用sklearn实现各种线性回归模型,训练数据为房价预测数据,数据文件见https://download.csdn.net/download/d1240673769/20910882
加载房价预测数据
import pandas as pd
df = pd.read_csv('sample_data_sets.csv')
print(df.columns)
df.head()
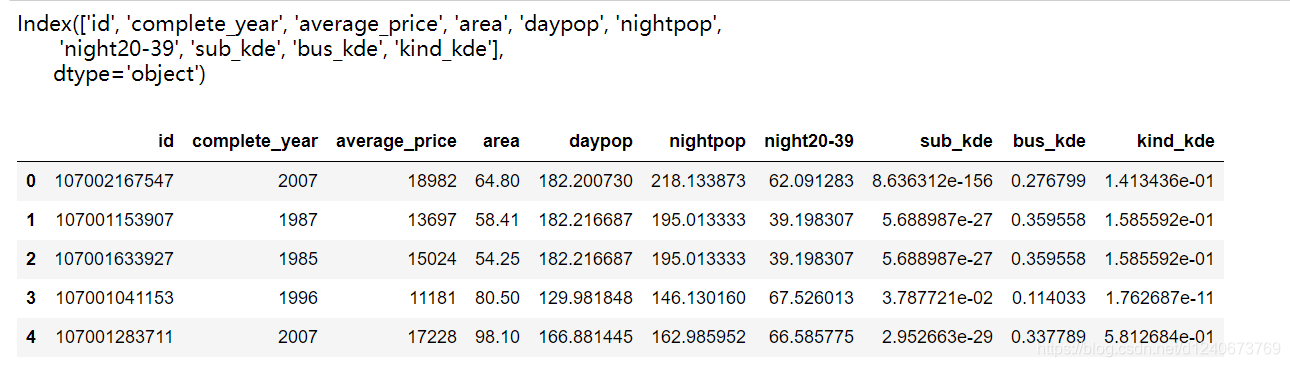
制作标签变量
price_median = df['average_price'].median()
print(price_median)
df['is_high'] = df['average_price'].map(lambda x: True if x>= price_median else False)
print(df['is_high'].value_counts())
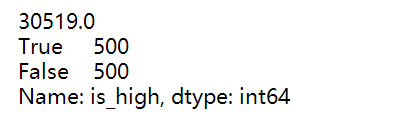
提取自变量和因变量
x_train = df.copy()[['area', 'daypop', 'nightpop',
'night20-39', 'sub_kde', 'bus_kde', 'kind_kde']]
y_train = df.copy()['average_price']
y_label = df.copy()['is_high']
线性回归模型
from sklearn.pipeline import Pipeline
from sklearn.linear_model import LinearRegression
pipe_lm = Pipeline([
('lm_regr',LinearRegression(fit_intercept=True))
])
pipe_lm.fit(x_train, y_train)
y_train_predict = pipe_lm.predict(x_train)
print(pipe_lm.named_steps['lm_regr'].coef_)
print(pipe_lm.named_steps['lm_regr'].intercept_)

coef = pipe_lm.named_steps['lm_regr'].coef_
features = x_train.columns.tolist()
coef_table = pd.DataFrame({'feature': features, 'coefficient': coef})
print(coef_table)
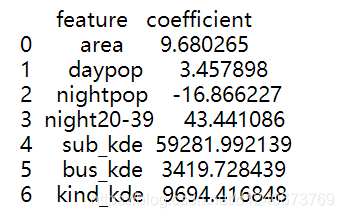
import matplotlib.pyplot as plt
coef_table.set_index(['feature']).plot.barh()
