? 最近在学习吴恩达老师的机器学习课程,所以在这里记录一下,主要是完成他的课后作业。
思路: ?1.首先,我们自己编写线性回归函数,看看整个计算的流程; ?2.使用sklearn进行线性回归计算; ?3.对比以上两种方法的优缺点。
1.单变量线性回归:
import numpy as np
import pandas as pd
import matplotlib.pyplot as plt
path = r'C:\Users\Administrator\Desktop\data1.txt'
data = pd.read_csv(path, header=None, names=['Population', 'Profit'])
data.head()
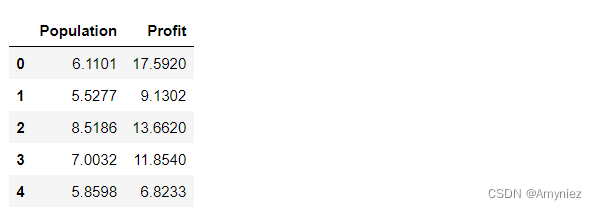
data.describe()
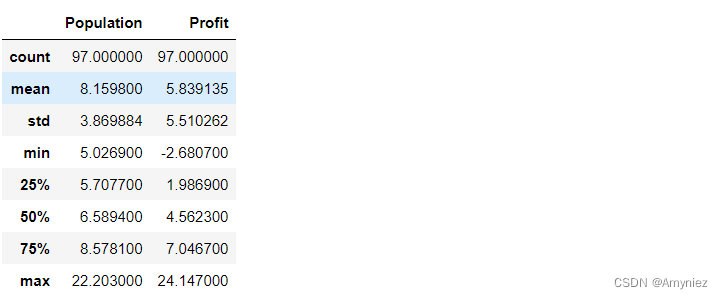
data.plot(kind='scatter', x='Population', y='Profit', figsize=(12,8))
plt.show()
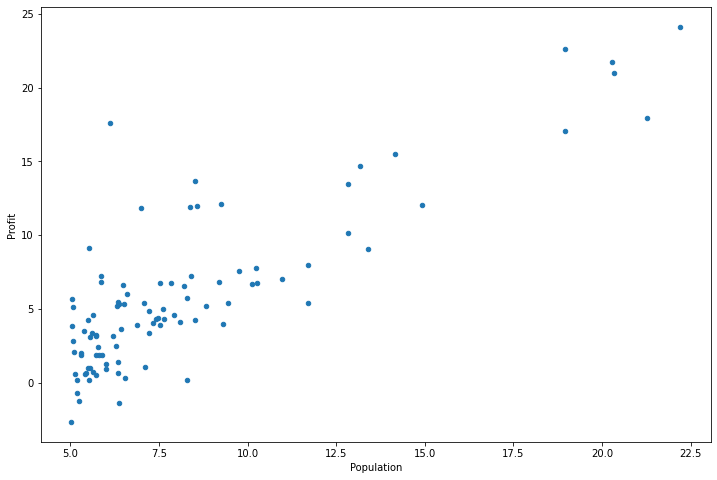
def computeCost(X, y, theta):
inner = np.power(((X * theta.T) - y), 2)
return np.sum(inner) / (2 * len(X)
data.insert(0, 'Ones', 1)
补充: 1、img.shape:(300, 534, 3) ? img.shape[0]:图像的垂直尺寸(高度);对于矩阵来说,表示矩阵的行数,即 300 ? img.shape[1]:图像的水平尺寸(宽度);对于矩阵来说,表示矩阵的列数,即 534 ? img.shape[2]:图像的通道数,即 3 2、iloc[ : , : ]函数的使用: ? 前面的冒号就是取行数,后面的冒号是取列数
cols = data.shape[1]
X = data.iloc[:,0:cols-1]
y = data.iloc[:,cols-1:cols]
X.head()
y.head()
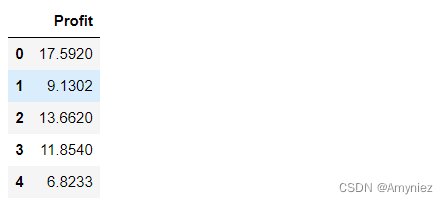 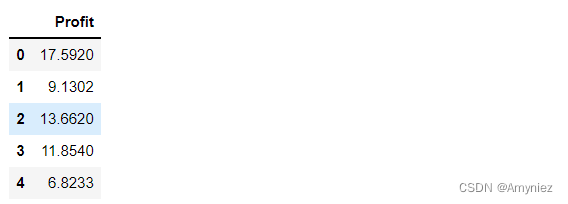
X = np.matrix(X.values)
y = np.matrix(y.values)
theta = np.matrix(np.array([0,0]))
theta
X.shape, y.shape, theta.shape
computeCost(X, y, theta)
32.072733877455676
def gradientDescent(X, y, theta, alpha, iters):
