推荐系统(十六)多任务学习:腾讯PLE模型(Progressive Layered Extraction model)
推荐系统系列博客:
- 推荐系统(一)推荐系统整体概览
- 推荐系统(二)GBDT+LR模型
- 推荐系统(三)Factorization Machines(FM)
- 推荐系统(四)Field-aware Factorization Machines(FFM)
- 推荐系统(五)wide&deep
- 推荐系统(六)Deep & Cross Network(DCN)
- 推荐系统(七)xDeepFM模型
- 推荐系统(八)FNN模型(FM+MLP=FNN)
- 推荐系统(九)PNN模型(Product-based Neural Networks)
- 推荐系统(十)DeepFM模型
- 推荐系统(十一)阿里深度兴趣网络(一):DIN模型(Deep Interest Network)
- 推荐系统(十二)阿里深度兴趣网络(二):DIEN模型(Deep Interest Evolution Network)
- 推荐系统(十三)阿里深度兴趣网络(三):DSIN模型(Deep Session Interest Network)
- 推荐系统(十四)多任务学习:阿里ESMM(完整空间多任务模型)
- 推荐系统(十五)多任务学习:谷歌MMoE(Multi-gate Mixture-of-Experts )
PLE模型是腾讯发表在RecSys ’20上的文章,这篇paper获得了recsys’20的best paper award,也算为腾讯脱离技术贫民的大业添砖加瓦了。这篇文章号称极大的缓解了多任务学习中存在的两大顽疾:负迁移(negative transfer)现象和跷跷板(seesaw phenomenon),由此带来了相比较其他MTL模型比较大的性能提升。从论文呈现的实验结果也确实是这样的,但从模型结构上来看,更像是大力出奇迹,即性能的提升是由参数量变多而带来的(仅仅是个人看法~)。这篇paper能拿best paper,一方面是实验结果所呈现出来的比较大的性能提升,另一方面是数据分析做的很好,实验做的也很全,因此看起来工作做的很扎实,这是非常值得学习的地方。
本篇博客依然延续之前博客的大纲,会从动机、模型细节结构、代码实现来介绍PLE模型。
一、动机
说到动机,先来说说多任务学习领域中存在的两大问题:
- 负迁移(negative transfer):MTL提出来的目的是为了不同任务,尤其是数据量较少的任务可以借助transfer learning(通过共享embedding,当然你也可以不仅共享embedding,再往上共享基层全连接网络等等这些很常见的操作)。但经常事与愿违,当两个任务之间的相关性很弱(比如一个任务是判断一张图片是否是狗,另一个任务是判断是否是飞机)或者非常复杂时,往往发生负迁移,即共享了之后效果反而很差,还不如不共享。
- 跷跷板现象:还是当两个task之间相关性很弱或者很复杂时,往往出现的现象是:一个task性能的提升是通过损害另一个task的性能做到的。这种现象存在很久,PLE论文里给它起了个非常贴切的名字『跷跷板』,想象一下你小时候玩跷跷板的情形吧,胖子把瘦子跷起来。
