2022/5/2日报
Label Enhancement with Sample Correlations via Low-Rank Representation
motivation
The downside of these partial-based processes for the graph construction of each instance is that only local topological features have been taken advantage of, and the holistic information of the feature space has been largely untapped. In addition, these approaches always require prior knowledge for hyperparameters.
提出
一种全局挖掘整个特征空间的全局结构并对参数具有鲁棒性的方法。
mapping model
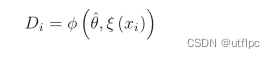
low-rank representation (LRR)
低秩表示(LRR)对数据子空间表示施加低秩约束以捕获所有实例的全局关系,通过从全局角度利用特征空间的结构来使 LE 受益 直观地说,构建的低秩结构通常可以平滑地转移到标签空间,并利用特征空间的最低秩表示来表示标签分布的LRR。 因此,作者将获得的 LRR 合并到目标函数中,以探索标签分布空间中的隐藏线索。
-
Liu, G., and Yan, S. 2011. Latent low-rank representation for subspace segmentation and feature extraction. In 2011 International Conference on Computer Vision, 1615–1622. IEEE -
Yin, M.; Gao, J.; and Lin, Z. 2015. Laplacian regular- ized low-rank representation and its applications. IEEE transactions on pattern analysis and machine intelligence 38(3):504–517. -
Zhai, L.; Zhu, J.; Zheng, Q.; Pang, S.; Li, Z.; and Wang, J. 2019. Multi-view spectral clustering via partial sum minimi- sation of singular values. Electronics Letters 55(6):314–316.
2022/5/3日报
θ
\theta
θ的目标函数

least-squares (LS) loss function
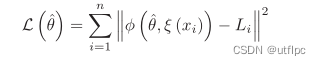
这是恢复的标签分布和逻辑标签之间的损失函数,该损失函数为最小二乘(LS)损失函数
标签分布的低秩恢复

C
^
\hat{C}
C^是特征空间的最小 LRR
augmented Lagrange multiplier (ALM) approach
Liu, G.; Lin, Z.; Yan, S.; Sun, J.; Yu, Y.; and Ma, Y. 2012. Robust recovery of subspace structures by low-rank representation. IEEE transactions on pattern analysis and machine intelligence 35(1):171–184.
limited memory BFGS (L-BFGS)
Nocedal, J., and Wright, S. 2006. Numerical optimization. Springer Science & Business Media.
2022/5/4日报
regularized rank minimization problem
特征空间中的最小 LRR由下列式子得到 
C
?
C^?
C? 是特征 X 相对于变量 C 的所谓“LRR”,E 表示特定于样本的损坏,λ2 是平衡两部分之间影响的低秩系数 以上凸优化问题通过增强拉格朗日乘数 (ALM) 方法来解决,当然还有很多其他方法
得到最优的
C
^
\hat{C}
C^后,
θ
\theta
θ的目标函数变为: 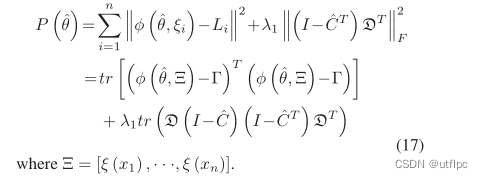 该目标函数的最小化将通过 limited memory BFGS (L-BFGS)得到
|