temp = np.matrix(np.zeros(theta.shape))
parameters = int(theta.ravel().shape[1])
cost = np.zeros(iters)
for i in range(iters):
error = (X * theta.T) - y
for j in range(parameters):
term = np.multiply(error, X[:,j])
temp[0,j] = theta[0,j] - ((alpha / len(X)) * np.sum(term))
theta = temp
cost[i] = computeCost(X, y, theta)
return theta, cost
补充: numpy.zeros(shape, dtype=float)使用方法: ? shape: 创建的新数组的形状(维度); ? dtype: 创建新数组的数据类型;如:dtype=np.int32; ? 返回值: 给定维度的全零数组。
alpha = 0.01
iters = 1000
g, cost = gradientDescent(X, y, theta, alpha, iters)
g
matrix([[-3.24140214, 1.1272942 ]])
computeCost(X, y, g)
4.515955503078914
x = np.linspace(data.Population.min(), data.Population.max(), 100)
f = g[0, 0] + (g[0, 1] * x)
fig, ax = plt.subplots(figsize=(12,8))
ax.plot(x, f, 'r', label='Prediction')
ax.scatter(data.Population, data.Profit, label='Traning Data')
ax.legend(loc=2)
ax.set_xlabel('Population')
ax.set_ylabel('Profit')
ax.set_title('Predicted Profit vs. Population Size')
plt.show()
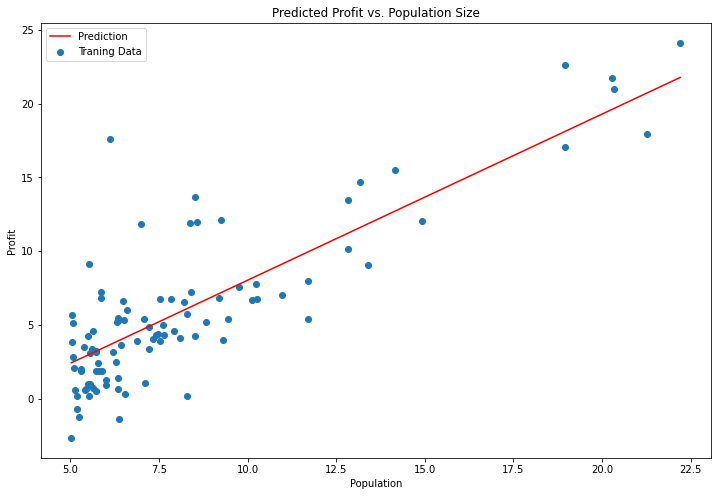
fig, ax = plt.subplots(figsize=(12,8))
ax.plot(np.arange(iters), cost, 'r')
ax.set_xlabel('Iterations')
ax.set_ylabel('Cost')
ax.set_title('Error vs. Training Epoch')
plt.show()
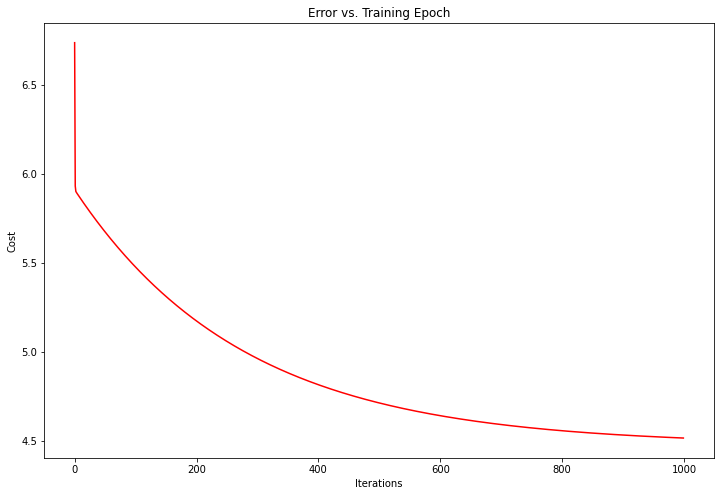
2.多变量线性回归
