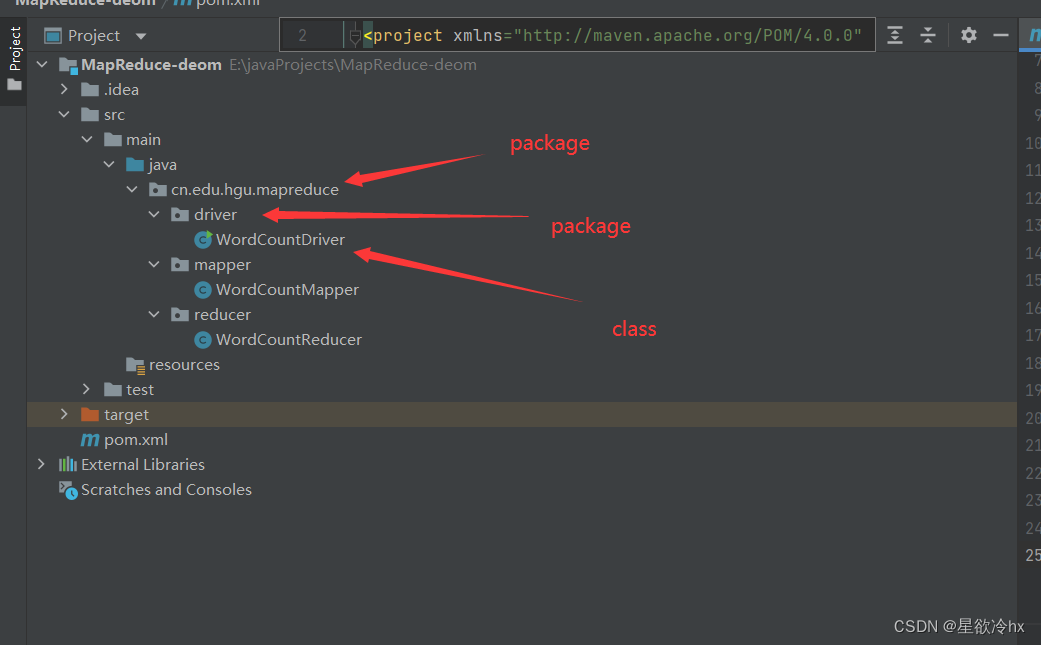
写mapper组件
import org.apache.hadoop.io.IntWritable;
import org.apache.hadoop.io.LongWritable;
import org.apache.hadoop.io.Text;
import org.apache.hadoop.mapreduce.Mapper;
import java.io.IOException;
public class WordCountMapper extends Mapper<LongWritable, Text, Text, IntWritable> {
@Override
protected void map(LongWritable key, Text value, Mapper<LongWritable, Text, Text, IntWritable>.Context context) throws IOException, InterruptedException {
// 接收传入的一行文本
String line = value.toString();
// 把一行文本分割成一个个的单词
String[] words = line.split(" ");
// 把每个单词组成<单词,1>二元组k-v格式输出,作为reducer的输入
for (String word : words) {
context.write(new Text(word), new IntWritable(1));
}
}
}
Reducer组件
在写reduce组件时protect void reducer应该没有r
package cn.edu.hgu.mapreduce.reducer;
import org.apache.hadoop.io.IntWritable;
import org.apache.hadoop.mapreduce.Reducer;
import org.apache.hadoop.io.Text;
import java.io.IOException;
public class WordCountReducer extends Reducer<Text, IntWritable, Text, IntWritable> {
protected void reduce(Text key, Iterable<IntWritable> value, Reducer<Text, IntWritable, Text, IntWritable>.Context context) throws IOException, InterruptedException {
// 定义一个计数器