PLE这篇论文,通过大量的实验发现,当前的MTL模型跷跷板现象非常严重(从数据实验中发现问题,进而提出解决办法,会让你的motivation显得非常扎实)。如下图所示,这里的VTR(View-Through Rate)是有效观看率,其定义是用户观看某个视频超过一定时间即认为是一次有效观看,所以是个二分类任务;VCR(View Completion Ratio)是视频观看完成率,是一个回归任务。下面这个图越靠近右上角说明模型在两个task上表现都比较好,左下角则是都很差,因此明显看出,目前MTL领域中主流的模型基本上都存在跷跷板问题(至少在腾讯视频的数据场景下),表现比较好的也就是上一篇博客中介绍的谷歌的MMoE,但还是存在。 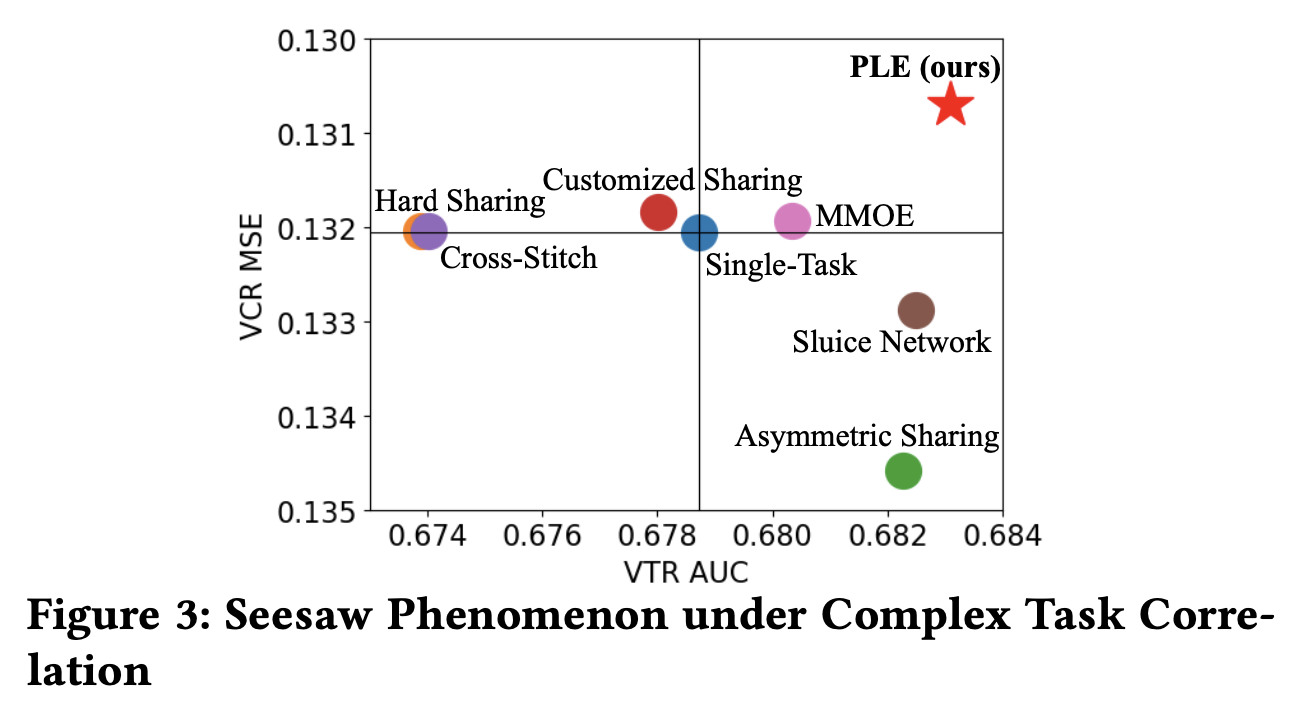
二、PLE模型细节
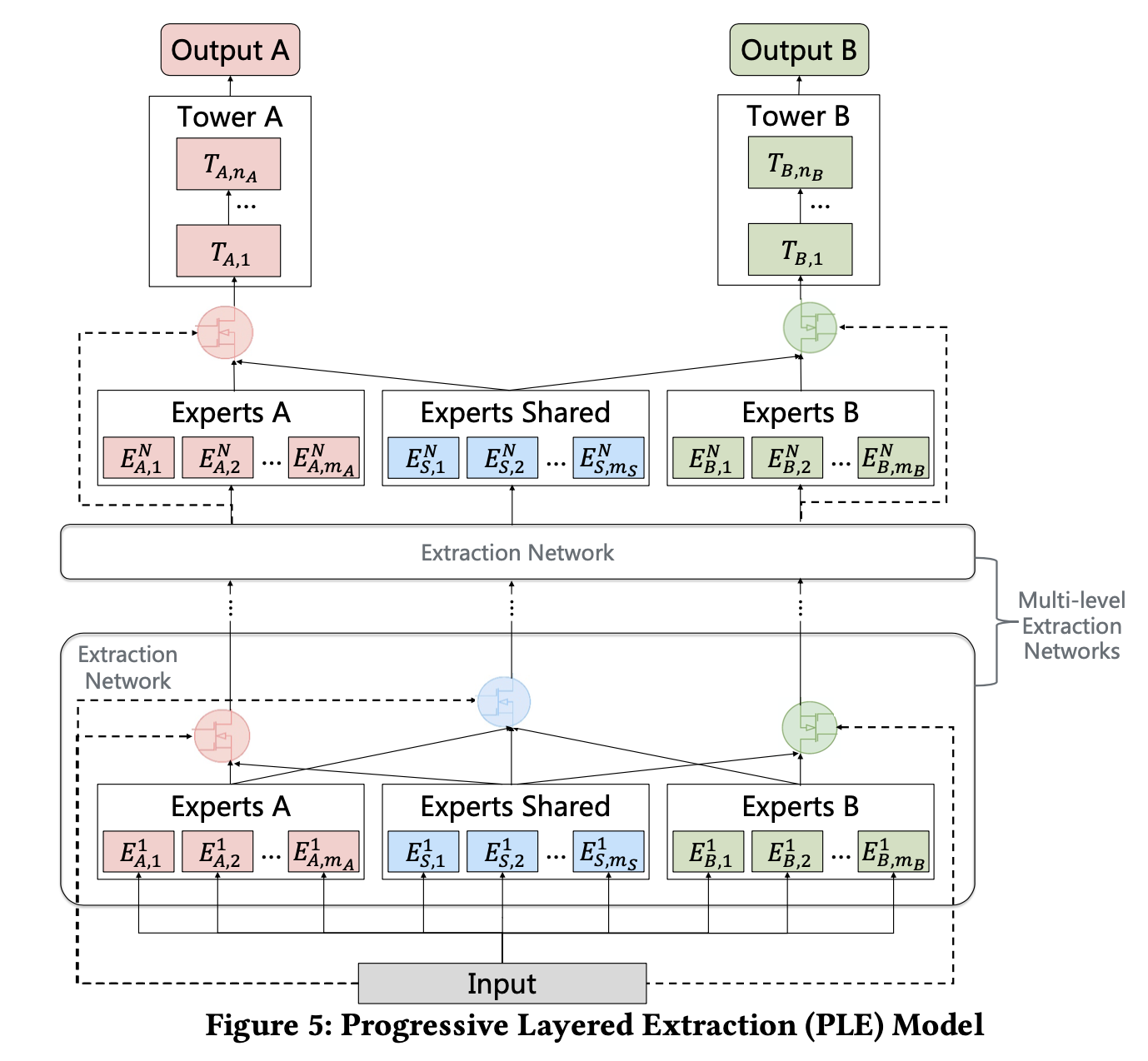 先来从整体上看看这个模型,大家自行对比下MMoE(参见我的博客推荐系统(十五)多任务学习:谷歌MMoE(Multi-gate Mixture-of-Experts )),可能就能体会到我前面说PLE性能提升更像是复杂参数所带来的这句话了,因为粗略的看,PLE做了deep化(expert完后再来一层expert),显然要比浅层的效果来得好。
我们来自底向上的拆解下PLE:
2.1、Extraction Network