path = r'C:\Users\Administrator\Desktop\house prise.txt'
data2 = pd.read_csv(path, header=None, names=['Size', 'Bedrooms', 'Price'])
data2.head()
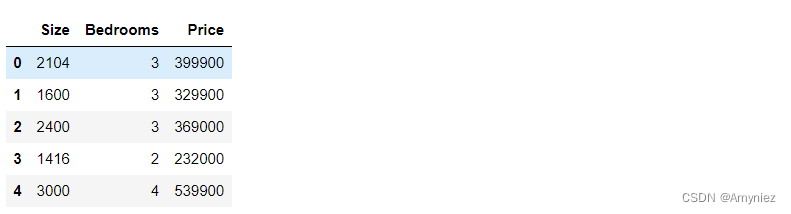
data2 = (data2 - data2.mean()) / data2.std()
data2.head()
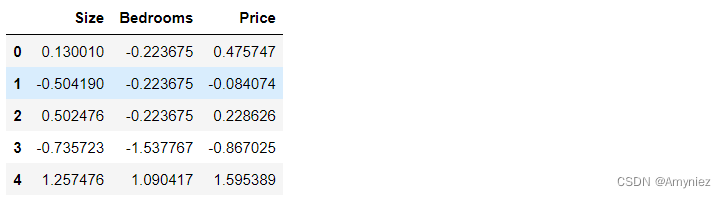
data2.insert(0, 'Ones', 1)
cols = data2.shape[1]
X2 = data2.iloc[:,0:cols-1]
y2 = data2.iloc[:,cols-1:cols]
X2 = np.matrix(X2.values)
y2 = np.matrix(y2.values)
theta2 = np.matrix(np.array([0,0,0]))
g2, cost2 = gradientDescent(X2, y2, theta2, alpha, iters)
computeCost(X2, y2, g2)
0.1307033696077189 此处的代价函数更小
fig, ax = plt.subplots(figsize=(12,8))
ax.plot(np.arange(iters), cost2, 'r')
ax.set_xlabel('Iterations')
ax.set_ylabel('Cost')
ax.set_title('Error vs. Training Epoch')
plt.show()
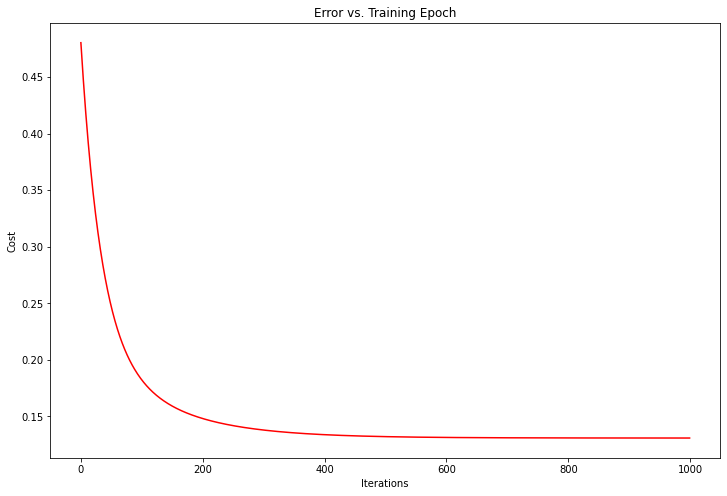
from sklearn import linear_model
model = linear_model.LinearRegression()
model.fit(X, y)
x = np.array(X[:, 1].A1)
f = model.predict(X).flatten()
fig, ax = plt.subplots(figsize=(12,8))
ax.plot(x, f, 'r', label='Prediction')
ax.scatter(data.Population, data.Profit, label='Traning Data')
ax.legend(loc=2)
ax.set_xlabel('Population')
ax.set_ylabel('Profit')
ax.set_title('Predicted Profit vs. Population Size')
plt.show()
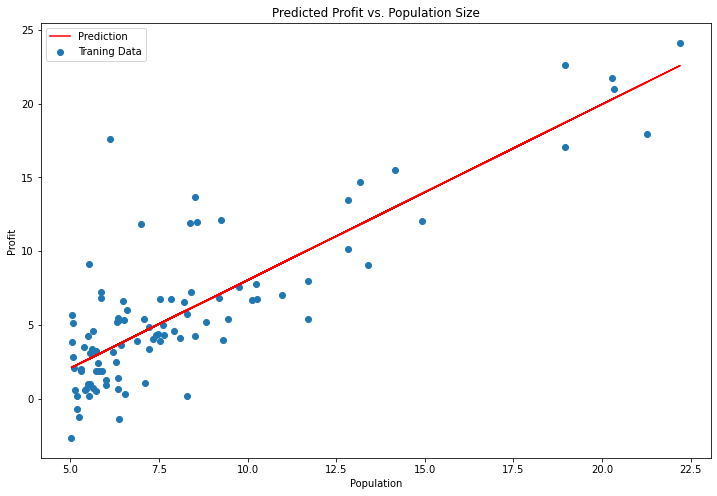
|