目录
一、方法介绍
1.1TarNet
1.1.1TarNet
1.1.2网络结构
1.1.3counterfactual loss
1.1.4代码实现
1.2Dragonet
1.3DRNet
1.4VCNet
VCNET AND FUNCTIONAL TARGETED REGULARIZATION FOR LEARNING CAUSAL EFFECTS OF CONTINUOUS TREATMENTS
二、待补充
一、方法介绍
1.1TarNet
1.1.1TarNet
????????S-Learner和T-Learner都不太好,因为S-Learner是把T和W一起训练,在高维情况下很容易忽视T的影响(too much bias),而T-Learner是各自对T=0和T=1训练两个独立的模型,这样会造成过高的方差(too much variance),与因果关系不大。TARNet是对所有数据进行训练,但是在后面分成了两个branch。
本质:多任务学习。
多个treatment分别建模
1.1.2网络结构
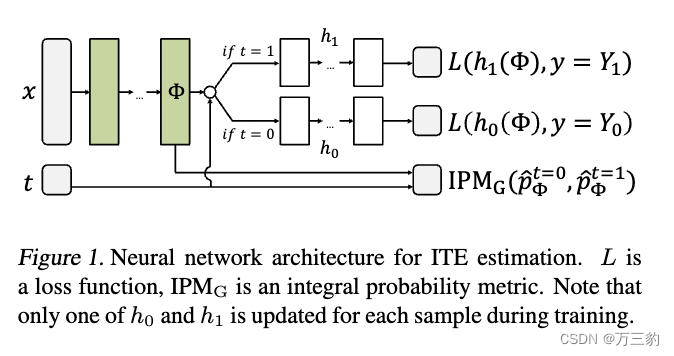
我们关注的是这样一种情况:因果关系很简单,已知为(Y1, Y0) ⊥x | t,,没有隐藏的混杂因素。在我们假设的因果模型下,最常见的目标是因果效应推理所使用的应用科学是求得平均处理效果: 。
我们的结果的一个观点是,当使用协变量调整来估计ITE时,我们指出了一个以前未被解释的方差来源。我们提出了一种新型的正则化,通过学习表示,减少了被处理和对照之间的IPM距离,实现了一种新型的偏差-方差权衡。
考虑到我们的observational的训练样本可能存在针对t的imbalance(t和x不独立)的情况,举个例子:我们想要知道一个药对某个病的作用,由于这个药比较贵,我们采集到的样本用这个药的大部分人是富人,不用这个药的大部分人是穷人,如果说富和穷是X的一个特征,用不用药是t,这个时候如果拟合出来的h1和h0就不准了,特别是h1对穷人,h0对富人都不准。什么时候准呢?当样本来自于随机试验的时候才准,但是我们现在又只有observational data,没办法做试验,所以我们只能对样本的分布进行一个调整。
另一种广泛应用于因果推理的统计方法是加权法。逆向倾向评分加权(Austin, 2011)等方法重新加权观察数据中的单位,以使处理人群和对照组人群更具可比性,并已用于估计条件效应(Cole et al., 2003)。主要的挑战,特别是在高维情况下,是控制估计的方差(Swaminathan & Joachims, 2015)。双稳健方法更进一步,结合倾向评分重加权和c中的协变量调整。
我们的工作与上述所有工作的不同之处在于,我们关注的是估计个体治疗效果的泛化误差方面,而不是渐近一致性,并且我们只关注观察性研究案例,没有随机成分或工具变量。
特别是,估计ITE需要预测与观测到的分布不同的结果。我们的ITE误差上界与bdavidet al.(2007)给出的领域适应的泛化界有相似之处;Mansour et al. (2009);Ben-David等人(2010);Cortes & Mohri(2014)。这些边界采用分布距离度量,如a距离或差异度量,这与我们使用的IPM距离有关。我们的算法类似于Ganin等人(2016)最近提出的领域自适应算法,原则上也类似于其他领域自适应方法(例如Daum ' e III (2007);Pan et al. (2011);Sun et al.(2016))可以适用于这里提出的ITE估计。
在本文中,我们专注于一个相关但更实质性的任务:估计个人治疗效果,建立在反事实错误项。我们提供了关于表示的绝对质量的信息约束。我们还推导了一个更灵活的算法家族,包括非线性假设和ipm形式的更强大的分布度量,如Wasserstein和MMD距离。最后,我们进行了更彻底的实验,包括真实世界的数据集和样本外性能,并表明我们的方法优于先前提出的方法。
1.1.3counterfactual loss
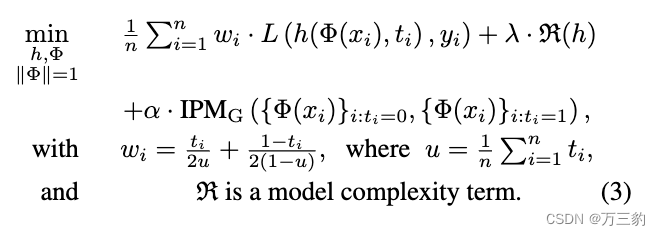
第一项wi的作用:对于treatment group和control group的样本数量不平均的情况做一个修正,使得他们在损失函数中的权重平衡。
第二项是模型复杂度的一个正则惩罚
第三项就是刚刚提到的对representation imbalance的一个修正。其中α用来控制这个修正的力度。当α>0时,这个模型就叫做CFR (Conterfactual Regression),当α=0时,则叫做TARNet (Treatment-Agnostic Representation Network)。实际上效果论文里是CFR好一些。 ?
1.1.4代码实现
import tensorflow as tf
from ..layers.gather import Gather_Streams
from ..layers.split import Split_Streams
from ..layers.MLP import MLP
from pickle import load
from typing import Any
class TARNet(tf.keras.Model):
"""Return a tarnet sub KERAS API model."""
def __init__(
self,
normalizer_layer: tf.keras.layers.Layer = None,
n_treatments: int = 2,
output_dim: int = 1,
phi_layers: int = 2,
units:int = 20,
y_layers: int = 3,
activation: str = "relu",