PLE这里相比较MMoE做了比较大的创新,在MMoE里,不同task通过gate(网络)共享相同的expert(网络),而PLE中则把expert分为了两种:共享的expert(即上图中的experts Shared)和每个task单独的expert(task-specific experts)。因此,这种设计即保留了transfer learning(通过共享expert)能力,又能够避免有害参数的干扰(避免negative transfer)。
同样的,在gate网络部分,也分为了task-specific和Shared,以上图左边的这个gate(即上图中红色的gate)为例,它的输入有两部分,分别为Experts A和Experts Shared。而shared gate(上图中蓝颜色的gate)的输入则为三部分:Experts A、Experts Shared和Experts B。最终三部分直接作为下一层的输入,分别对应到下一层的Experts A、Experts Shared和Experts B。有一说一,图figure5如果把中间那个extraction network去掉,会更加清晰。算了,原来想偷个懒,不想画图的,我还是上个简化版的图吧。 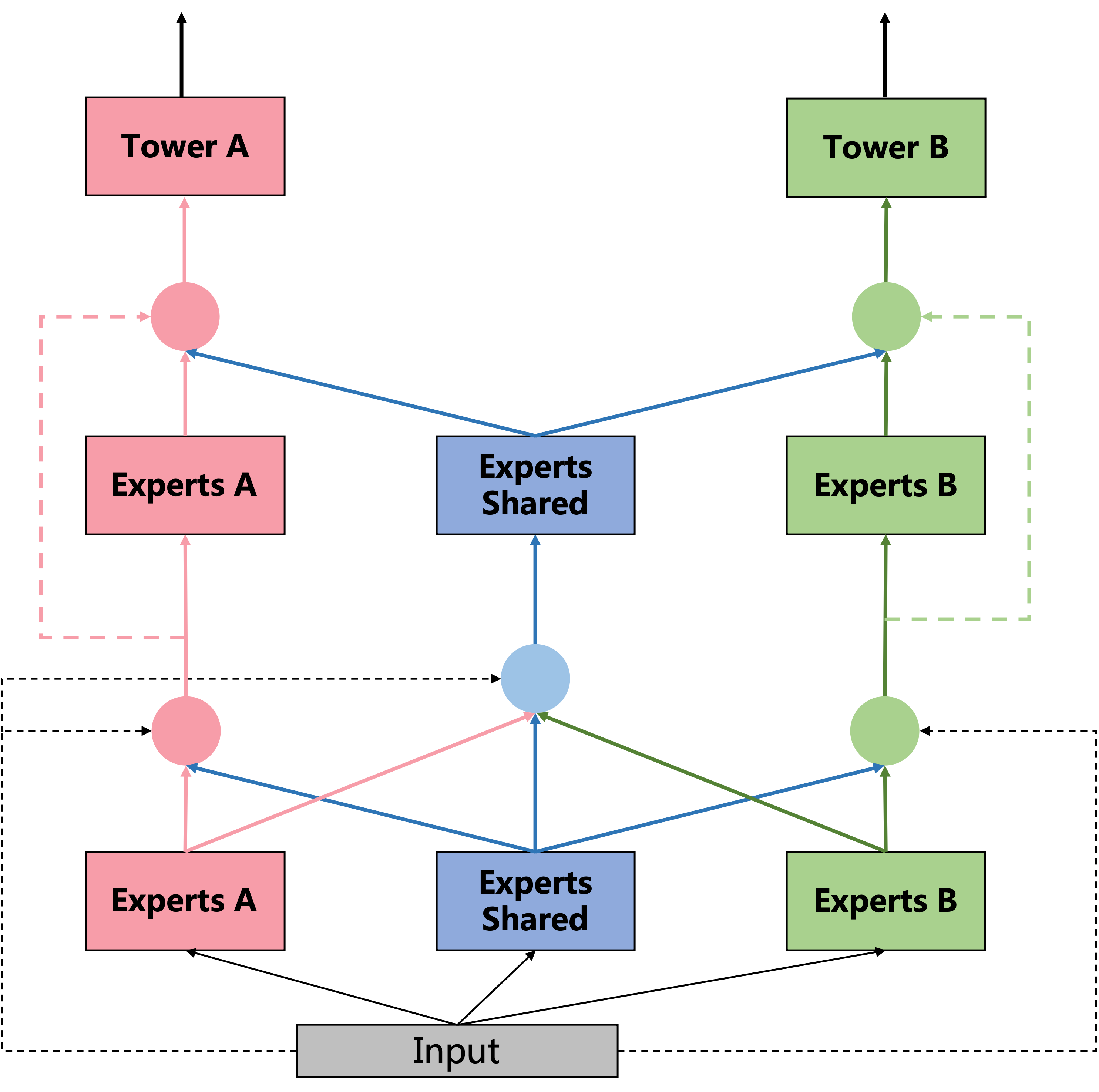
图3. PLE模型简化版
额,上完这个简化版的图后,我觉得都不用在写文字了…因为再多的文字也是苍白。
2.2、其他一些细节
- 腾讯视频rerank模块的公式为:
s
c
o
r
e
=
P
V
T
R
W
V
T
R
×
P
V
C
R
W
V
C
R
×
P
S
H
R
W
S
H
R
×
.