plt.axvline(0, color='k')
plt.show()
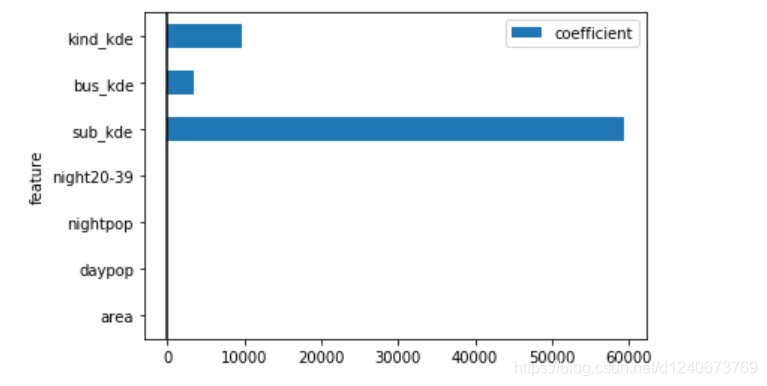
coef_table.set_index(['feature']).iloc[0:4].plot.barh()
plt.axvline(0, color='k')
plt.show()
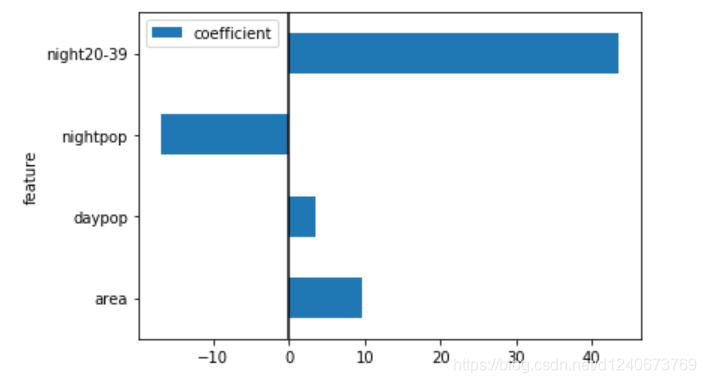
lasso线性回归模型
from sklearn.linear_model import Lasso
pipe_lasso = Pipeline([
('lasso_regr',Lasso(alpha=500, fit_intercept=True))
])
pipe_lasso.fit(x_train, y_train)
y_train_predict = pipe_lasso.predict(x_train)
print(pipe_lasso.named_steps['lasso_regr'].coef_)
print(pipe_lasso.named_steps['lasso_regr'].intercept_)
 如上图:后三个特征值的参数被约束到0
coef = pipe_lasso.named_steps['lasso_regr'].coef_
features = x_train.columns.tolist()
coef_table = pd.DataFrame({'feature': features, 'coefficient': coef})
print(coef_table)
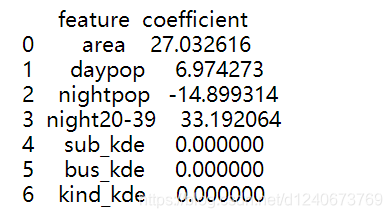
coef_table.set_index(['feature']).plot.barh()
plt.axvline(0, color='k')
plt.show()
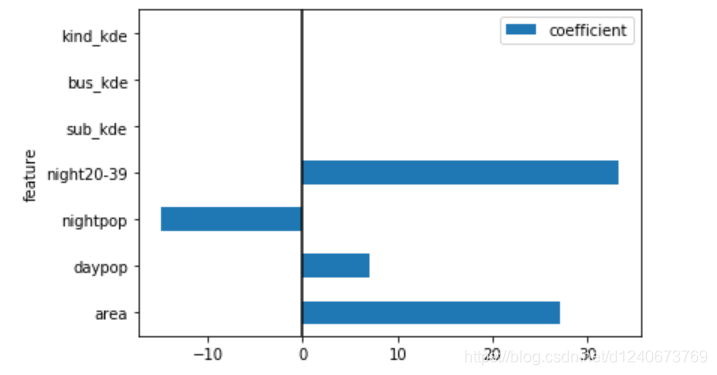
ridge线性回归模型
from sklearn.linear_model import Ridge
pipe_ridge = Pipeline([
('ridge_regr',Ridge(alpha=500, fit_intercept=True, solver = 'lsqr'))
])
pipe_ridge.fit(x_train, y_train)
y_train_predict = pipe_ridge.predict(x_train)
print(pipe_ridge.named_steps['ridge_regr'].coef_)
print(pipe_ridge.named_steps['ridge_regr'].intercept_)

coef = pipe_ridge.named_steps['ridge_regr'].coef_