int count = 0;
// 对迭代器做累加的操作
for (IntWritable i : value) {
// get()获取IntWritable整型值
count = count + i.get();
}
// 输出
context.write(key, new IntWritable(count));
}
}
Driver组件
package cn.edu.hgu.mapreduce.driver;
import cn.edu.hgu.mapreduce.mapper.WordCountMapper;
import cn.edu.hgu.mapreduce.reducer.WordCountReducer;
import org.apache.hadoop.conf.Configuration;
import org.apache.hadoop.fs.Path;
import org.apache.hadoop.io.IntWritable;
import org.apache.hadoop.io.Text;
import org.apache.hadoop.mapreduce.Job;
import org.apache.hadoop.mapreduce.lib.input.FileInputFormat;
import org.apache.hadoop.mapreduce.lib.output.FileOutputFormat;
import java.io.IOException;
public class WordCountDriver {
public static void main(String[] args) throws IOException, ClassNotFoundException, InterruptedException {
Configuration conf = new Configuration();
// 设置mapreduce的运行模式,为本地模式
conf.set("mapreduce.framework.name","local");
// 创建mapreduce的job
Job job = Job.getInstance(conf);
// 开始装配各个组件
// 指定job的运行主类
job.setJarByClass(WordCountDriver.class);
// 指定job的mapper组件
job.setMapperClass(WordCountMapper.class);
// 指定job的reduce组件
job.setReducerClass(WordCountReducer.class);
// 设置Mapper类的key和value的数据类型
job.setMapOutputKeyClass(Text.class);