.
.
×
P
C
M
R
W
C
M
R
×
f
(
v
i
d
e
o
_
l
e
n
)
score={P_{VTR}}^{W_{VTR}} \times {P_{VCR}}^{W_{VCR}} \times {P_{SHR}}^{W_{SHR}} \times ... \times{P_{CMR}}^{W_{CMR}} \times f(video\_len)
score=PVTR?WVTR?×PVCR?WVCR?×PSHR?WSHR?×...×PCMR?WCMR?×f(video_len),其中,VCR(View Completion Ratio)为视频观看完成率,VTR(View- Through Rate)为视频是否为有效观看,CMR(Comment Rate)为评论率,SHR(Share Rate)为分享率。
W
W
W为权重因子,用于调整每个指标的权重。
- 关于loss函数,每个task的loss加了个可学习的权重参数
w
w
w,用于模型自动学习每个task的loss的权重。
三、代码实现
老规矩,直接上paddle给出的实现代码,我这里把向量维度加了注释,方便理解。
组网代码:
import paddle
import paddle.nn as nn
import paddle.nn.functional as F
class PLELayer(nn.Layer):
def __init__(self, feature_size, task_num, exp_per_task, shared_num,
expert_size, tower_size, level_number):
super(PLELayer, self).__init__()
"""
feature_size: 499
"""
self.task_num = task_num
self.exp_per_task = exp_per_task
self.shared_num = shared_num
self.expert_size = expert_size
self.tower_size = tower_size
self.level_number = level_number
"""
ple_layers:
[SinglePLELayer(
(lev_0_exp_0_0): Linear(in_features=499, out_features=16, dtype=float32, name=lev_0_exp_0_0)
(lev_0_exp_0_1): Linear(in_features=499, out_features=16, dtype=float32, name=lev_0_exp_0_1)
(lev_0_exp_0_2): Linear(in_features=499, out_features=16, dtype=float32, name=lev_0_exp_0_2)
(lev_0_exp_1_0): Linear(in_features=499, out_features=16, dtype=float32, name=lev_0_exp_1_0)
(lev_0_exp_1_1): Linear(in_features=499, out_features=16, dtype=float32, name=lev_0_exp_1_1)
(lev_0_exp_1_2): Linear(in_features=499, out_features=16, dtype=float32, name=lev_0_exp_1_2)
(lev_0_exp_shared_0): Linear(in_features=499, out_features=16, dtype=float32, name=lev_0_exp_shared_0)
(lev_0_exp_shared_1): Linear(in_features=499, out_features=16, dtype=float32, name=lev_0_exp_shared_1)
(lev_0_gate_0): Linear(in_features=499, out_features=5, dtype=float32, name=lev_0_gate_0)
(lev_0_gate_1): Linear(in_features=499, out_features=5, dtype=float32, name=lev_0_gate_1)
(lev_0_gate_shared_): Linear(in_features=499, out_features=8, dtype=float32, name=lev_0_gate_shared_)
(_param_gate_shared): Linear(in_features=499, out_features=8, dtype=float32, name=lev_0_gate_shared_)),
SinglePLELayer(
(lev_1_exp_0_0): Linear(in_features=16, out_features=16, dtype=float32, name=lev_1_exp_0_0)
(lev_1_exp_0_1): Linear(in_features=16, out_features=16, dtype=float32, name=lev_1_exp_0_1)
(lev_1_exp_0_2): Linear(in_features=16, out_features=16, dtype=float32, name=lev_1_exp_0_2)
(lev_1_exp_1_0): Linear(in_features=16, out_features=16, dtype=float32, name=lev_1_exp_1_0)
(lev_1_exp_1_1): Linear(in_features=16, out_features=16, dtype=float32, name=lev_1_exp_1_1)
(lev_1_exp_1_2): Linear(in_features=16, out_features=16, dtype=float32, name=lev_1_exp_1_2)
(lev_1_exp_shared_0): Linear(in_features=16, out_features=16, dtype=float32, name=lev_1_exp_shared_0)
