????????非关系型数据库redis以及levedb,rockdb其核心存储引擎的数据结构就是跳表。本项目是基于跳表实现的轻量级键值型存储引擎,使用C++实现。插入数据、删除数据、查询数据、数据展示、数据落盘、文件加载数据,以及数据库大小显示。
一、跳表定义
????????链表的数据结构为一个节点中除了存储数据,还存储了下一个节点的地址。因此如果我们想要在其中查找某个数据,也只能从头到尾遍历,查询效率低,时间复杂度是O(n)。如下图所示。

? ? ? ? ?为了提高查找效率,在原始链表上建立索引节点,每个索引节点保存下一个索引节点与下一层相同数据节点的地址,如下图所示。

????????假如我们现在查找某个节点,比如23,先遍历索引层,当遍历到19的时候,发现下一个节点是25,那么23肯定在19到25这两个节点之间。使用down指针,下降到下一级原始链表层,继续遍历,这时候我们只需要遍历2个节点就找到23了,相比于从原始链表开始遍历,要快3个节点。
????????从上面可以看出,加上一层索引之后,查询效率提高了很多,那么再加上一层呢?我们在第一级索引上再加上第二级索引,建立方式和之前一样,每两个节点抽象出一个节点到第二级索引。现在查找23节点,只需要遍历6个节点就能找到,遍历的次数又减少了。
????????假如节点变得越来越多,一个有64个节点,建立5个索引层,那么查询第62个节点,在没有建立索引表之前,需要遍历62个节点,建立索引表之后,只需要遍历11个节点,速度提高了很多。所以当链表的长度n比较大的时候,比如1000000,在构建索引之后,查询效率的提升会非常的明显。
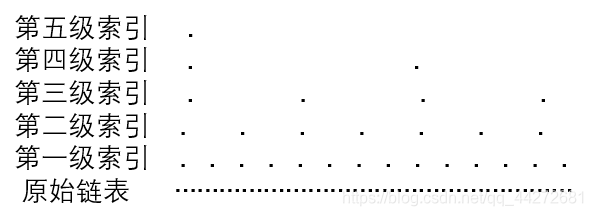
二、代码分析
1.节点与链表类
template<typename K, typename V>
class Node
{
public:
Node(){}
Node(K k, V v, int);
~Node();
K get_key() const;
V get_value() const;
void set_value(V);
Node<K, V> **forward;
int node_level;
private:
K key;
V value;
};
template<typename K, typename V>
class SkipList
{
public:
SkipList(int);
~SkipList();
int get_random_level();
Node<K, V>* create_node(K, V, int);
int insert_element(K, V);
void display();
bool search_element(K);
void delete_element(K);
void upload_data();
void download_data();
int size();
private:
void get_data_from_string(const std::string& str, std::string* key, std::string* value);
bool is_valid_string(const std::string& str);
int max_level;
int skiplist_level;
Node<K, V>* _header;
std::ifstream file_read;
std::ofstream file_write;
int element_count;
};
程序的数据结构提供了插入数据、删除数据、查询数据、数据展示、存储数据、加载文件数据以及数据结构大小显示的接口。其中max_level为程序限制的最大层数,skiplist_level为数据结构的当前层数。
2.插入数据
template<typename K, typename V>
int SkipList<K, V>::insert_element(const K key, const V value)
{
mtx.lock();
Node<K, V>* current = this->_header;
Node<K, V>* update[max_level + 1];
memset(update, 0, sizeof(Node<K, V>*)*(max_level + 1));
for(int i = skiplist_level; i >= 0; --i)
{
while(current->forward[i] != NULL && current->forward[i]->get_key() < key)
current = current->forward[i];
update[i] = current;
}
current = current->forward[0];
if(current != NULL && current->get_key() == key)
{
std::cout << "key: " << key <<", exist" <<std::endl;
mtx.unlock();
return 1;
}
else
{
int random_level = get_random_level();
if(random_level > skiplist_level)
{
for(int i = skiplist_level + 1; i <= random_level; ++i)
update[i] = _header;
skiplist_level = random_level;
}
Node<K, V>* insert_node = create_node(key, value, random_level);
for(int i = 0; i < random_level; ++i)
{
insert_node->forward[i] = update[i]->forward[i];
update[i]->forward[i] = insert_node;
}
std::cout << "insert success. key: " << key << ", value: " << value << std::endl;
element_count++;
}
mtx.unlock();
return 0;
}
首先定义一个节点数组update,用来保存从头节点_header开始,每层第一个大于等于节点大小的节点地址。接着从当前的最高层开始,保存该节点数据到update。那么到了原始链表后,如果要插入的数据在原始链表中不存在,current->forward[0]与current必然为分别大于小于插入数据的原始链表数据。接着用get_random_level()函数取一个不大于最大层数的随机数,用来作为该插入值的层数。最后从原始链表开始,逐层往上递增,插入数据。
3.删除与查询数据
template<typename K, typename V>
void SkipList<K, V>::delete_element(K key)
{
mtx.lock();
Node<K, V>* current = this->_header;
Node<K, V>* update[max_level + 1];
memset(update, 0, sizeof(Node<K, V>*)*(max_level + 1));
for(int i = skiplist_level; i >= 0; --i)
{
while(current->forward[i] != NULL && current->forward[i]->get_key() < key)
current = current->forward[i];
update[i] = current;
}
current = current->forward[0];
if(current != NULL && current->get_key() == key)
{
for(int i = 0; i <=skiplist_level; ++i)
{
if(update[i]->forward[i] != current)
break;
update[i]->forward[i] = current->forward[i];
}
while(skiplist_level > 0 && _header->forward[skiplist_level] == 0)
skiplist_level--;
std::cout << "delete key " << key << std::endl;
element_count--;
}
mtx.unlock();
return;
}
template<typename K, typename V>
bool SkipList<K, V>::search_element(K key)
{
std::cout << "search element--------" << std::endl;
Node<K, V>* current = _header;
for(int i = max_level; i >= 0; --i)
{
while(current->forward[i] && current->forward[i]->get_key() < key)
current = current->forward[i];
}
current = current->forward[0];
if(current && current->get_key() == key)
{
std::cout << "Found key: " << key << " , value: " << current->get_value() << std::endl;
return true;
}
else
{
std::cout << "Not Found key: " << key << std::endl;
return false;
}
}
这两个函数的功能类似,先查询某个值在链表中是否存在,若存在,查询函数打印,删除函数删除。
4.上传与下载数据
template<typename K, typename V>
void SkipList<K, V>::upload_data()
{
std::cout << "dump file------" << std::endl;
file_write.open(STORE_FILE);
Node<K, V>* node = this->_header->forward[0];
while(node != NULL)
{
file_write << node->get_key() << " : " << node->get_value() << "\n";
std::cout << node->get_key() << " : " << node->get_value() << ";\n";
node = node->forward[0];
}
file_write.flush();
file_write.close();
}
template<typename K, typename V>
void SkipList<K, V>::download_data()
{
file_read.open(STORE_FILE);
std::cout << "download data--------" << std::endl;
std::string line;
std::string* key = new std::string();
std::string* value = new std::string();
while(getline(file_read, line))
{
get_data_from_string(line, key, value);
if(key->empty() || value->empty())
continue;
insert_element(*key, *value);
std::cout << "key: " << *key << " value: " << *value << std::endl;
}
file_read.close();
}
即把数据保存到单独的文件中。
三、数据结构复杂度分析
1.空间复杂度
假设原始链表的长度是 N,第一级索引大约是 N/2,第二级索引大约是 N/4,以此类推,每一层减少一半,直至剩下两个点,其实就是一个等比数列,计算可以得到: ,所以跳表的空间复杂度是 O(n),也就是说如果将n个节点的单链表构成跳表,需要额外将近n个节点的空间。如果我们使用3个节点或者5个节点:

?求和得到的节点,大约是? ,尽管空间复杂度还是O(n),但是存储空间减少了一半。
注意,当原始链表中存储的数据很多的时候,索引节点只需要存储关键值和几个指针,并不需要存储对象,所以当对象比索引节点大很多的时候,索引节点的存储空间就可以忽略不计了。
2.时间复杂度
按照每两个节点抽出一个节点作为上一级索引的节点,那么第一级索引节点大约是 n/2 个,第二级的索引大约是 n/4 个,以此类推,第 k 级索引的节点个数是第 k-1 级索引的节点个数的 1/2,那么第 k 级索引点的个数: 。
假设索引有 h 级,最高级的索引是 2 个节点,通过上面的例子可以得到:

求得:
如果包含原始链表这一层,那么整个跳表的高度是: 。?
在跳表查询某个数据的时候,如果每一层都要遍历m个节点,那在跳表查询一个数据的时间复杂度是: 。在每两个节点建立一个索引的极限情况下,每层最多遍历三个节点,因此m的最大值为3。所以在跳表中查找任意数据的时间复杂度是 O(logn),查找的时间复杂度和二分查找是一样的。这种查找效率的提升,前提是建立了很多级索引。
四、总结与源码
跳表使用的是空间换时间的思想,通过构建多级索引来提高查询效率,实现基于链表的“二分查找”,是一种动态的数据结构,支持快速的查找、插入和删除操作,时间复杂度是 O(logn),空间复杂度是 O(n),不过可以通过改变索引策略,动态平衡执行效率和内存消耗。
?源码:nanjingu/skiplist (github.com)
|