job.setMapOutputValueClass(IntWritable.class);
// 设置reduce类的输出阶段的key和value的数据类型
job.setOutputKeyClass(Text.class);
job.setOutputValueClass(IntWritable.class);
// 指定本地模式的数据源
FileInputFormat.setInputPaths(job, "E:/wordcount/input");
// 指定本地模式的输出目的的
FileOutputFormat.setOutputPath(job,new Path("E:/wordcount/output"));
// 提交程序运行
boolean result = job.waitForCompletion(true);
System.exit(result ? 0 : 1);
}
}
运行结果
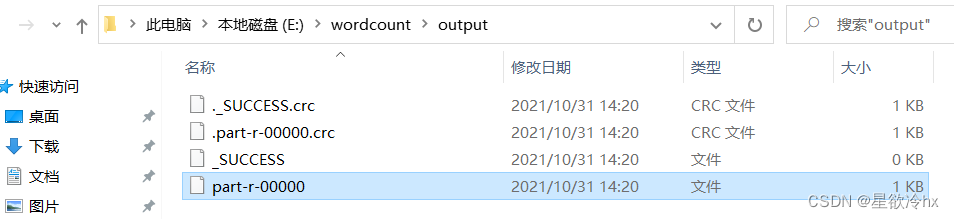
?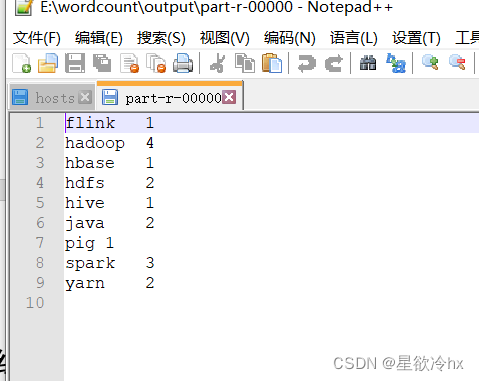
集群模式
要把项目打成jar包,然后提交到hadoop集群上运行
maven一劳永逸
如果想一劳永逸而不每次都配置maven
就要用到以下操作
当然如果你找不到这个界面也可以去最左上角然后下拉找到setting
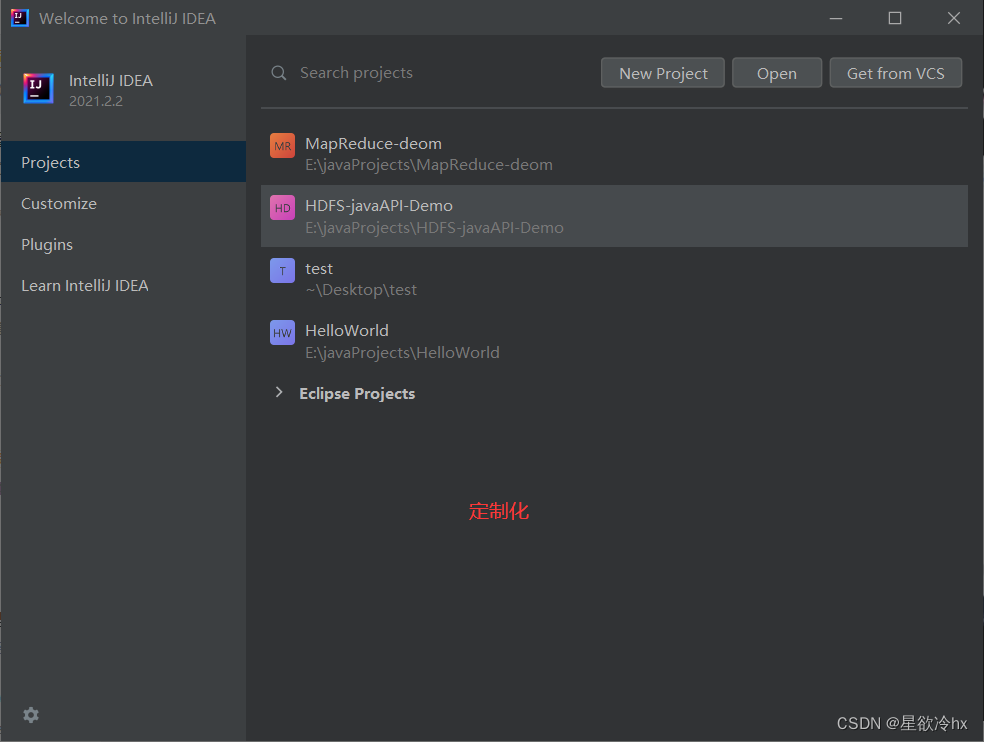
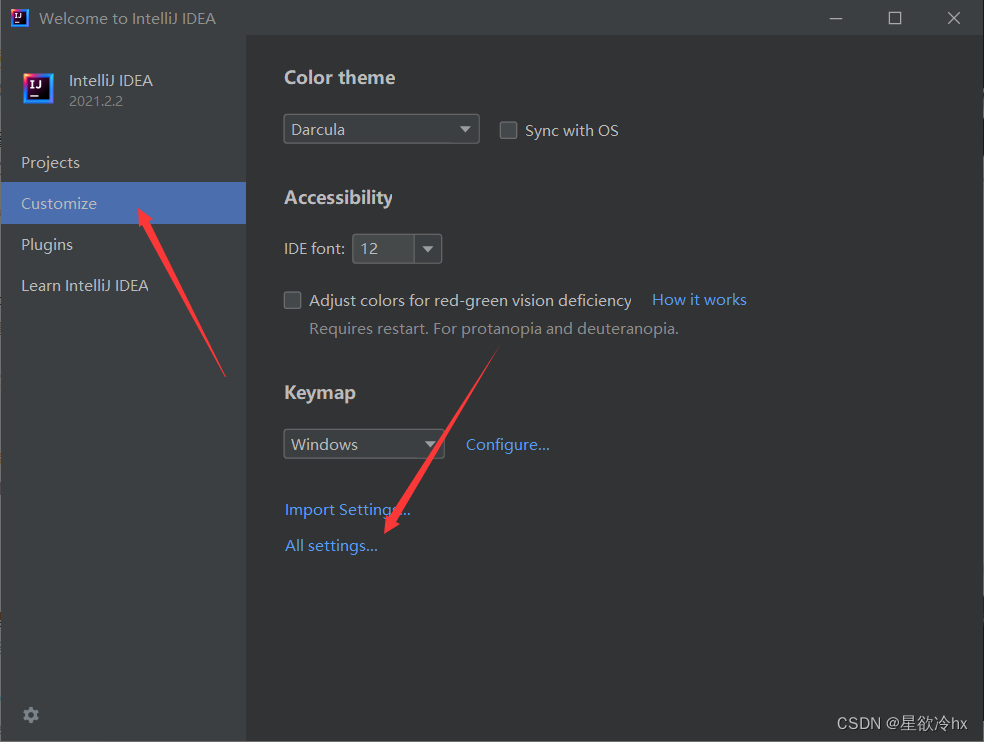
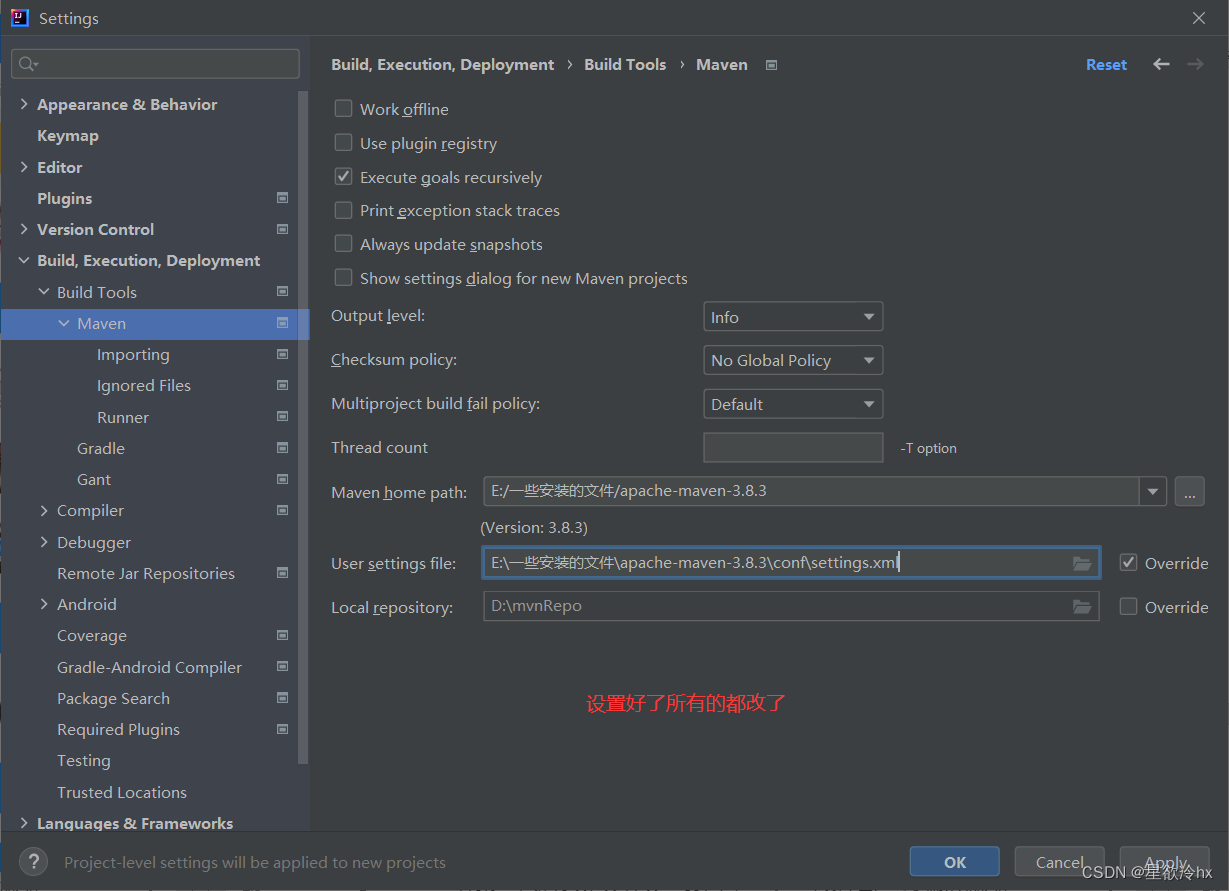
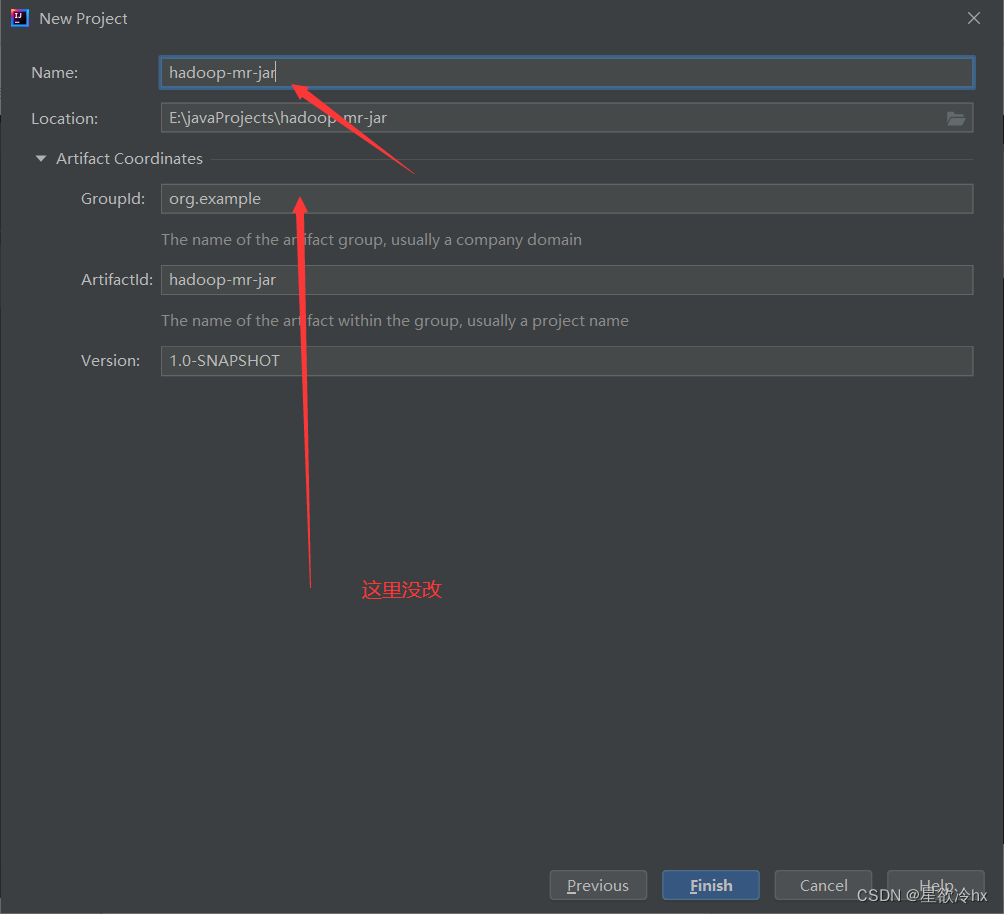
?修改pom文件
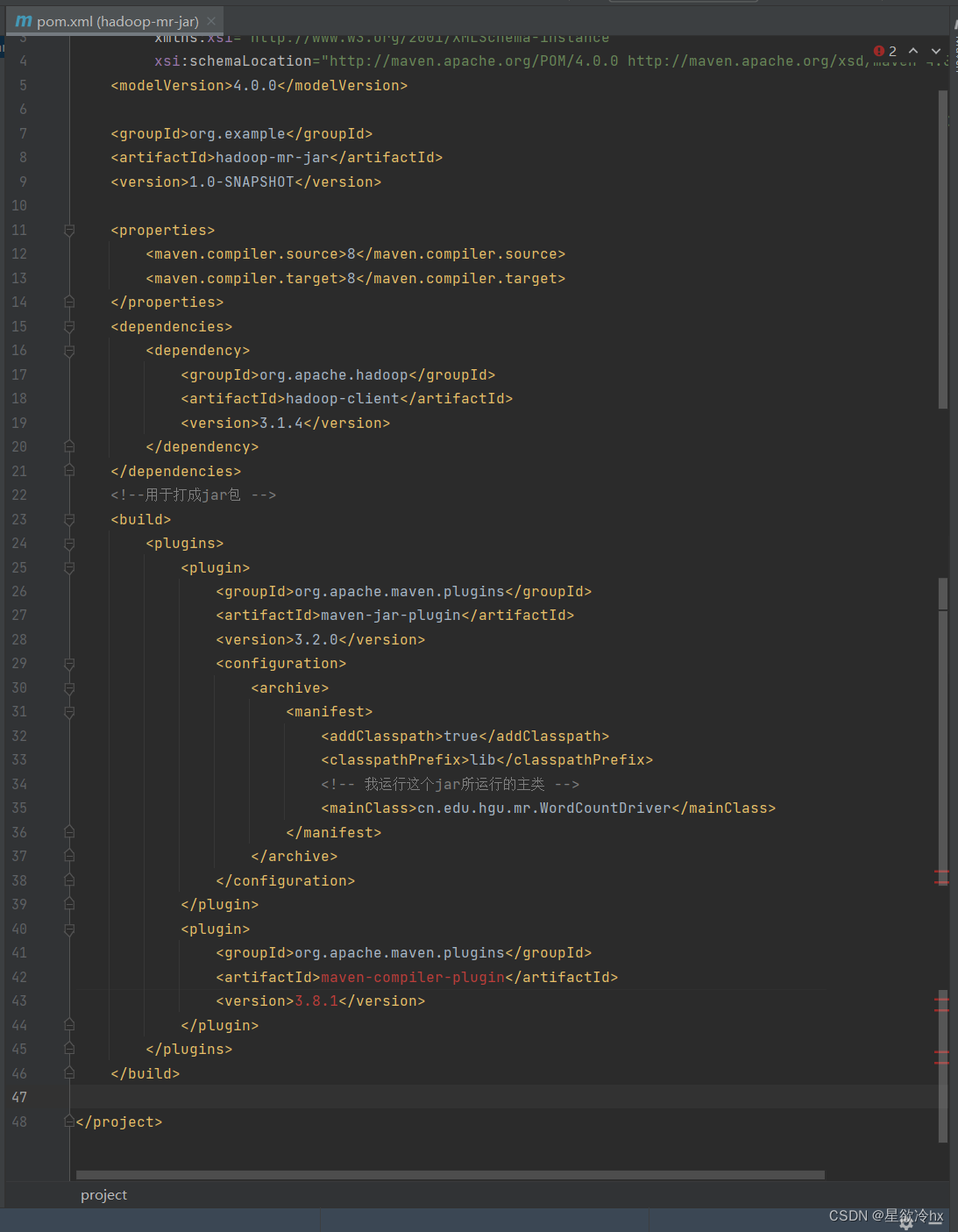
<dependencies>
<dependency>
<groupId>org.apache.hadoop</groupId>
<artifactId>hadoop-client</artifactId>
<version>3.1.4</version>
</dependency>
</dependencies>
<!--用于打成jar包 -->
<build>
<plugins>
<plugin>
<groupId>org.apache.maven.plugins</groupId>
<artifactId>maven-jar-plugin</artifactId>
<version>3.2.0</version>
<configuration>
<archive>
<manifest>
<addClasspath>true</addClasspath>