(lev_1_exp_shared_1): Linear(in_features=16, out_features=16, dtype=float32, name=lev_1_exp_shared_1)
(lev_1_gate_0): Linear(in_features=16, out_features=5, dtype=float32, name=lev_1_gate_0)
(lev_1_gate_1): Linear(in_features=16, out_features=5, dtype=float32, name=lev_1_gate_1))]
"""
self.ple_layers = []
for i in range(0, self.level_number):
if i == self.level_number - 1:
ple_layer = self.add_sublayer(
name='lev_' + str(i),
sublayer=SinglePLELayer(
feature_size, task_num, exp_per_task, shared_num,
expert_size, 'lev_' + str(i), True))
self.ple_layers.append(ple_layer)
break
else:
ple_layer = self.add_sublayer(
name='lev_' + str(i),
sublayer=SinglePLELayer(
feature_size, task_num, exp_per_task, shared_num,
expert_size, 'lev_' + str(i), False))
self.ple_layers.append(ple_layer)
feature_size = expert_size
self._param_tower = []
self._param_tower_out = []
for i in range(0, self.task_num):
linear = self.add_sublayer(
name='tower_' + str(i),
sublayer=nn.Linear(
expert_size,
tower_size,
weight_attr=nn.initializer.Constant(value=0.1),
bias_attr=nn.initializer.Constant(value=0.1),
name='tower_' + str(i)))
self._param_tower.append(linear)
linear = self.add_sublayer(
name='tower_out_' + str(i),
sublayer=nn.Linear(
tower_size,
2,
weight_attr=nn.initializer.Constant(value=0.1),
bias_attr=nn.initializer.Constant(value=0.1),
name='tower_out_' + str(i)))
self._param_tower_out.append(linear)
def forward(self, input_data):
"""
input_data: Tensor(shape=[2, 499], 2--->batch_size
"""
inputs_ple = []
for i in range(0, self.task_num + 1):
inputs_ple.append(input_data)
ple_out = []
for i in range(0, self.level_number):
print "^^^^^number: %d^^^^^^"%i
ple_out = self.ple_layers[i](inputs_ple)
inputs_ple = ple_out
output_layers = []
for i in range(0, self.task_num):
cur_tower = self._param_tower[i](ple_out[i])
cur_tower = F.relu(cur_tower)
out = self._param_tower_out[i](cur_tower)
out = F.softmax(out)
out = paddle.clip(out, min=1e-15, max=1.0 - 1e-15)
output_layers.append(out)
return output_layers
class SinglePLELayer(nn.Layer):
def __init__(self, input_feature_size, task_num, exp_per_task, shared_num,
expert_size, level_name, if_last):
super(SinglePLELayer, self).__init__()
self.task_num = task_num
self.exp_per_task = exp_per_task
self.shared_num = shared_num
self.expert_size = expert_size
self.if_last = if_last
self._param_expert = []
for i in range(0, self.task_num):
for j in range(0, self.exp_per_task):
linear = self.add_sublayer(
name=level_name + "_exp_" + str(i) + "_" + str(j),
sublayer=nn.Linear(
input_feature_size,
expert_size,
weight_attr=nn.initializer.Constant(value=0.1),
bias_attr=nn.initializer.Constant(value=0.1),
name=level_name + "_exp_" + str(i) + "_" + str(j)))
self._param_expert.append(linear)
for i in range(0, self.shared_num):
linear = self.add_sublayer(
name=level_name + "_exp_shared_" + str(i),
sublayer=nn.Linear(
input_feature_size,
