1.出处来源
论文来源: Mei Zheng, Fan Min, Heng-Ru Zhang, Wen-Bin Chen, Fast recommendations with the M-distance, IEEE Access 4 (2016) 1464–1468 的源代码. 点击下载论文.
通过下面这张图来观察:
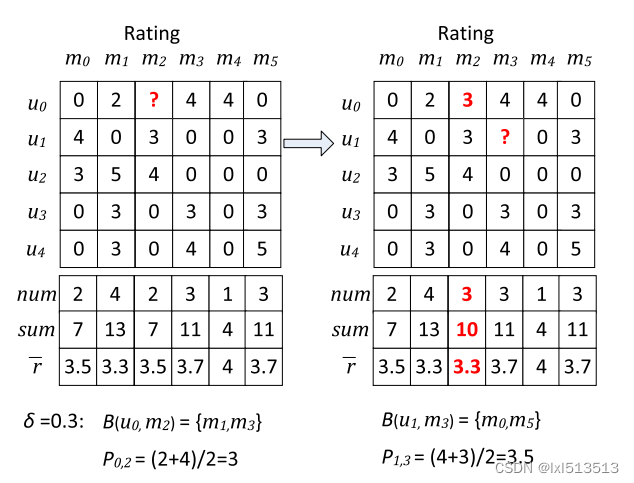 图解:u0,u1,…是代表不同的用户,m0,m1,…是代表不同的项目,图中的数据的用户对不同项目的评分,现在我们根据M-distance来估计出? 所在位置的得分。首先计算出每一种项目所得的平均分,同时也要计算出未知分所在的项目的均分,然后我们在这个未知项目的周围找到误差满足在0.3以内的项目,然后根据未知分对应的用户对满足那个误差的项目的评分就平均值,该平均值我们估计就是为未知分数的值。 我们以右边的这个表为例,首先r就是提前算出来的那个平均值,我们需要求的未知分数(u1,m3)所在的平均数是3.7,我们要找到满足误差在0.3以内的项目平均分,那就是项目m0,m5,然后未知分数对应的用户是u1,然后u1对m0,m5的评分分别是4,3,平均分为(4+3)/2=3.5,所以我们估计未知分数为3.5。
2.基于M-distance的推荐
下面以不同用户对不同电影的评分为例,然后将数据存储在 https://github.com/FanSmale/sampledata/ 中 movielens-943u1682m.txt. 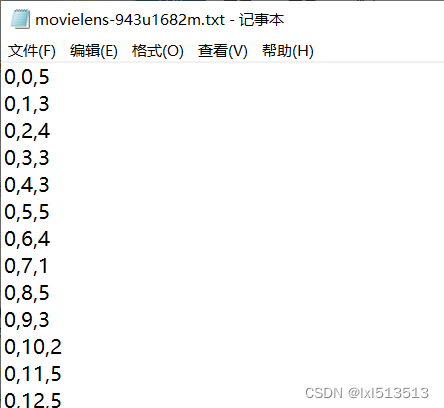 文档中,一共943个用户,1682部电影,10000条评分记录 0,11,5表示:用户0对电影11的评分是5
3.代码实现
3.1变量解释
public static final double DEFAULT_RATING = 3.0;
private int numUsers;
private int numItems;
private int numRatings;
private double[] predictions;
private int[][] compressedRatingMatrix;
private int[] userDegrees;
private double[] userAverageRatings;
private int[] itemDegrees;
private double[] itemAverageRatings;
private int[] userStartingIndices;
private int numNonNeighbors;
private double radius;
3.2构造方法
主要是完成一些数据的初始化
- 首先传入4个变量,分别是文件路径,用户数量,电影数量,记录数量
public MBR(String paraFilename, int paraNumUsers, int paraNumItems, int paraNumRatings) throws Exception {
numItems = paraNumItems;
numUsers = paraNumUsers;
numRatings = paraNumRatings;
userDegrees = new int[numUsers];
userStartingIndices = new int[numUsers + 1];
userAverageRatings = new double[numUsers];
itemDegrees = new int[numItems];
compressedRatingMatrix = new int[numRatings][3];
itemAverageRatings = new double[numItems];
predictions = new double[numRatings];
System.out.println("Reading " + paraFilename);
File tempFile = new File(paraFilename);
if (!tempFile.exists()) {
System.out.println("File " + paraFilename + " does not exists.");
System.exit(0);
}
BufferedReader tempBufReader = new BufferedReader(new FileReader(tempFile));
String tempString;
String[] tempStrArray;
int tempIndex = 0;
userStartingIndices[0] = 0;
userStartingIndices[numUsers] = numRatings;
while ((tempString = tempBufReader.readLine()) != null) {
tempStrArray = tempString.split(",");
compressedRatingMatrix[tempIndex][0] = Integer.parseInt(tempStrArray[0]);
compressedRatingMatrix[tempIndex][1] = Integer.parseInt(tempStrArray[1]);
compressedRatingMatrix[tempIndex][2] = Integer.parseInt(tempStrArray[2]);
userDegrees[compressedRatingMatrix[tempIndex][0]]++;