<classpathPrefix>lib</classpathPrefix>
<!-- 我运行这个jar所运行的主类 -->
<mainClass>cn.edu.hgu.mr.WordCountDriver</mainClass>
</manifest>
</archive>
</configuration>
</plugin>
<plugin>
<groupId>org.apache.maven.plugins</groupId>
<artifactId>maven-compiler-plugin</artifactId>
<version>3.8.1</version>
</plugin>
</plugins>
</build>
添加mapper、reducer和driver三个组件
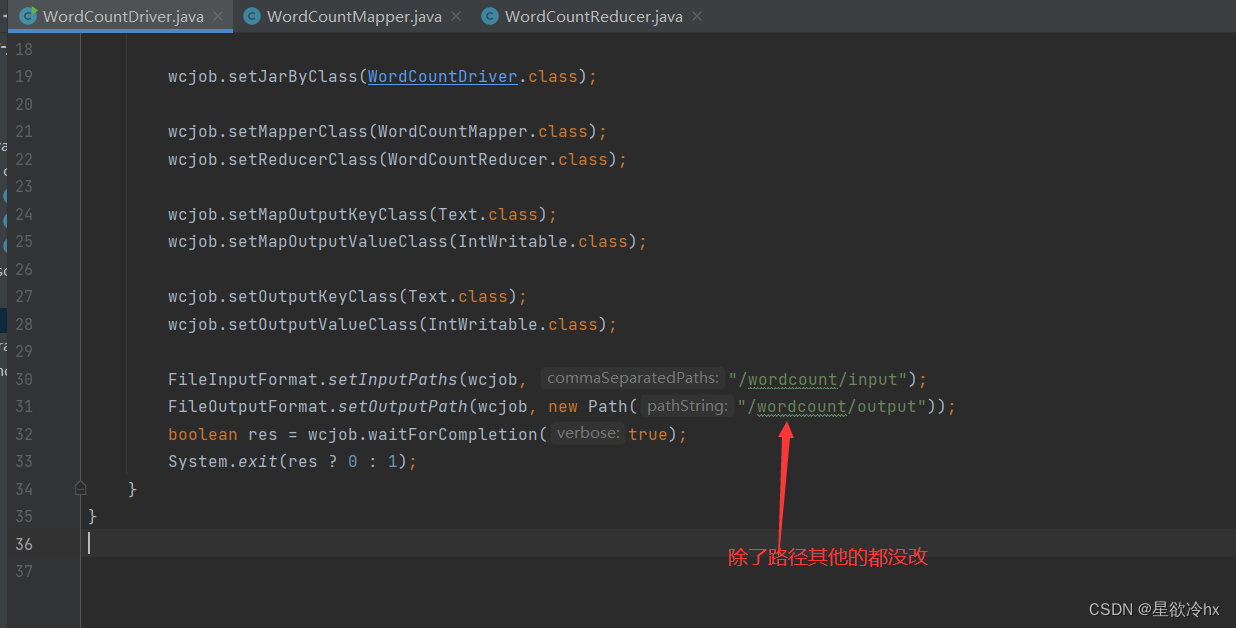
准备用于单词计数的文件夹和文件
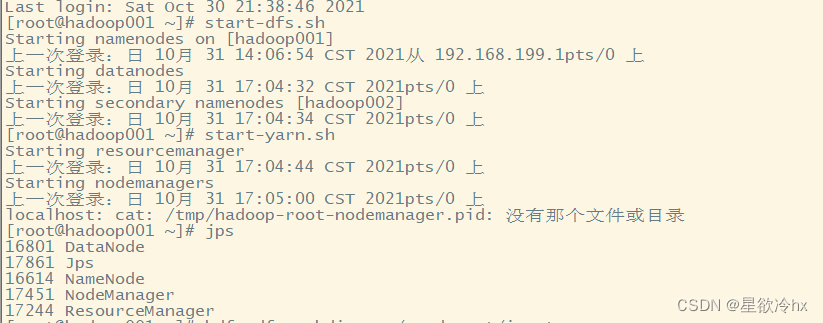
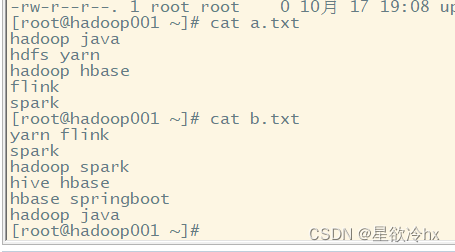

?将文件放到dfs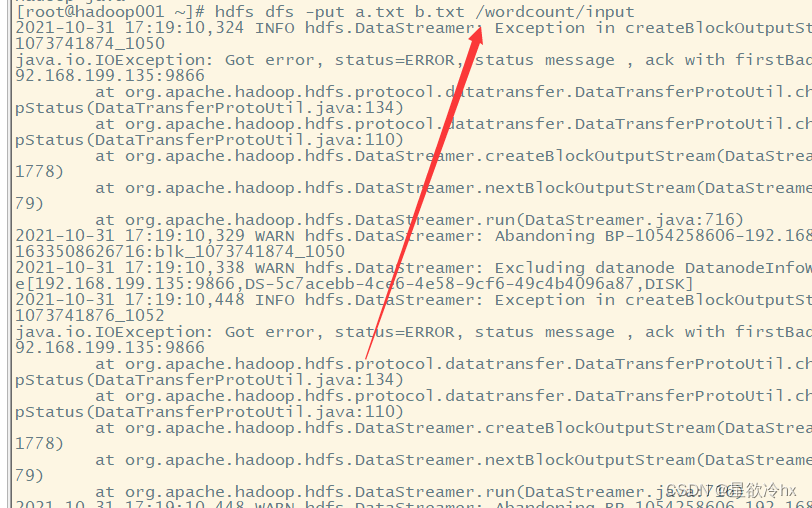
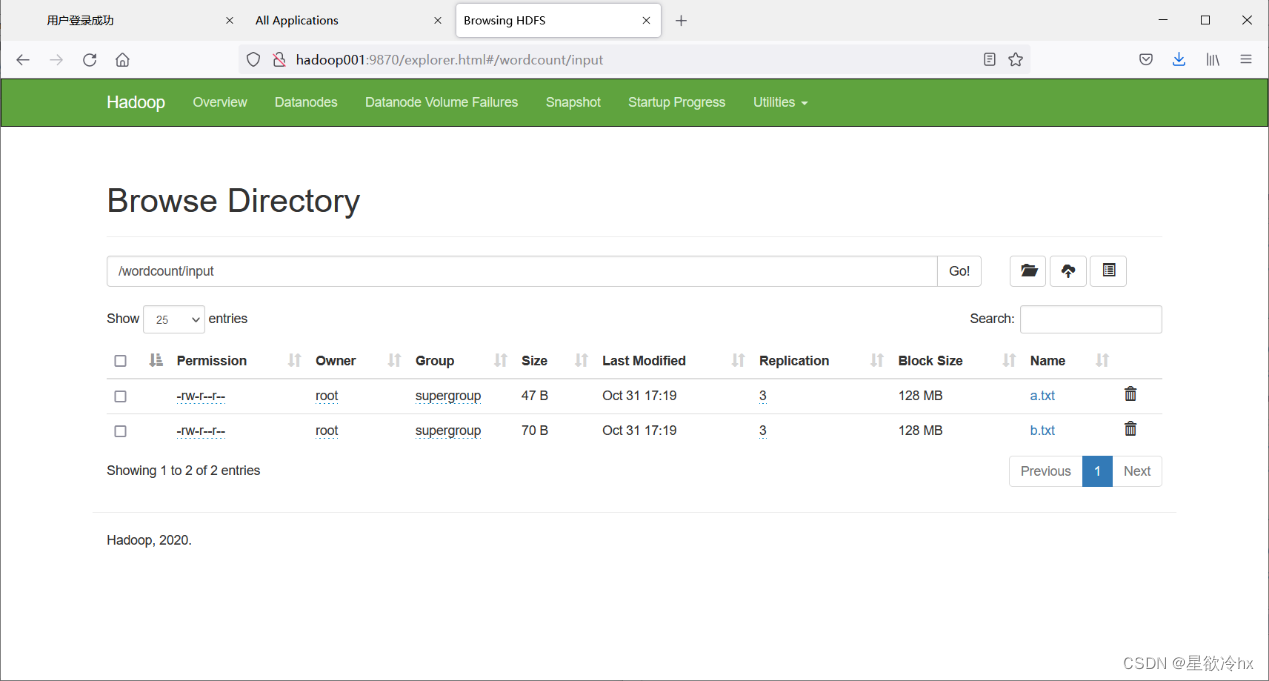
项目本地进行调试
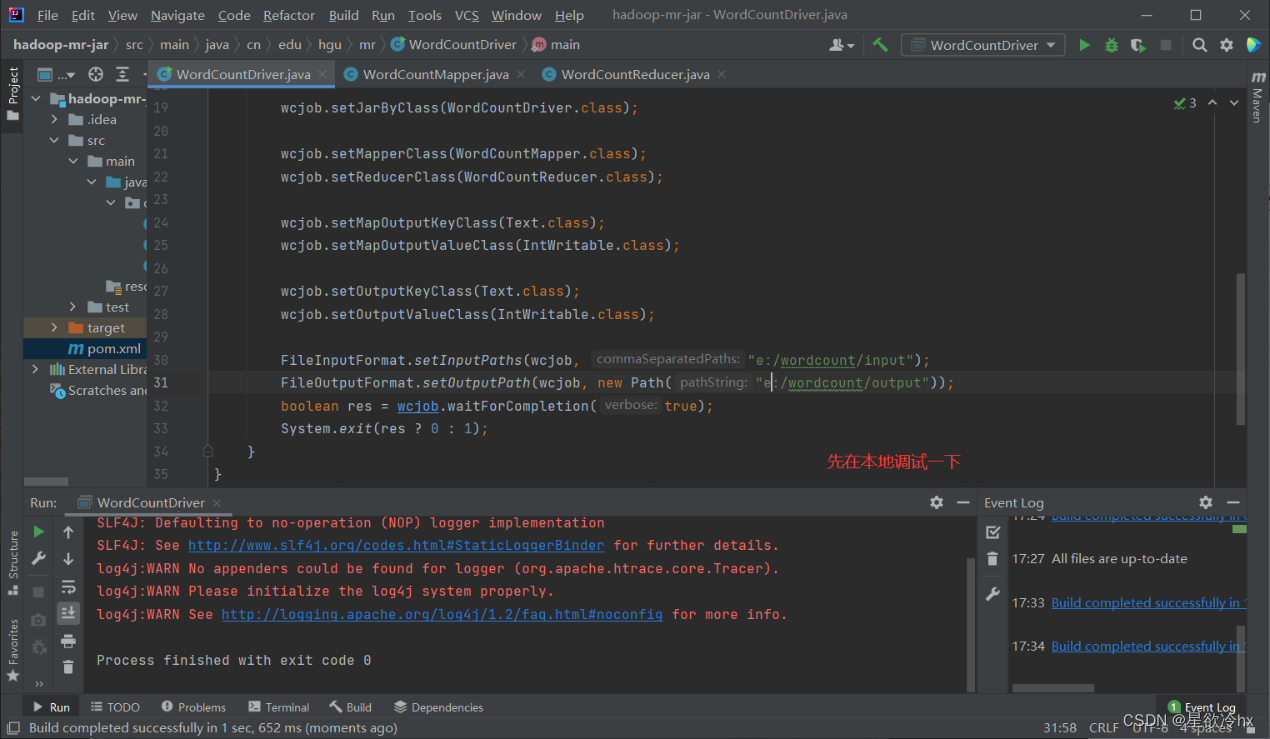
?项目打jar包
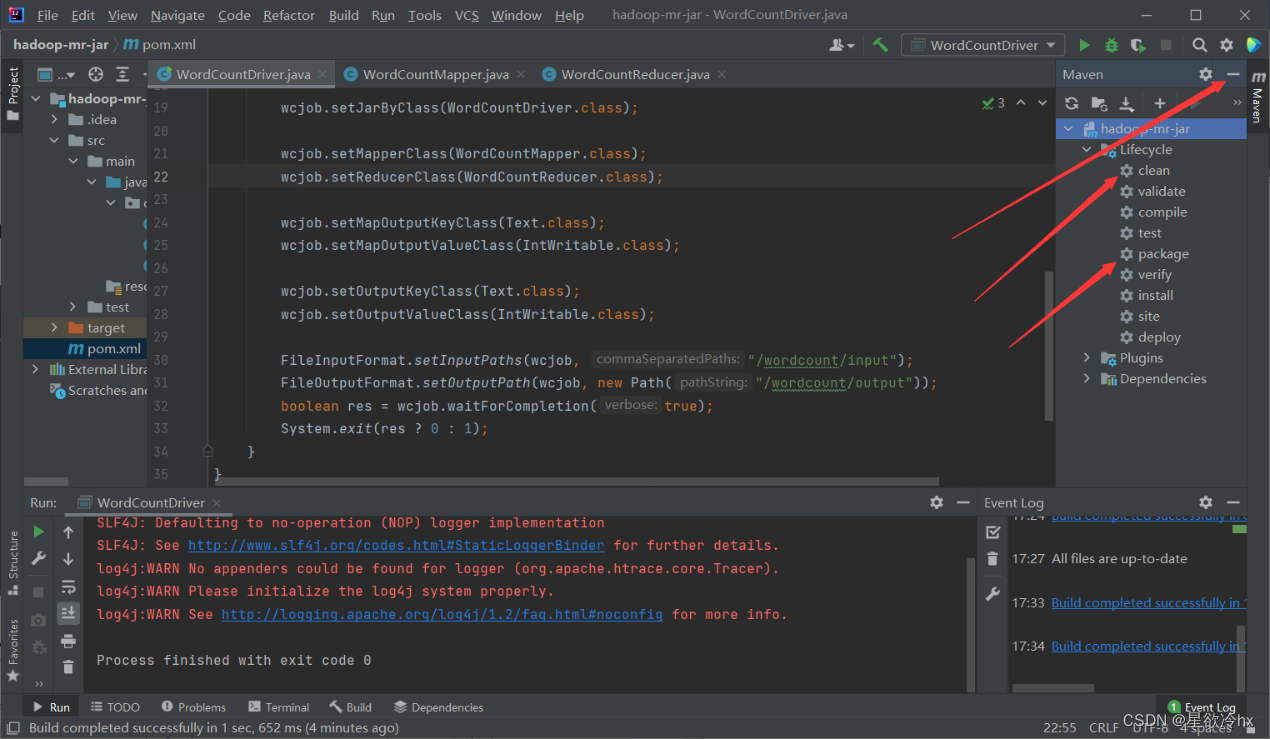
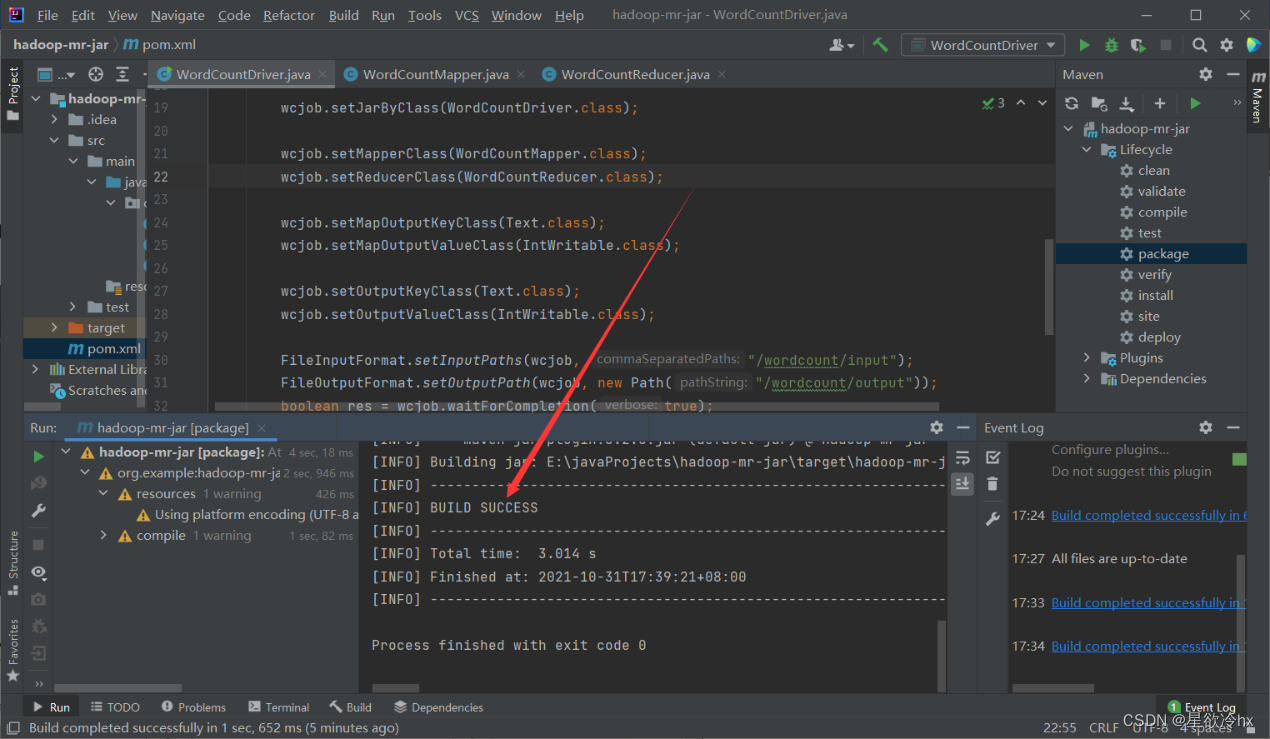
我们可以看到打完包之后左面有许多警告,再试一次
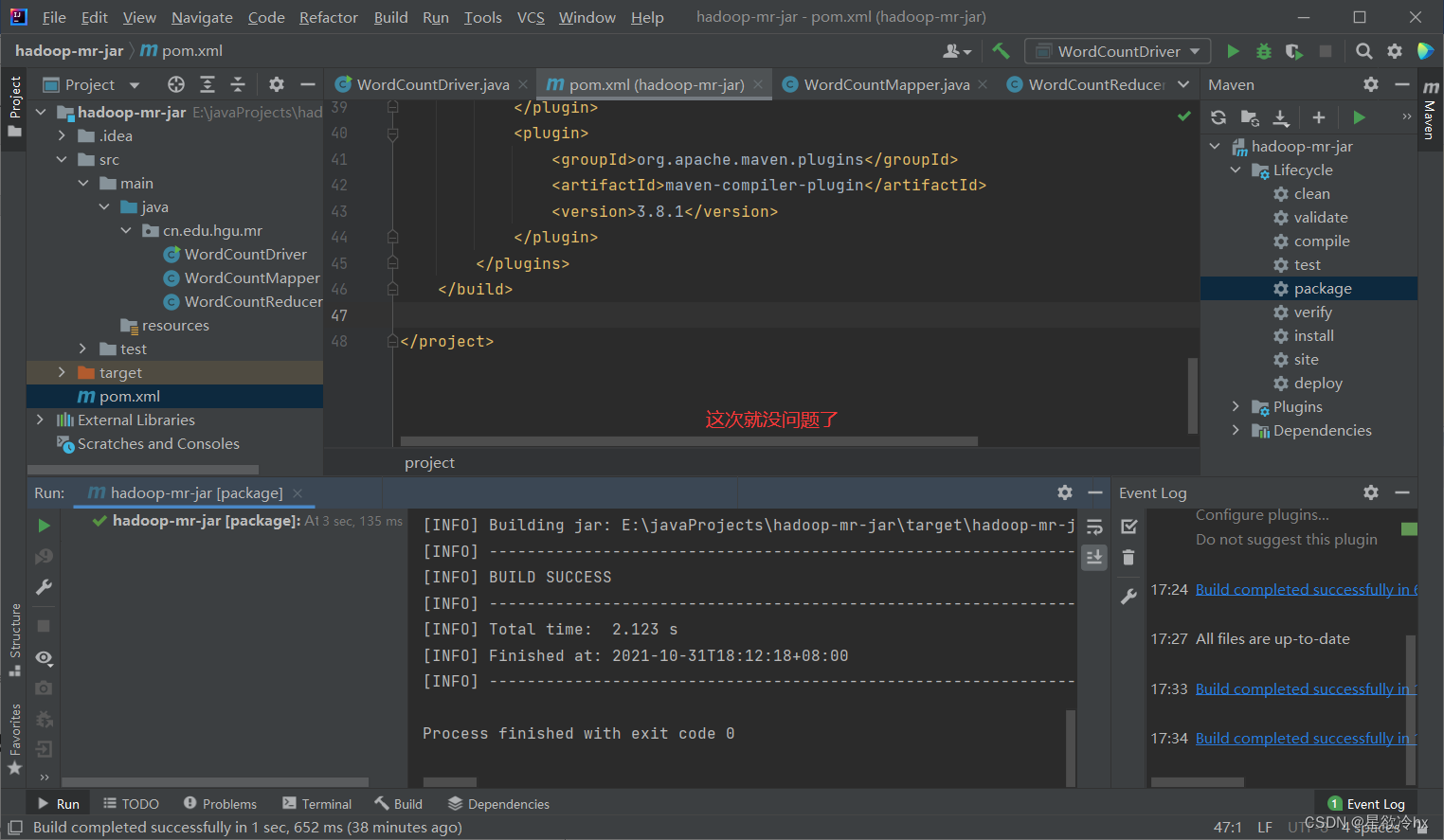
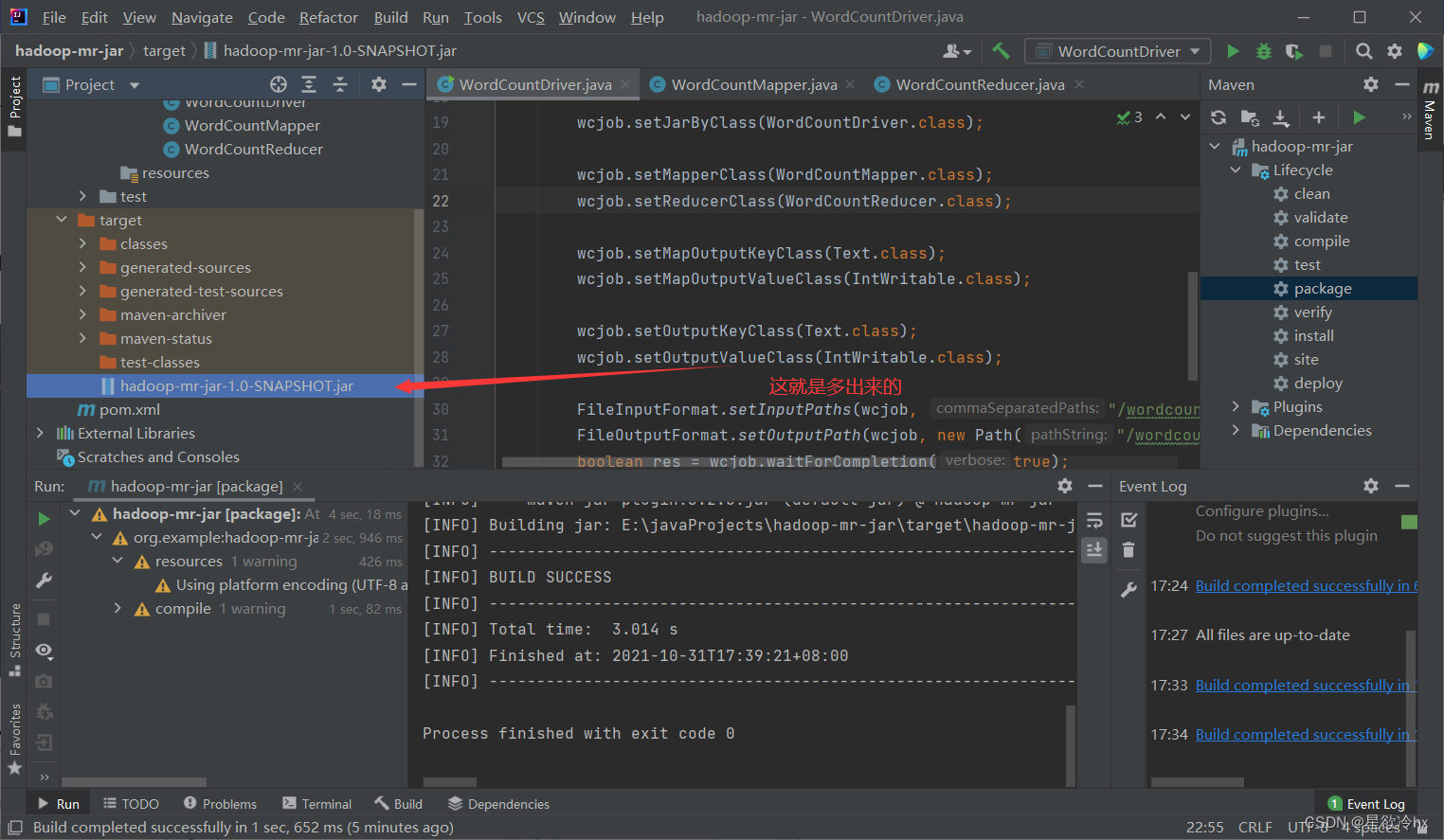
这张图就是多出来打包出来的那个包,找到之后就可以上传虚拟机
把jar包上传到centos下
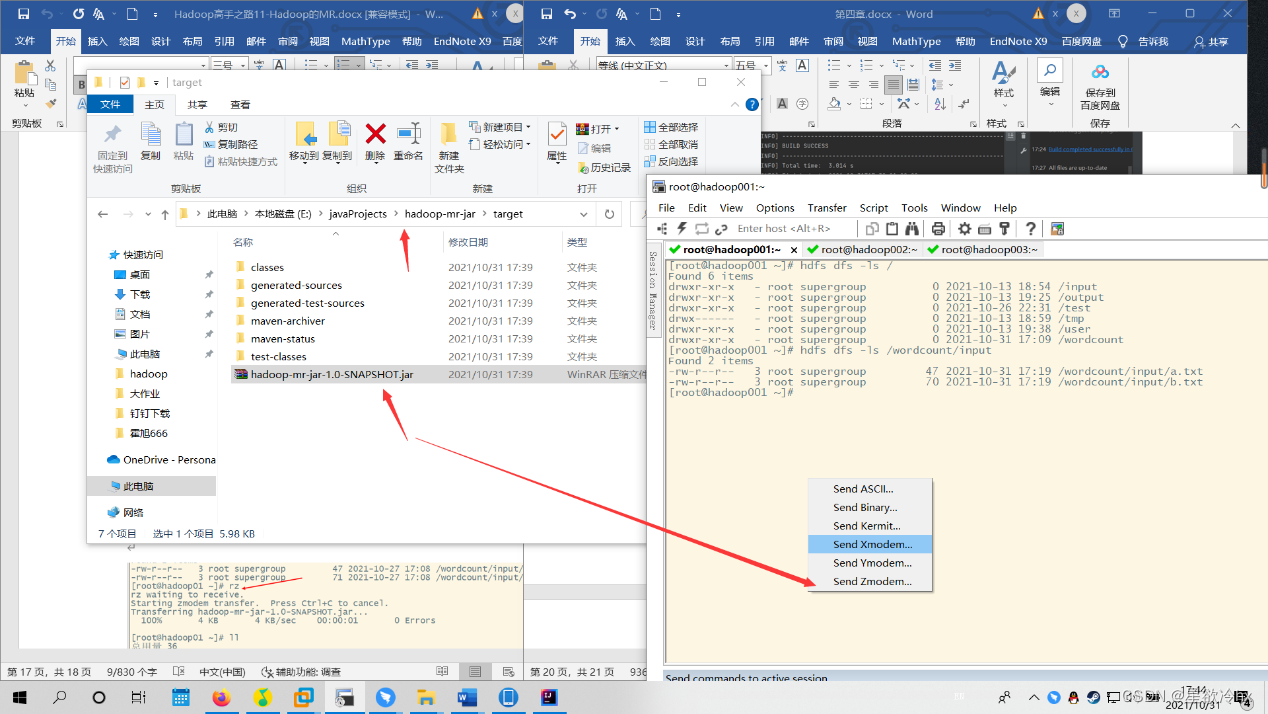
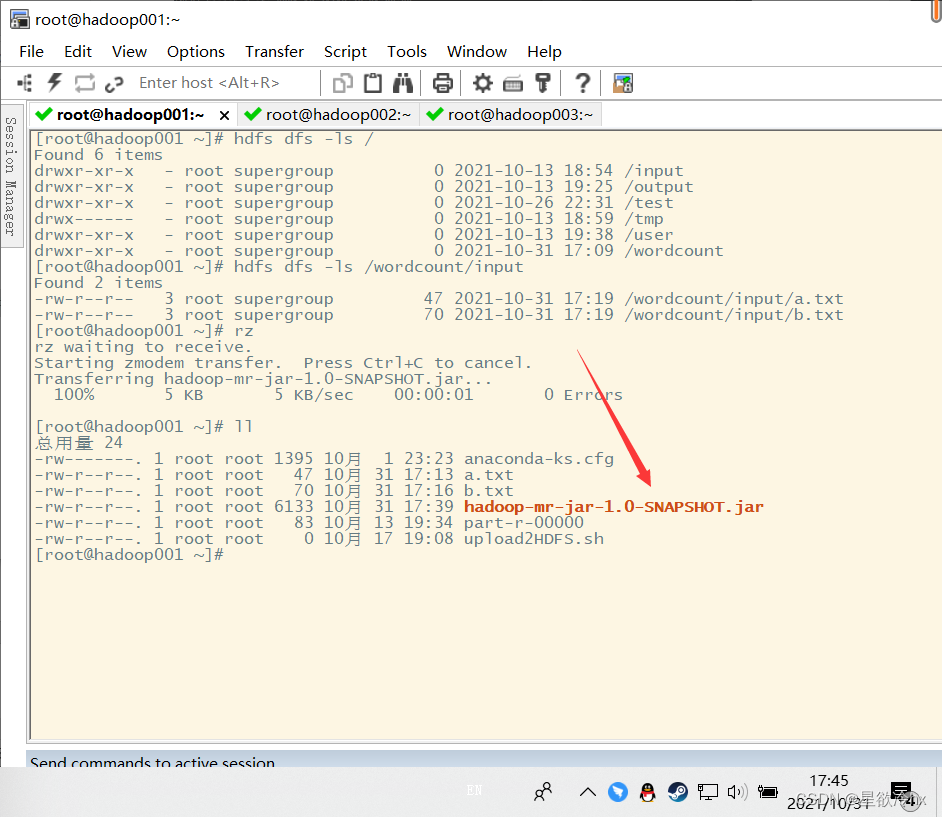
复制主类运行
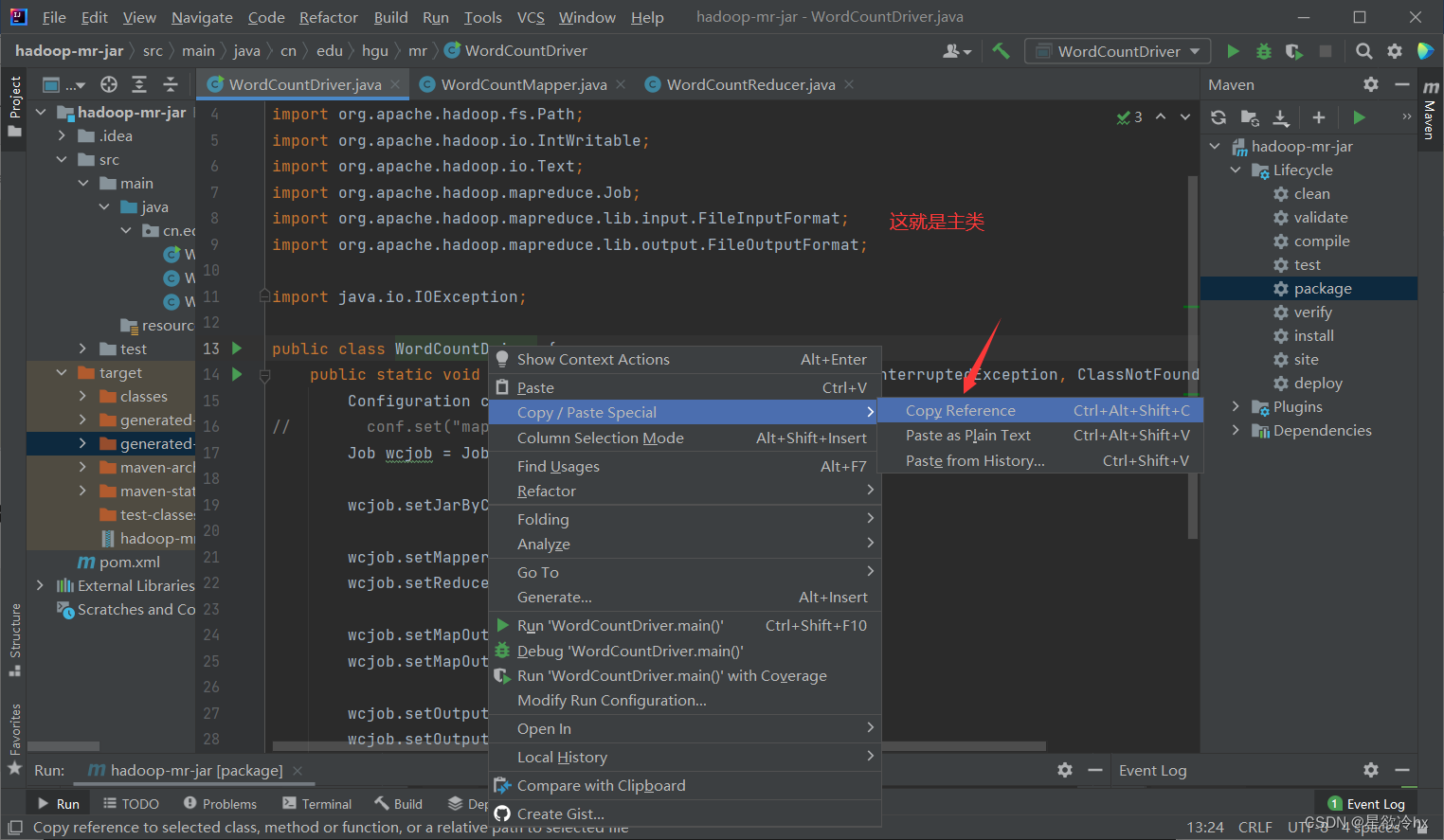

?查看运行过程和结果
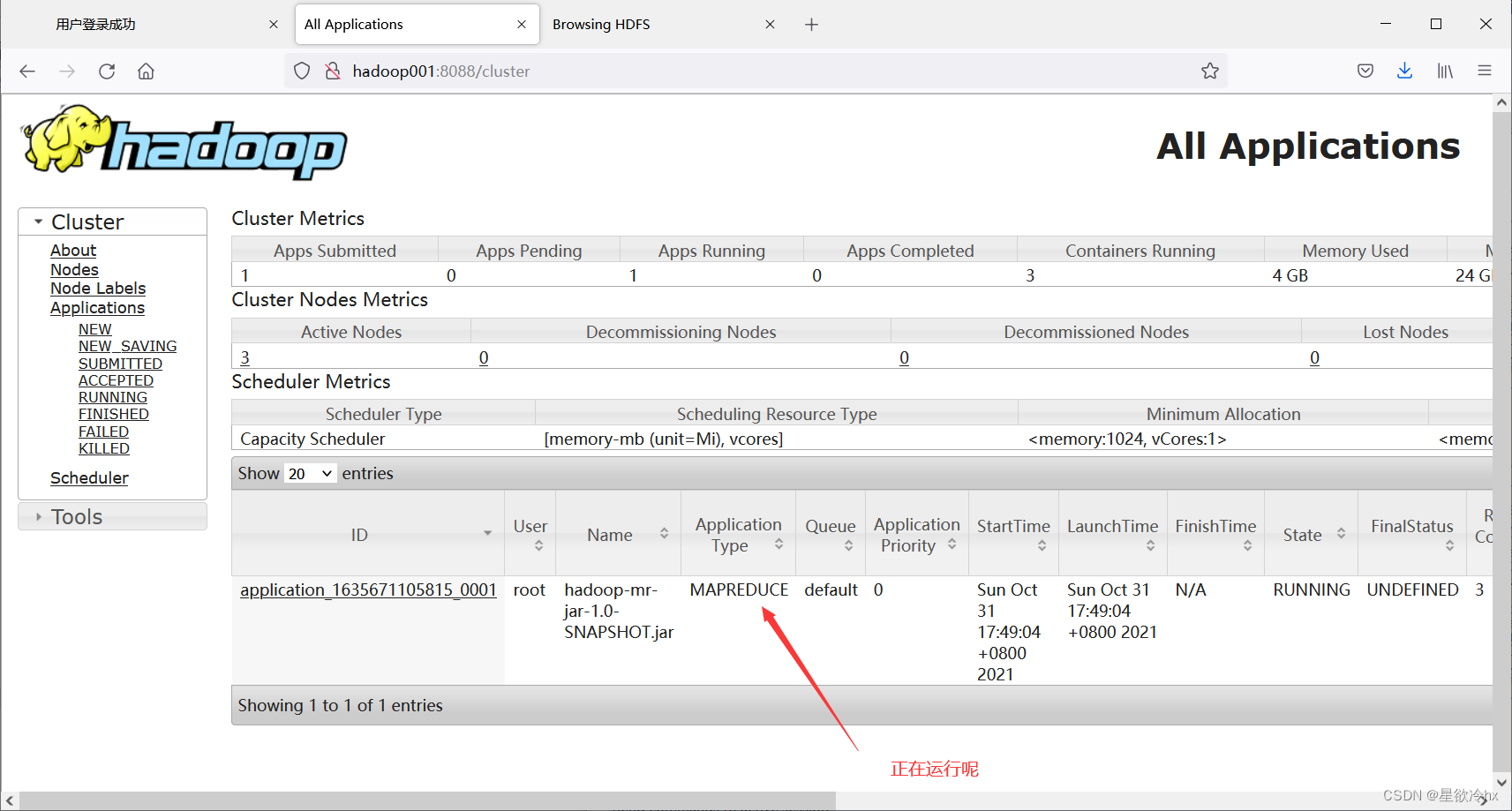
?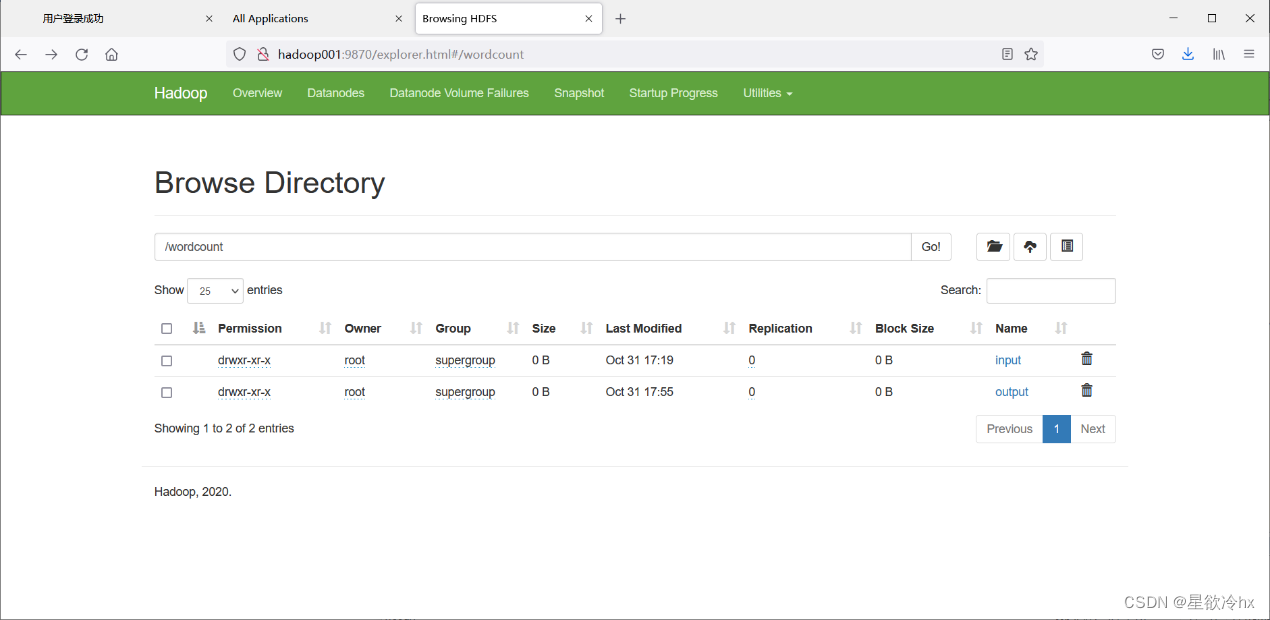
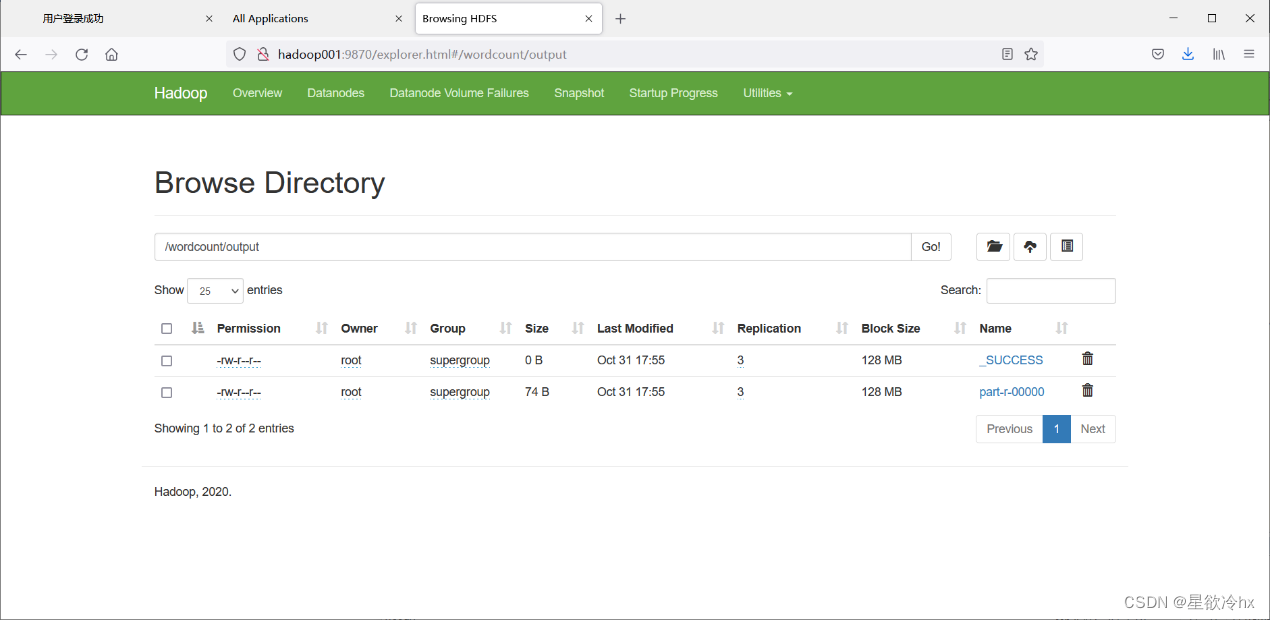
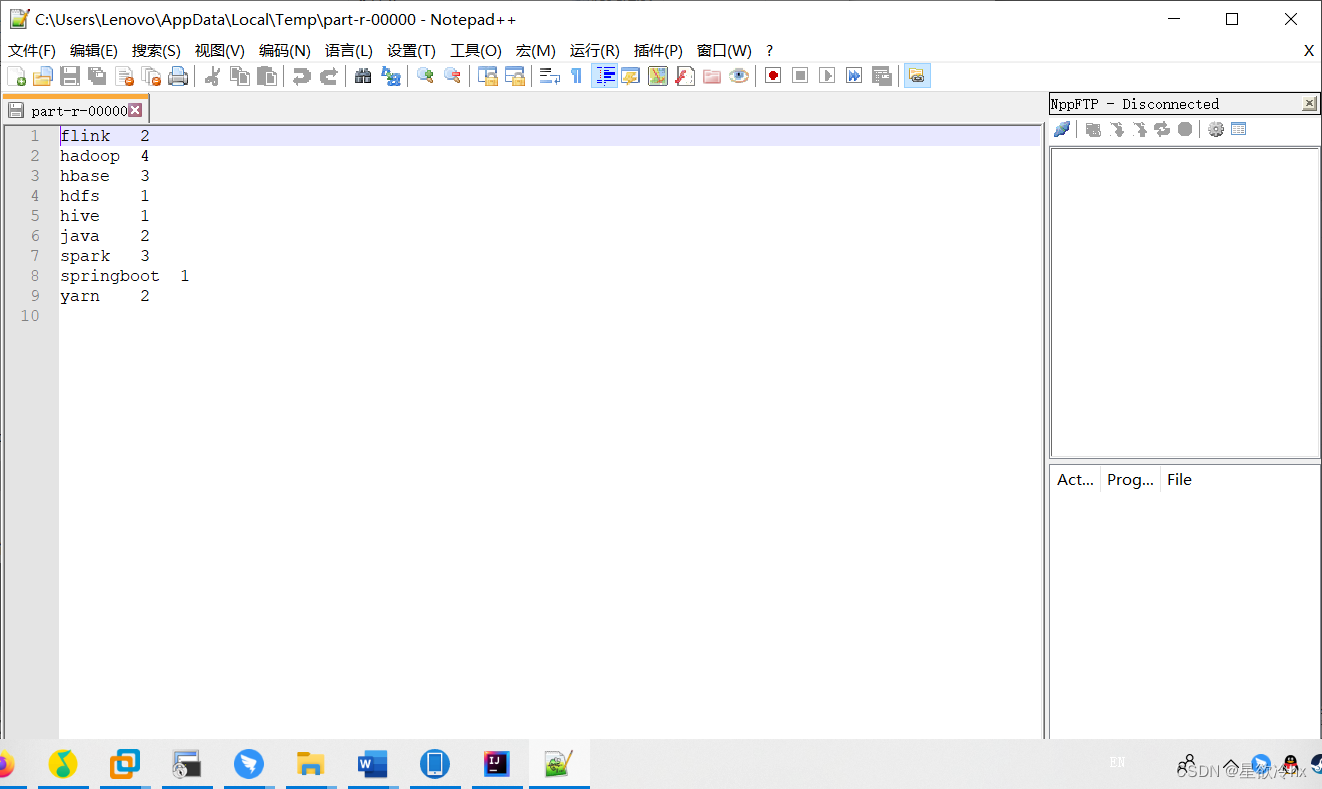
可以看到单词词频的统计结果已经成功。
三个教材上的案例