reg_l2: float = 0.0,
treatment_as_input: bool = False,
scaler: Any = None,
output_bias: float = None,
):
"""Initialize the layers used by the model.
Args:
normalizer_layer (tf.keras.layer, optional): _description_. Defaults to None.
n_treatments (int, optional): _description_. Defaults to 2.
output_dim (int, optional): _description_. Defaults to 1.
phi_layers (int, optional): _description_. Defaults to 2.
y_layers (int, optional): _description_. Defaults to 3.
activation (str, optional): _description_. Defaults to "relu".
reg_l2 (float, optional): _description_. Defaults to 0.0.
"""
super(TARNet, self).__init__()
# uniform quantile transform for treatment
self.scaler = scaler if scaler else load(open("scaler.pkl", "rb"))
# input normalization layer
self.normalizer_layer = normalizer_layer
self.phi = MLP(
units=units,
activation=activation,
name="phi",
num_layers=phi_layers,
)
self.splitter = Split_Streams()
self.y_hiddens = [
MLP(
units=units,
activation=activation,
name=f"y_{k}",
num_layers=y_layers,
)
for k in range(n_treatments)
]
# add linear function to cover the normalized output
self.y_outputs = [
tf.keras.layers.Dense(
output_dim,
activation="sigmoid",
bias_initializer=output_bias,
name=f"top_{k}",
)
for k in range(n_treatments)
]
self.n_treatments = n_treatments
self.output_ = Gather_Streams()
def call(self, x):
cofeatures_input, treatment_input = x
treatment_cat = tf.cast(treatment_input, tf.int32)
if self.normalizer_layer:
cofeatures_input = self.normalizer_layer(cofeatures_input)
x_flux = self.phi(cofeatures_input)
streams = [
self.splitter([x_flux, treatment_cat, tf.cast(indice_treatment, tf.int32)])
for indice_treatment in range(len(self.y_hiddens))
]
# xstream is a list of tuple, containing the gathered and indice position, let's unpack them
x_streams, indice_streams = zip(*streams)
# tf.print(indice_streams, output_stream=sys.stderr)
x_streams = [
y_hidden(x_stream) for y_hidden, x_stream in zip(self.y_hiddens, x_streams)
]
x_streams = [
y_output(x_stream) for y_output, x_stream in zip(self.y_outputs, x_streams)
]
return self.output_([x_streams, indice_streams])
import tensorflow as tf
class Split_Streams(tf.keras.layers.Layer):
def __init__(self):
super(Split_Streams, self).__init__()
def call(self, inputs):
x, y, z = inputs
indice_position = tf.reshape(
tf.cast(tf.where(tf.equal(tf.reshape(y, (-1,)), z)), tf.int32),
(-1,),
)
return tf.gather(x, indice_position), indice_position
pd.get_dummies相当于onehot编码,常用与把离散的类别信息转化为onehot编码形式。
1.2Dragonet
多个treatment分别建模?