expert_size,
weight_attr=nn.initializer.Constant(value=0.1),
bias_attr=nn.initializer.Constant(value=0.1),
name=level_name + "_exp_shared_" + str(i)))
self._param_expert.append(linear)
self._param_gate = []
cur_expert_num = self.exp_per_task + self.shared_num
for i in range(0, self.task_num):
linear = self.add_sublayer(
name=level_name + "_gate_" + str(i),
sublayer=nn.Linear(
input_feature_size,
cur_expert_num,
weight_attr=nn.initializer.Constant(value=0.1),
bias_attr=nn.initializer.Constant(value=0.1),
name=level_name + "_gate_" + str(i)))
self._param_gate.append(linear)
if not if_last:
cur_expert_num = self.task_num * self.exp_per_task + self.shared_num
linear = self.add_sublayer(
name=level_name + "_gate_shared_",
sublayer=nn.Linear(
input_feature_size,
cur_expert_num,
weight_attr=nn.initializer.Constant(value=0.1),
bias_attr=nn.initializer.Constant(value=0.1),
name=level_name + "_gate_shared_"))
self._param_gate_shared = linear
def forward(self, input_data):
print "****SinglePLELayer forward****"
expert_outputs = []
"""
_param_expert: level0:
[Linear(in_features=499, out_features=16, dtype=float32, name=lev_0_exp_0_0),
Linear(in_features=499, out_features=16, dtype=float32, name=lev_0_exp_0_1),
Linear(in_features=499, out_features=16, dtype=float32, name=lev_0_exp_0_2),
Linear(in_features=499, out_features=16, dtype=float32, name=lev_0_exp_1_0),
Linear(in_features=499, out_features=16, dtype=float32, name=lev_0_exp_1_1),
Linear(in_features=499, out_features=16, dtype=float32, name=lev_0_exp_1_2),
Linear(in_features=499, out_features=16, dtype=float32, name=lev_0_exp_shared_0),
Linear(in_features=499, out_features=16, dtype=float32, name=lev_0_exp_shared_1)]
level1:
[Linear(in_features=16, out_features=16, dtype=float32, name=lev_1_exp_0_0),
Linear(in_features=16, out_features=16, dtype=float32, name=lev_1_exp_0_1),
Linear(in_features=16, out_features=16, dtype=float32, name=lev_1_exp_0_2),
Linear(in_features=16, out_features=16, dtype=float32, name=lev_1_exp_1_0),
Linear(in_features=16, out_features=16, dtype=float32, name=lev_1_exp_1_1),
Linear(in_features=16, out_features=16, dtype=float32, name=lev_1_exp_1_2),
Linear(in_features=16, out_features=16, dtype=float32, name=lev_1_exp_shared_0),
Linear(in_features=16, out_features=16, dtype=float32, name=lev_1_exp_shared_1)]
"""
for i in range(0, self.task_num):
for j in range(0, self.exp_per_task):
linear_out = self._param_expert[i * self.task_num + j](
input_data[i])
expert_output = F.relu(linear_out)
expert_outputs.append(expert_output)
for i in range(0, self.shared_num):
linear_out = self._param_expert[self.exp_per_task * self.task_num + i](input_data[-1])
expert_output = F.relu(linear_out)
expert_outputs.append(expert_output)
outputs = []
"""
self._param_gate:
[Linear(in_features=499, out_features=5, dtype=float32, name=lev_0_gate_0),