这是在上课的时候老师给留下的案例作业
就是参考的教材,教材是下面这版,大家有兴趣也可以去看一看
不过我看书看不太下去
书就是这本《Hadoop大数据技术原理与应用》,是2019年5月清华大学出版社出版的图书,作者是黑马程序员。
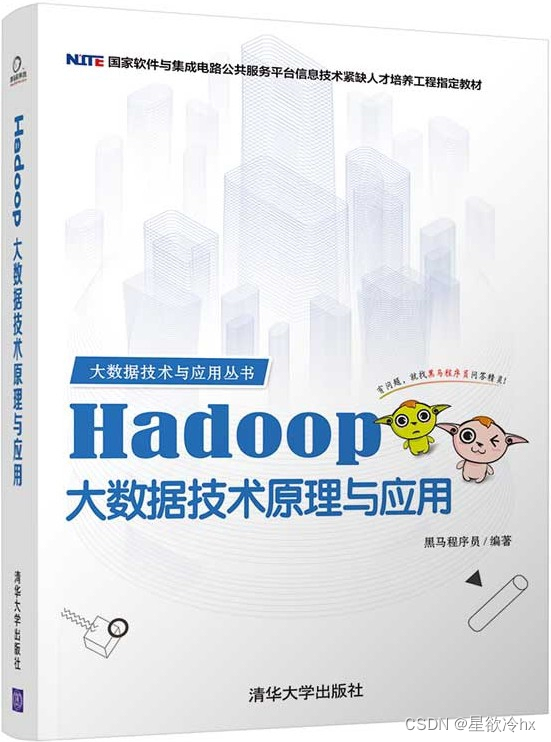
dedup 去重
Dedup是用来去重的
首先新建完maven之后要修改pom文件
为了方便可以直接复制之前的的mapreducer
也可以在一个项目里的不同包里写
首先先编辑pom文件
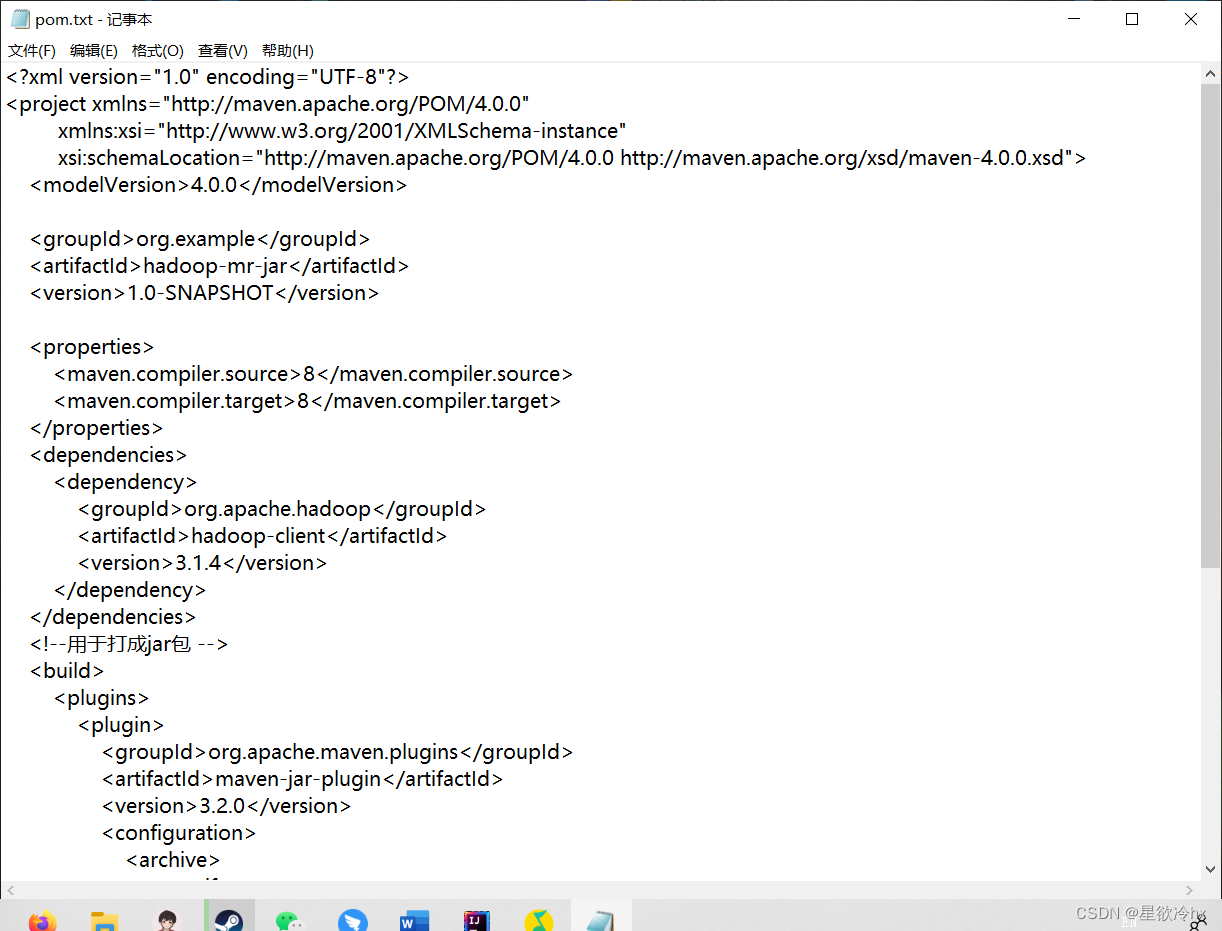
Driver
这里需要注意的的一点是代码不可能直接拿过去就用,除非你和我的环境一模一样
不然会就需要改地址和主机名之类的东西。
package dedup;
import java.io.IOException;
import org.apache.hadoop.conf.Configuration;
import org.apache.hadoop.fs.Path;
import org.apache.hadoop.io.NullWritable;
import org.apache.hadoop.io.Text;
import org.apache.hadoop.mapreduce.Job;
import org.apache.hadoop.mapreduce.lib.input.FileInputFormat;
import org.apache.hadoop.mapreduce.lib.output.FileOutputFormat;
public class DedupDriver {
public static void main(String[] args) throws IOException, ClassNotFoundException, InterruptedException{
Configuration conf = new Configuration();
Job job =Job.getInstance();
job.setJarByClass(DedupDriver.class);
job.setMapperClass(DedupMapper.class);
job.setReducerClass(DedupReducer.class);
job.setOutputKeyClass(Text.class);
job.setOutputValueClass(NullWritable.class);
FileInputFormat.setInputPaths(job, new Path("E://Dedup//input"));
//指定处理完成之后的结果所保存的位置