import tensorflow as tf
import keras.backend as K
from keras.engine.topology import Layer
from keras.metrics import binary_accuracy
from keras.layers import Input, Dense, Concatenate, BatchNormalization, Dropout
from keras.models import Model
from keras import regularizers
def binary_classification_loss(concat_true, concat_pred):
t_true = concat_true[:, 1]
t_pred = concat_pred[:, 2]
t_pred = (t_pred + 0.001) / 1.002
losst = tf.reduce_sum(K.binary_crossentropy(t_true, t_pred))
return losst
def regression_loss(concat_true, concat_pred):
y_true = concat_true[:, 0]
t_true = concat_true[:, 1]
y0_pred = concat_pred[:, 0]
y1_pred = concat_pred[:, 1]
loss0 = tf.reduce_sum((1. - t_true) * tf.square(y_true - y0_pred))
loss1 = tf.reduce_sum(t_true * tf.square(y_true - y1_pred))
return loss0 + loss1
def ned_loss(concat_true, concat_pred):
t_true = concat_true[:, 1]
t_pred = concat_pred[:, 1]
return tf.reduce_sum(K.binary_crossentropy(t_true, t_pred))
def dead_loss(concat_true, concat_pred):
return regression_loss(concat_true, concat_pred)
def dragonnet_loss_binarycross(concat_true, concat_pred):
return regression_loss(concat_true, concat_pred) + binary_classification_loss(concat_true, concat_pred)
def treatment_accuracy(concat_true, concat_pred):
t_true = concat_true[:, 1]
t_pred = concat_pred[:, 2]
return binary_accuracy(t_true, t_pred)
def track_epsilon(concat_true, concat_pred):
epsilons = concat_pred[:, 3]
return tf.abs(tf.reduce_mean(epsilons))
class EpsilonLayer(Layer):
def __init__(self):
super(EpsilonLayer, self).__init__()
def build(self, input_shape):
# Create a trainable weight variable for this layer.
self.epsilon = self.add_weight(name='epsilon',
shape=[1, 1],
initializer='RandomNormal',
# initializer='ones',
trainable=True)
super(EpsilonLayer, self).build(input_shape) # Be sure to call this at the end
def call(self, inputs, **kwargs):
# import ipdb; ipdb.set_trace()
return self.epsilon * tf.ones_like(inputs)[:, 0:1]
def make_tarreg_loss(ratio=1., dragonnet_loss=dragonnet_loss_binarycross):
def tarreg_ATE_unbounded_domain_loss(concat_true, concat_pred):
vanilla_loss = dragonnet_loss(concat_true, concat_pred)
y_true = concat_true[:, 0]
t_true = concat_true[:, 1]
y0_pred = concat_pred[:, 0]
y1_pred = concat_pred[:, 1]
t_pred = concat_pred[:, 2]
epsilons = concat_pred[:, 3]
t_pred = (t_pred + 0.01) / 1.02
# t_pred = tf.clip_by_value(t_pred,0.01, 0.99,name='t_pred')
y_pred = t_true * y1_pred + (1 - t_true) * y0_pred
h = t_true / t_pred - (1 - t_true) / (1 - t_pred)
y_pert = y_pred + epsilons * h
targeted_regularization = tf.reduce_sum(tf.square(y_true - y_pert))
# final
loss = vanilla_loss + ratio * targeted_regularization
return loss
return tarreg_ATE_unbounded_domain_loss
def make_dragonnet(input_dim, reg_l2):
"""
Neural net predictive model. The dragon has three heads.