Linear(in_features=499, out_features=5, dtype=float32, name=lev_0_gate_1)]
"""
for i in range(0, self.task_num):
cur_expert_num = self.exp_per_task + self.shared_num
linear_out = self._param_gate[i](input_data[i])
cur_gate = F.softmax(linear_out)
cur_gate = paddle.reshape(cur_gate, [-1, cur_expert_num, 1])
cur_experts = expert_outputs[i * self.exp_per_task:(i + 1) * self.exp_per_task] + \
expert_outputs[-int(self.shared_num):]
expert_concat = paddle.concat(x=cur_experts, axis=1)
expert_concat = paddle.reshape(
expert_concat, [-1, cur_expert_num, self.expert_size])
cur_gate_expert = paddle.multiply(x=expert_concat, y=cur_gate)
cur_gate_expert = paddle.sum(x=cur_gate_expert, axis=1)
outputs.append(cur_gate_expert)
if not self.if_last:
cur_expert_num = self.task_num * self.exp_per_task + self.shared_num
linear_out = self._param_gate_shared(input_data[-1])
cur_gate = F.softmax(linear_out)
cur_gate = paddle.reshape(cur_gate, [-1, cur_expert_num, 1])
cur_experts = expert_outputs
expert_concat = paddle.concat(x=cur_experts, axis=1)
expert_concat = paddle.reshape(
expert_concat, [-1, cur_expert_num, self.expert_size])
cur_gate_expert = paddle.multiply(x=expert_concat, y=cur_gate)
cur_gate_expert = paddle.sum(x=cur_gate_expert, axis=1)
outputs.append(cur_gate_expert)
return outputs
loss函数代码:
def net(self, inputs, is_infer=False):
input_data = inputs[0]
label_income = inputs[1]
label_marital = inputs[2]
PLE = PLELayer(self.feature_size, self.task_num, self.exp_per_task,
self.shared_num, self.expert_size, self.tower_size,
self.level_number)
pred_income, pred_marital = PLE.forward(input_data)
pred_income_1 = paddle.slice(
pred_income, axes=[1], starts=[1], ends=[2])
pred_marital_1 = paddle.slice(
pred_marital, axes=[1], starts=[1], ends=[2])
auc_income, batch_auc_1, auc_states_1 = paddle.static.auc(
input=pred_income,
label=paddle.cast(
x=label_income, dtype='int64'))
auc_marital, batch_auc_2, auc_states_2 = paddle.static.auc(
input=pred_marital,
label=paddle.cast(
x=label_marital, dtype='int64'))
if is_infer:
fetch_dict = {'auc_income': auc_income, 'auc_marital': auc_marital}
return fetch_dict
cost_income = paddle.nn.functional.log_loss(
input=pred_income_1,
label=paddle.cast(
label_income, dtype="float32"))
cost_marital = paddle.nn.functional.log_loss(
input=pred_marital_1,
label=paddle.cast(
label_marital, dtype="float32"))
avg_cost_income = paddle.mean(x=cost_income)
avg_cost_marital = paddle.mean(x=cost_marital)
cost = avg_cost_income + avg_cost_marital
self._cost = cost
fetch_dict = {
'cost': cost,
'auc_income': auc_income,
'auc_marital': auc_marital
}
return fetch_dict
参考文献
[1]: Tang H , Liu J , Zhao M , et al. Progressive Layered Extraction (PLE): A Novel Multi-Task Learning (MTL) Model for Personalized Recommendations[C]// RecSys '20: Fourteenth ACM Conference on Recommender Systems. ACM, 2020.
|