FileOutputFormat.setOutputPath(job, new Path("E://Dedup//output"));
job.waitForCompletion(true);
}
}
mapper
package dedup;
import java.io.IOException;
import org.apache.hadoop.io.LongWritable;
import org.apache.hadoop.io.NullWritable;
import org.apache.hadoop.io.Text;
import org.apache.hadoop.mapreduce.Mapper;
public class DedupMapper extends Mapper<LongWritable, Text, Text, NullWritable> {
private static Text field = new Text();
//<0,2020-9-3 c><11,2020-9-4 d>@Override
protected void map(LongWritable key, Text value, Context context) throws IOException, InterruptedException{
field = value;
//NullWritable.get()方法设置空值
context.write(field, NullWritable.get());
}
}
reducer
package dedup;
import java.io.IOException;
import org.apache.hadoop.io.NullWritable;
import org.apache.hadoop.io.Text;
import org.apache.hadoop.mapreduce.Reducer;
public class DedupReducer extends Reducer<Text, NullWritable, Text, NullWritable> {
//<2020-9-3 c.null> <2020-9-4 d.null><2020-9-4 d.null>
@Override
protected void reduce(Text key, Iterable<NullWritable> values, Context context)
throws IOException, InterruptedException {
context.write(key, NullWritable.get());
}
}
编写需要输入的文件
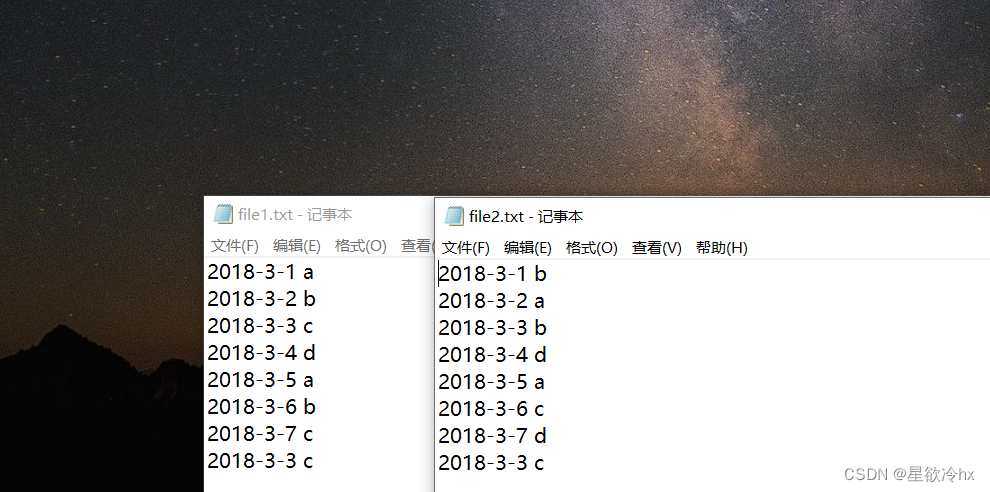
?