:param input_dim:
:param reg:
:return:
"""
t_l1 = 0.
t_l2 = reg_l2
inputs = Input(shape=(input_dim,), name='input')
# representation
x = Dense(units=200, activation='elu', kernel_initializer='RandomNormal')(inputs)
x = Dense(units=200, activation='elu', kernel_initializer='RandomNormal')(x)
x = Dense(units=200, activation='elu', kernel_initializer='RandomNormal')(x)
t_predictions = Dense(units=1, activation='sigmoid')(x)
# HYPOTHESIS
y0_hidden = Dense(units=100, activation='elu', kernel_regularizer=regularizers.l2(reg_l2))(x)
y1_hidden = Dense(units=100, activation='elu', kernel_regularizer=regularizers.l2(reg_l2))(x)
# second layer
y0_hidden = Dense(units=100, activation='elu', kernel_regularizer=regularizers.l2(reg_l2))(y0_hidden)
y1_hidden = Dense(units=100, activation='elu', kernel_regularizer=regularizers.l2(reg_l2))(y1_hidden)
# third
y0_predictions = Dense(units=1, activation=None, kernel_regularizer=regularizers.l2(reg_l2), name='y0_predictions')(
y0_hidden)
y1_predictions = Dense(units=1, activation=None, kernel_regularizer=regularizers.l2(reg_l2), name='y1_predictions')(
y1_hidden)
dl = EpsilonLayer()
epsilons = dl(t_predictions, name='epsilon')
# logging.info(epsilons)
concat_pred = Concatenate(1)([y0_predictions, y1_predictions, t_predictions, epsilons])
model = Model(inputs=inputs, outputs=concat_pred)
return model
def make_tarnet(input_dim, reg_l2):
"""
Neural net predictive model. The dragon has three heads.
:param input_dim:
:param reg:
:return:
"""
inputs = Input(shape=(input_dim,), name='input')
# representation
x = Dense(units=200, activation='elu', kernel_initializer='RandomNormal')(inputs)
x = Dense(units=200, activation='elu', kernel_initializer='RandomNormal')(x)
x = Dense(units=200, activation='elu', kernel_initializer='RandomNormal')(x)
t_predictions = Dense(units=1, activation='sigmoid')(inputs)
# HYPOTHESIS
y0_hidden = Dense(units=100, activation='elu', kernel_regularizer=regularizers.l2(reg_l2))(x)
y1_hidden = Dense(units=100, activation='elu', kernel_regularizer=regularizers.l2(reg_l2))(x)
# second layer
y0_hidden = Dense(units=100, activation='elu', kernel_regularizer=regularizers.l2(reg_l2))(y0_hidden)
y1_hidden = Dense(units=100, activation='elu', kernel_regularizer=regularizers.l2(reg_l2))(y1_hidden)
# third
y0_predictions = Dense(units=1, activation=None, kernel_regularizer=regularizers.l2(reg_l2), name='y0_predictions')(
y0_hidden)
y1_predictions = Dense(units=1, activation=None, kernel_regularizer=regularizers.l2(reg_l2), name='y1_predictions')(
y1_hidden)
dl = EpsilonLayer()
epsilons = dl(t_predictions, name='epsilon')
# logging.info(epsilons)
concat_pred = Concatenate(1)([y0_predictions, y1_predictions, t_predictions, epsilons])
model = Model(inputs=inputs, outputs=concat_pred)
return model
def make_ned(input_dim, reg_l2=0.01):
"""
Neural net predictive model. The dragon has three heads.