itemDegrees[compressedRatingMatrix[tempIndex][1]]++;
if (tempIndex > 0) {
if (compressedRatingMatrix[tempIndex][0] != compressedRatingMatrix[tempIndex - 1][0]) {
userStartingIndices[compressedRatingMatrix[tempIndex][0]] = tempIndex;
}
}
tempIndex++;
}
tempBufReader.close();
double[] tempUserTotalScore = new double[numUsers];
double[] tempItemTotalScore = new double[numItems];
for (int i = 0; i < numRatings; i++) {
tempUserTotalScore[compressedRatingMatrix[i][0]] += compressedRatingMatrix[i][2];
tempItemTotalScore[compressedRatingMatrix[i][1]] += compressedRatingMatrix[i][2];
}
for (int i = 0; i < numUsers; i++) {
userAverageRatings[i] = tempUserTotalScore[i] / userDegrees[i];
}
for (int i = 0; i < numItems; i++) {
itemAverageRatings[i] = tempItemTotalScore[i] / itemDegrees[i];
}
}
3.3 核心代码
public void leaveOneOutPrediction() {
double tempItemAverageRating;
int tempUser, tempItem, tempRating;
System.out.println("\r\nLeaveOneOutPrediction for radius " + radius);
numNonNeighbors = 0;
for (int i = 0; i < numRatings; i++) {
tempUser = compressedRatingMatrix[i][0];
tempItem = compressedRatingMatrix[i][1];
tempRating = compressedRatingMatrix[i][2];
tempItemAverageRating = (itemAverageRatings[tempItem] * itemDegrees[tempItem] -tempRating)/ (itemDegrees[tempItem] - 1);
int tempNeighbors = 0;
double tempTotal = 0;
int tempComparedItem;
for (int j = userStartingIndices[tempUser]; j < userStartingIndices[tempUser + 1]; j++) {
tempComparedItem = compressedRatingMatrix[j][1];
if (tempItem == tempComparedItem) {
continue;
}
if (Math.abs(tempItemAverageRating - itemAverageRatings[tempComparedItem]) < radius) {
tempTotal += compressedRatingMatrix[j][2];
tempNeighbors++;
}
}
if (tempNeighbors > 0) {
predictions[i] = tempTotal / tempNeighbors;
} else {
predictions[i] = DEFAULT_RATING;
numNonNeighbors++;
}
}
}
3.4 算法评价
MAE(平均绝对误差)
public double computeMAE() throws Exception {
double tempTotalError = 0;
for (int i = 0; i < predictions.length; i++) {
tempTotalError += Math.abs(predictions[i] - compressedRatingMatrix[i][2]);
}
return tempTotalError / predictions.length;
}
RMSE(平方根误差)
public double computeRSME() throws Exception {
double tempTotalError = 0;
for (int i = 0; i < predictions.length; i++) {
tempTotalError += (predictions[i] - compressedRatingMatrix[i][2]) * (predictions[i] - compressedRatingMatrix[i][2]);
}
double tempAverage = tempTotalError / predictions.length;
return Math.sqrt(tempAverage);
}
4.运行结果
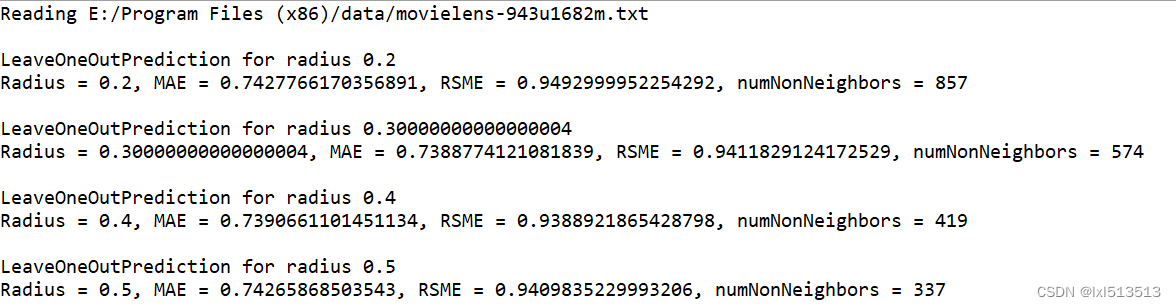
|