成功运行
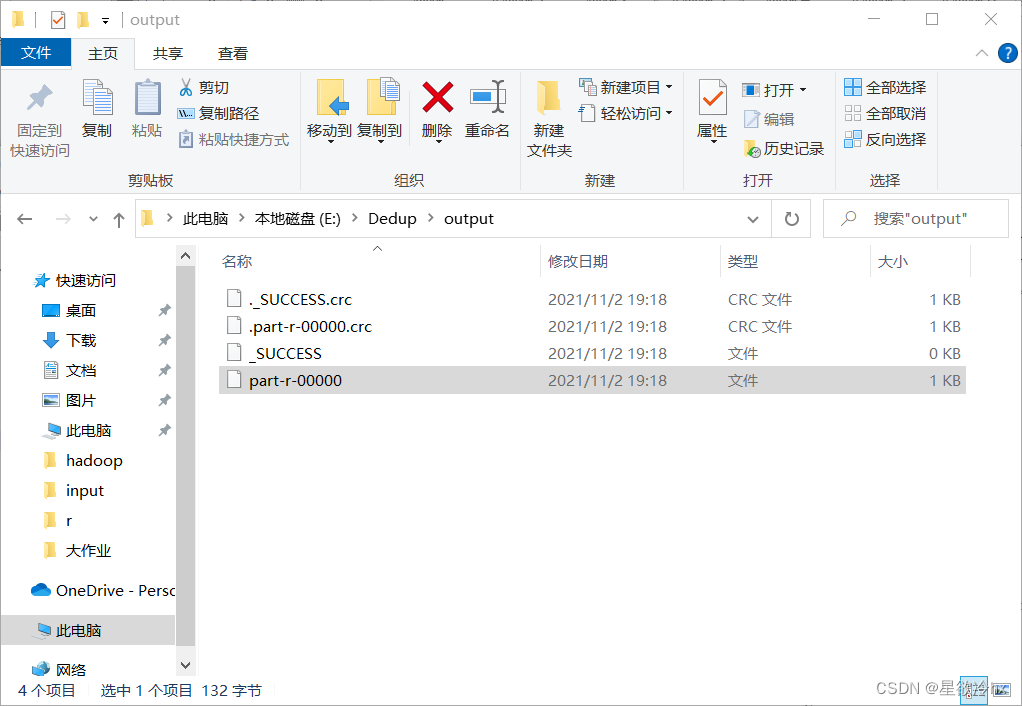
?
?invertedIndex倒序索引
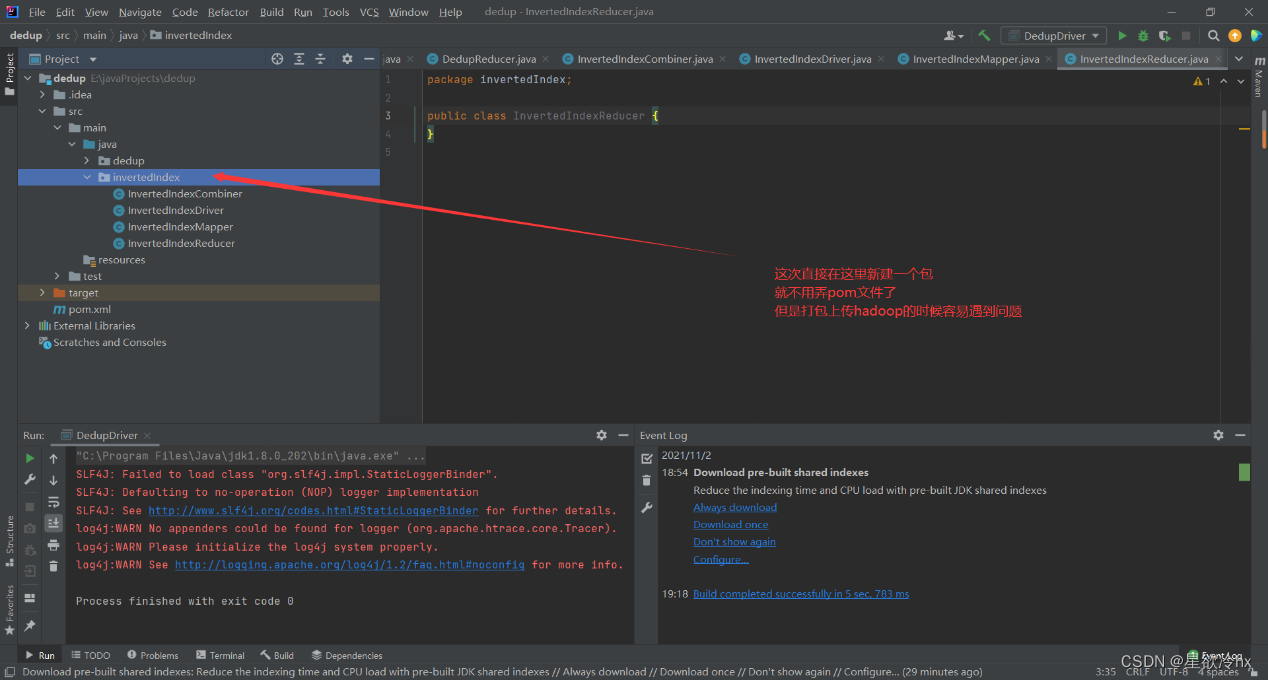
?Combiner
package invertedIndex;
import java.io.IOException;
import org.apache.hadoop.io.Text;
import org.apache.hadoop.mapreduce.Reducer;
public class InvertedIndexCombiner extends Reducer<Text, Text, Text, Text>{
private static Text info = new Text();
//输入: <MapReduce:file3 {1,1..>
//输出: <MapReduce file3:2>
@Override
protected void reduce(Text key, Iterable<Text> values, Context context) throws IOException,InterruptedException{
int sum=0;//统计词频
for (Text value : values) {
sum += Integer.parseInt(value.toString());
}
int splitIndex = key.toString().indexOf(":");//重新设置value值由URL和词频组成
info.set(key.toString().substring(splitIndex +1) +":" + sum);
//重新设置key值为单词
key.set(key.toString().substring(0, splitIndex));
context.write(key, info);
}
}
Driver
package invertedIndex;
import java.io.IOException;
import org.apache.hadoop.conf.Configuration;
import org.apache.hadoop.fs.Path;
import org.apache.hadoop.io.Text;
import org.apache.hadoop.mapreduce.Job;
import org.apache.hadoop.mapreduce.lib.input. FileInputFormat;
import org.apache.hadoop.mapreduce.lib.output.FileOutputFormat;
public class InvertedIndexDriver {
public static void main(String[] args) throws ClassNotFoundException,IOException,InterruptedException{
Configuration conf = new Configuration();
Job job = Job.getInstance();
job.setJarByClass(InvertedIndexDriver.class);
job.setMapperClass(InvertedIndexMapper.class);
job.setCombinerClass(InvertedIndexCombiner.class);
job.setReducerClass(InvertedIndexReducer.class);
job.setOutputKeyClass(Text.class);
job.setOutputValueClass(Text.class);
FileInputFormat.setInputPaths(job, new Path("E:\\InvertedIndex\\input"));
//指定处理完成之后的结果所保存的位置
FileOutputFormat.setOutputPath(job, new Path("E:\\InvertedIndex\\output"));
//向yarn集群提交这个job
boolean res =job.waitForCompletion(true);
System.exit(res? 0: 1);
}
}
reducer
package invertedIndex;
import java.io.IOException;
import org.apache.hadoop.io.Text;
import org.apache.hadoop.mapreduce.Reducer;
public class InvertedIndexReducer extends Reducer<Text, Text, Text, Text> {
private static Text result = new Text();
//输入: <MapReduce file3:2>
//输出: <MapReduce file1:1;file2:1;file3:2;>
@Override
protected void reduce(Text key, Iterable<Text> values, Context context)
throws IOException, InterruptedException {
//生成文档列表
String fileList = new String();
for (Text value : values) {
fileList += value.toString() +";";
}
result.set(fileList);
context.write(key, result);
}
}
创建所需的输入文件
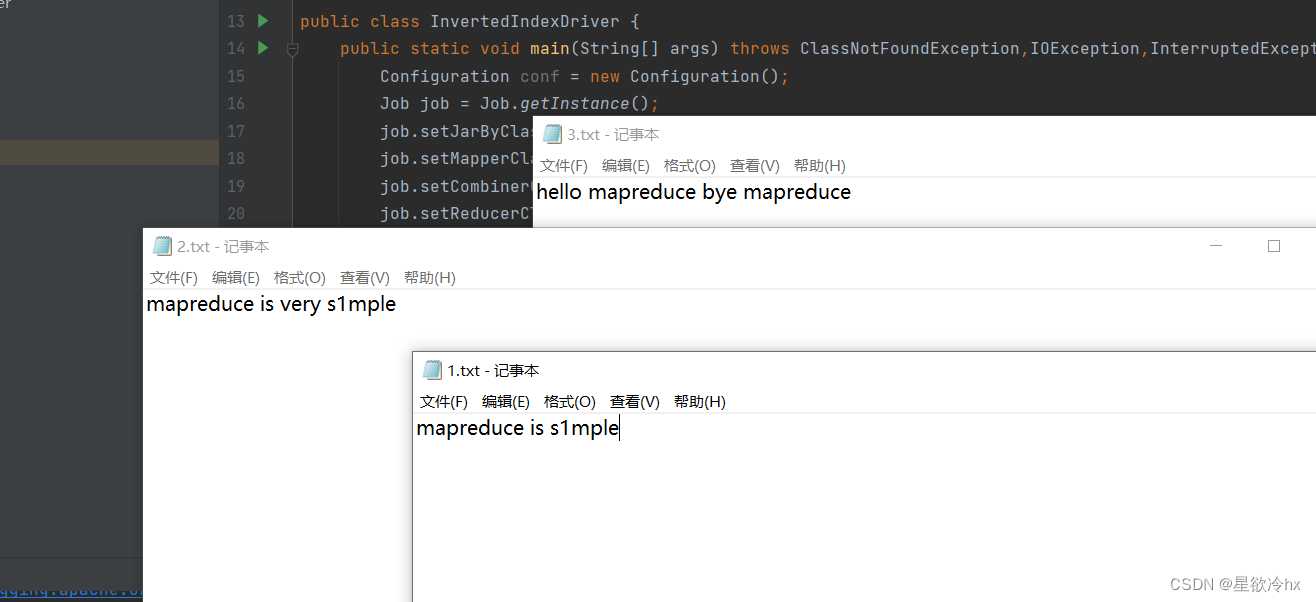
?运行成功
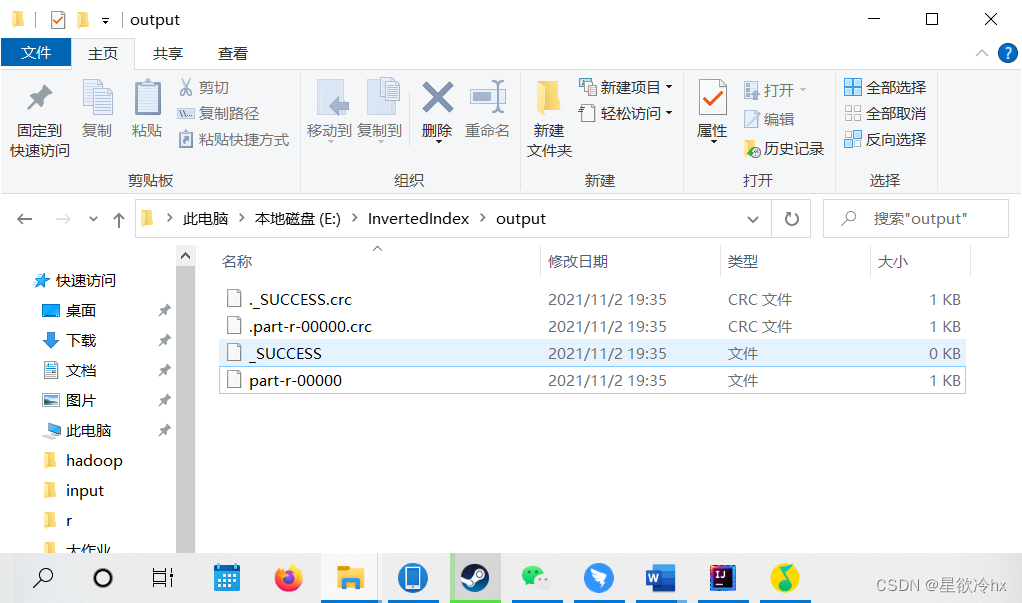
?