:param input_dim:
:param reg:
:return:
"""
inputs = Input(shape=(input_dim,), name='input')
# representation
x = Dense(units=200, activation='elu', kernel_initializer='RandomNormal', name='ned_hidden1')(inputs)
x = Dense(units=200, activation='elu', kernel_initializer='RandomNormal', name='ned_hidden2')(x)
x = Dense(units=200, activation='elu', kernel_initializer='RandomNormal', name='ned_hidden3')(x)
t_predictions = Dense(units=1, activation='sigmoid', name='ned_t_activation')(x)
y_predictions = Dense(units=1, activation=None, name='ned_y_prediction')(x)
concat_pred = Concatenate(1)([y_predictions, t_predictions])
model = Model(inputs=inputs, outputs=concat_pred)
return model
def post_cut(nednet, input_dim, reg_l2=0.01):
for layer in nednet.layers:
layer.trainable = False
nednet.layers.pop()
nednet.layers.pop()
nednet.layers.pop()
frozen = nednet
x = frozen.layers[-1].output
frozen.layers[-1].outbound_nodes = []
input = frozen.input
y0_hidden = Dense(units=100, activation='elu', kernel_regularizer=regularizers.l2(reg_l2), name='post_cut_y0_1')(x)
y1_hidden = Dense(units=100, activation='elu', kernel_regularizer=regularizers.l2(reg_l2), name='post_cut_y1_1')(x)
# second layer
y0_hidden = Dense(units=100, activation='elu', kernel_regularizer=regularizers.l2(reg_l2), name='post_cut_y0_2')(
y0_hidden)
y1_hidden = Dense(units=100, activation='elu', kernel_regularizer=regularizers.l2(reg_l2), name='post_cut_y1_2')(
y1_hidden)
# third
y0_predictions = Dense(units=1, activation=None, kernel_regularizer=regularizers.l2(reg_l2), name='y0_predictions')(
y0_hidden)
y1_predictions = Dense(units=1, activation=None, kernel_regularizer=regularizers.l2(reg_l2), name='y1_predictions')(
y1_hidden)
concat_pred = Concatenate(1)([y0_predictions, y1_predictions])
model = Model(inputs=input, outputs=concat_pred)
return model
1.3DRNet
Learning Counterfactual Representations for Estimating Individual Dose-Response Curves
1.4VCNet
处理连续的treatment
本文基于varying coefficient model,让treatment对应的branch成为treatment的函数,而不需要单独设计branch,达到真正的连续性。除此之外,本文也沿用了《Adapting Neural Networks for the Estimation of Treatment Effects》一文中的思路,训练一个分类器来抽取协变量中与T最相关的部分变量。 ?
参考:
- [Dragonet] Adapting Neural Network for the Estimation of Treatment Effects - 知乎
- BradyNeal因果推断课程笔记8-经典模型框架DML、S-learner、T-learner、X-learner、TARNet
- 《因果推理导论》课程(2020) by Brady Neal_哔哩哔哩_bilibili
-
Shi, Claudia, David Blei, and Victor Veitch. "Adapting neural networks for the estimation of treatment effects." Advances in neural information processing systems 32 (2019). -
Shalit, Uri, Fredrik D. Johansson, and David Sontag. "Estimating individual treatment effect: generalization bounds and algorithms." International Conference on Machine Learning. PMLR, 2017. -
详解“因果效应估计”_AI_盲的博客-CSDN博客_连续型干预因果效应估计 -
Search :: Anaconda.org -
dragonnet/models.py at master · claudiashi57/dragonnet · GitHub -
PackagesNotFoundError: The following packages are not available from current channels的解决办法_正在学习的黄老师的博客-CSDN博客_packagesnotfounderror -
DRNet:Learning Counterfactual Representations for Estimating Individual Dose-Response Curves
二、待补充
- 论文精读:DRNet、VCNet
- 相关代码细节
- 工业应用实例
|