features = x_train.columns.tolist()
coef_table = pd.DataFrame({'feature': features, 'coefficient': coef})
print(coef_table)
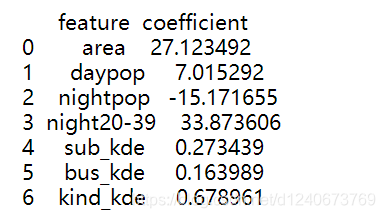
coef_table.set_index(['feature']).plot.barh()
plt.axvline(0, color='k')
plt.show()
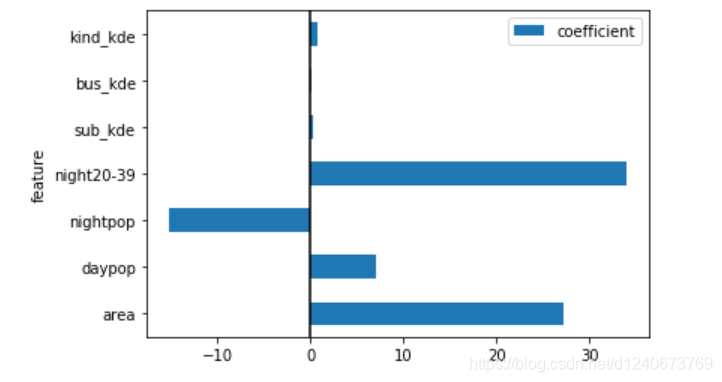
logstic回归模型
from sklearn.linear_model import LogisticRegression
pipe_logistic = Pipeline([
('logistic_clf',LogisticRegression(penalty='l1', fit_intercept=True, solver='liblinear'))
])
pipe_logistic.fit(x_train, y_label)
y_train_predict = pipe_logistic.predict(x_train)
逻辑回归模型参数解释:
penalty(默认使用l2正则系数)
- ‘l1’: l1正则系数,
- ‘l2’: l2正则系数
- ‘none’:无正则系数
solver(默认是’liblinear’:坐标下降法)
- ‘liblinear’:坐标下降法,可以处理了l1和l2正则系数,适用于小数据量(一般指10w个样本以下)
- ‘sag’:sag是随机平均梯度下降法,只能处理l2正则系数,适用于大数据量
- ‘saga’: saga是sag的变体,能处理l1和l2正则系数,适用于大数据量
print(pipe_logistic.named_steps['logistic_clf'].coef_)
print(pipe_logistic.named_steps['logistic_clf'].intercept_)

coef = pipe_logistic.named_steps['logistic_clf'].coef_[0]
features = x_train.columns.tolist()
coef_table = pd.DataFrame({'feature': features, 'coefficient': coef})
print(coef_table)
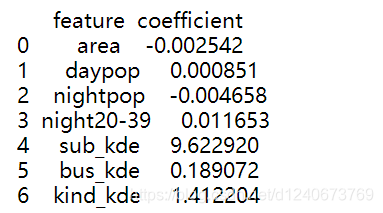
coef_table.set_index(['feature']).plot.barh()
plt.axvline(0, color='k')
plt.show()
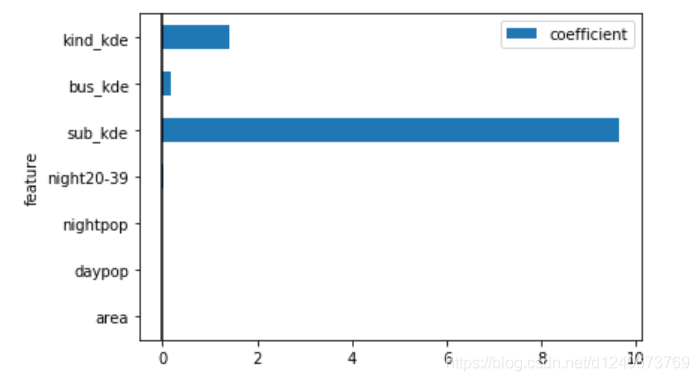
|