?topN
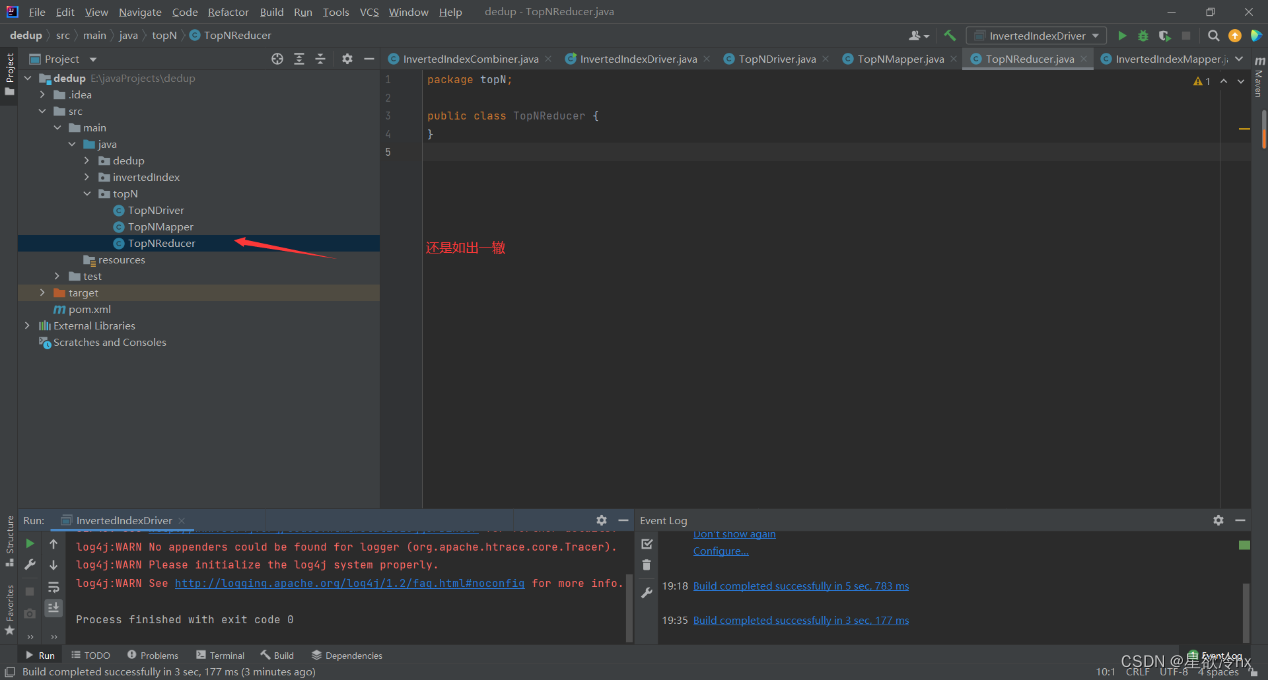
?Driver
package topN;
import org.apache.hadoop.fs.Path;
import org.apache.hadoop.io.IntWritable;
import org.apache.hadoop.io.NullWritable;
import org.apache.hadoop.mapreduce.Job;
import org.apache.hadoop.mapreduce.lib.input.FileInputFormat;
import org.apache.hadoop.mapreduce.lib.output.FileOutputFormat;
public class TopNDriver {
public static void main(String[] args) throws Exception {
Job job = Job.getInstance();
job.setJarByClass(TopNDriver.class);
job.setMapperClass(TopNMapper.class);
job.setReducerClass(TopNReducer.class);
job.setMapOutputKeyClass(NullWritable.class);
job.setMapOutputValueClass(IntWritable.class);
job.setOutputKeyClass(NullWritable.class);
job.setOutputValueClass(IntWritable.class);
FileInputFormat.addInputPath(job, new Path("E:\\topN\\input"));
FileOutputFormat.setOutputPath(job, new Path("E:\\topN\\output"));
boolean flag = job.waitForCompletion(true);
System.exit(flag ? 0 : 1);
}
}
mapper
package topN;
import java.util.TreeMap;
import org.apache.hadoop.io.IntWritable;
import org.apache.hadoop.io.LongWritable;
import org.apache.hadoop.io.NullWritable;
import org.apache.hadoop.io.Text;
import org.apache.hadoop.mapreduce.Mapper;
import java.io.IOException;
public class TopNMapper extends Mapper<LongWritable, Text, NullWritable, IntWritable>{
//TreeMap默认对key升序排序
private TreeMap<Integer, String> treemap = new TreeMap<Integer, String>();
private IntWritable iw = new IntWritable();
@Override
protected void map(LongWritable key, Text value,
Mapper<LongWritable, Text, NullWritable, IntWritable>.Context context)
throws IOException, InterruptedException {
String val = value.toString();
String[] vals = val.split(" ");
for(String v : vals) {
treemap.put(Integer.parseInt(v), v);
if(treemap.size()>5) {
//如果treemap长度大于5,就把第一个key删掉
treemap.remove(treemap.firstKey());
}
}
}
@Override
protected void cleanup(Mapper<LongWritable, Text, NullWritable, IntWritable>.Context context)
throws IOException, InterruptedException {
for(Integer i : treemap.keySet()) {
iw.set(i);
context.write(NullWritable.get(), iw);
}
}
}
reducer
package topN;
import java.io.IOException;
import java.util.Comparator;
import java.util.TreeMap;
import org.apache.hadoop.io.IntWritable;
import org.apache.hadoop.io.NullWritable;
import org.apache.hadoop.mapreduce.Reducer;
public class TopNReducer extends Reducer<NullWritable, IntWritable, NullWritable, IntWritable>{
private IntWritable iw = new IntWritable();
//倒序输出
private TreeMap<Integer, String> treemap = new TreeMap<Integer, String>(new Comparator<Integer>() {
public int compare(Integer o1, Integer o2) {
return o2-o1;
}
});
@Override
protected void reduce(NullWritable key, Iterable<IntWritable> value,
Reducer<NullWritable, IntWritable, NullWritable, IntWritable>.Context context)
throws IOException, InterruptedException {
for(IntWritable iw : value) {
treemap.put(iw.get(), NullWritable.get()+" ");
if(treemap.size()>5) {
treemap.remove(treemap.lastKey());
}
}
}
@Override
protected void cleanup(Reducer<NullWritable, IntWritable, NullWritable, IntWritable>.Context context)
throws IOException, InterruptedException {
for(Integer i : treemap.keySet()) {
iw.set(i);
context.write(NullWritable.get(), iw);
}
}
}
设置所需的文件
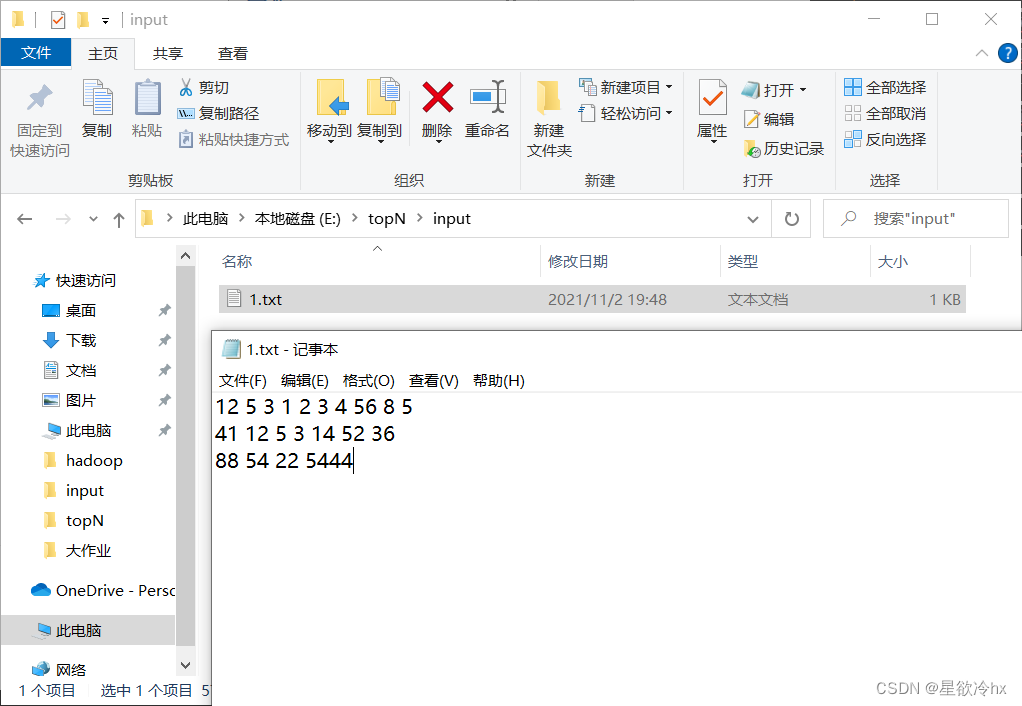
?运行成功
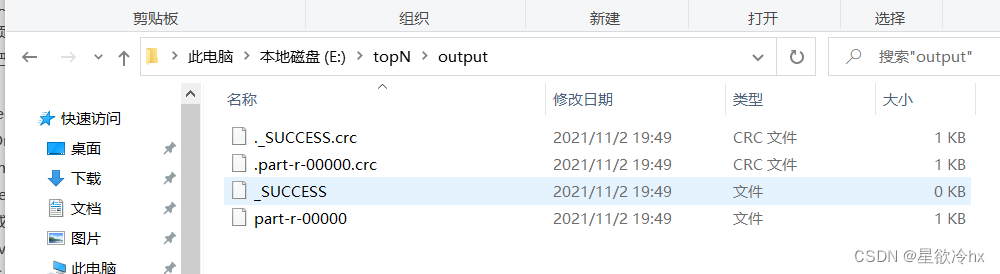
?
到这里mapreduce基本上就介绍完毕了,大家可以多研读这几个代码,理解起来其实也相对简单。
下一节内容应该会是zookeeper,也希望大家一起进步。
如遇侵权,请联系删除。
|